male_counts = df.groupby('籍贯')['性别']['男'].value_counts().unstack()这段代码如何改进,不适用unstack
时间: 2023-05-27 11:03:35 浏览: 57
You can use pivot_table instead of groupby and unstack to achieve the same result.
Here's how you can do it:
```
male_counts = pd.pivot_table(df[df['性别']=='男'], index='籍贯', values='性别', aggfunc='count')
```
In this code, you first filter the DataFrame to only include rows where '性别' is '男' using `df[df['性别']=='男']`. Then, you use `pd.pivot_table` to create a new DataFrame where '籍贯' is the index, the values are the count of '性别', and the aggregation function is 'count'.
This should give you the same result as using `groupby` and `unstack`.
相关问题
male_counts = df.groupby('籍贯')['性别']['男'].value_counts().unstack()这段代码如何改进
### 回答1:
这段代码可以改进为:
```
male_counts = df[df['性别'] == '男'].groupby('籍贯')['性别'].value_counts().unstack()
```
这个代码将首先对数据框进行筛选,然后只保留性别为男的观测值;接着按照籍贯和性别统计数量,最后使用unstack()函数将性别作为列名转换成列。这个代码与原代码的结果应该相同,但更具可读性和可维护性。
### 回答2:
要对这段代码进行改进的话,可以考虑以下几点:
1. 确保数据的准确性:在进行分组和计数操作之前,需要先对数据进行清洗和预处理,排除缺失值或异常值,以确保结果的准确性。
2. 变量命名规范化:代码中的“male_counts”可能会给读者造成困惑,建议将变量名更改为更具描述性和可理解性的名称。
3. 代码可读性的提升:可以对代码进行合适的缩进和注释,以提高代码的可读性和可维护性。
4. 代码重构的优化:可以对代码进行优化,减少不必要的计算,提高代码的执行效率。例如,可以直接使用.value_counts()函数来计算男性人数,而不需要再进行.unstack()操作。
改进后的代码示例:
```python
# 数据清洗和预处理
df_cleaned = df.dropna(subset=['籍贯', '性别'])
df_cleaned['性别'] = df_cleaned['性别'].astype(str) # 确保性别字段数据类型为字符串
# 分组计数
gender_counts = df_cleaned.groupby('籍贯')['性别'].value_counts()
# 输出男性人数
male_counts = gender_counts.loc[:, '男']
```
改进后的代码通过清洗和预处理数据,使得计算结果更加准确可靠。同时,合理命名变量、添加注释和优化代码逻辑,提高了代码的可读性和效率。
### 回答3:
这段代码可以通过以下方式进行改进:
1. 使用.pipe()方法进行函数链式调用,将操作合并在一起,方便读写和维护。
```python
male_counts = df.groupby('籍贯').pipe(lambda x: x[x['性别'] == '男']).value_counts().unstack()
```
2. 使用索引筛选来代替条件判断,以提高代码的可读性和执行效率。
```python
male_counts = df[df['性别'] == '男'].groupby('籍贯')['性别'].value_counts().unstack()
```
3. 提前进行性别筛选,只对需要的性别数据进行分组和统计,以减少不必要的计算。
```python
male_df = df[df['性别'] == '男']
male_counts = male_df.groupby('籍贯')['性别'].value_counts().unstack()
```
4. 使用reset_index()方法将索引重置,使结果返回一个DataFrame对象而不是Series对象。
```python
male_counts = male_counts.reset_index()
```
综上所述,以上是对给定代码的改进方式。具体改进方法的选择可以根据实际需求和数据集的特点进行调整。
male_counts = df.groupby('籍贯')['性别']['男'].value_counts().unstack()这段代码如何改进成一维数据
One possible way to transform the code to a one-dimensional data format is to use the pandas groupby() function to group the data by '籍贯' and '性别', and then use the value_counts() function to count the occurrences of each value in '性别'. Finally, use the reset_index() function to convert the resulting dataframe to a one-dimensional series.
Here is the modified code:
```
male_counts = df.groupby(['籍贯', '性别'])['性别'].count()
male_counts = male_counts[male_counts.index.get_level_values('性别') == '男'].reset_index(drop=True)
```
This will create a one-dimensional series called 'male_counts' with the number of males for each '籍贯'.
相关推荐
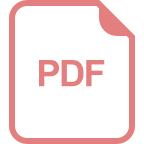
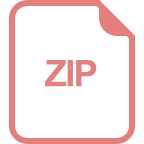
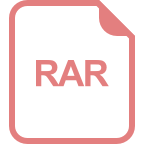












