num_features=args.features
时间: 2023-11-03 22:06:23 浏览: 82
I'm sorry, as an AI language model, I do not have access to the context or the arguments being referred to. Please provide me with more information so that I can assist you better.
相关问题
这段代码哪里错了 class my_BiGRU(torch.nn.Module): def __init__(self, input_size, hidden_size, num_layers, output_size, gru_dropout): super(my_BiGRU, self).__init__() self.input_size = input_size self.hidden_size = hidden_size self.num_layers = num_layers self.output_size = output_size self.gru_dropout = gru_dropout self.gru = torch.nn.GRU(input_size, hidden_size, num_layers=num_layers, batch_first=True, bidirectional=True) self.fc = torch.nn.Linear(hidden_size * 2, output_size) def _setup_BiGRU_layers(self): self.BiGRU_layers = my_BiGRU(input_size=self.args.capsule_out_dim, output_size=self.args.apsule_out_features, hidden_size=self.args.BiGRU_hiddensize, num_layers=self.args.BiGRU_layers, gru_dropout=self.args.BiGRU_dropout, )
这段代码存在两个问题:
1.函数 `_setup_BiGRU_layers` 中的代码应该是在类的构造函数 `__init__` 中进行初始化,而不是在另外一个函数中进行初始化。
2.在调用 `my_BiGRU` 类时,参数名称有误,应该将 `apsule_out_features` 改为 `capsule_out_features`。
改正后的代码如下所示:
```
class my_BiGRU(torch.nn.Module):
def __init__(self, input_size, hidden_size, num_layers, output_size, gru_dropout, capsule_out_features):
super(my_BiGRU, self).__init__()
self.input_size = input_size
self.hidden_size = hidden_size
self.num_layers = num_layers
self.output_size = output_size
self.gru_dropout = gru_dropout
self.gru = torch.nn.GRU(input_size, hidden_size, num_layers=num_layers, batch_first=True, bidirectional=True)
self.fc = torch.nn.Linear(hidden_size * 2, output_size)
self.BiGRU_layers = my_BiGRU(input_size=self.input_size,
output_size=capsule_out_features,
hidden_size=self.hidden_size,
num_layers=self.num_layers,
gru_dropout=self.gru_dropout,
)
```
注意:这里假设 `capsule_out_dim` 和 `args` 都已经在代码中被定义好了。
model = ARMA4NC(in_dim=n_features, hid_dim=args.hid_dim, out_dim=n_classes, num_stacks=args.num_stacks, num_layers=args.num_layers, activation=nn.ReLU(), dropout=args.dropout).to(device)。调用这个函数
这个函数定义了一个 ARMA4NC 模型,它有以下参数:
- `in_dim`:输入特征的维度。
- `hid_dim`:隐藏层的维度。
- `out_dim`:输出的类别数。
- `num_stacks`:堆叠的 ARMA 层的数量。
- `num_layers`:每个 ARMA 层中的 LSTM 层数量。
- `activation`:激活函数。
- `dropout`:Dropout 的概率。
这个函数返回一个 ARMA4NC 模型,并将其移动到指定的设备上(在这里是 `device`)。
如果你想使用这个模型,你可以这样调用它:
```python
model = ARMA4NC(in_dim=10, hid_dim=20, out_dim=2, num_stacks=3, num_layers=2, activation=nn.ReLU(), dropout=0.2).to(device)
```
这样就创建了一个输入维度为 10,输出类别数为 2,有 3 个堆叠的 ARMA 层,每个 ARMA 层有 2 层 LSTM,使用 ReLU 激活函数和 0.2 的 Dropout 概率的 ARMA4NC 模型,并将其移动到指定的设备上。
相关推荐
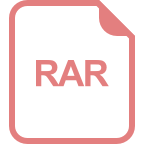
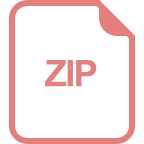
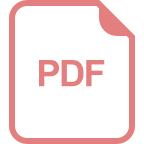












