解释代码:softmax: bool = False,
时间: 2024-06-05 13:07:59 浏览: 16
这是一个函数的参数,被赋予了默认值 False。该参数用于控制是否在计算输出时使用 softmax 函数。
softmax 函数是一种常用的激活函数,常用于多分类问题的输出层。它将一个向量中的每个元素转化为一个介于 0 和 1 之间的值,并且所有元素的和为 1。这样可以将向量看作是一个概率分布,每个元素表示该类别的概率。
如果参数 softmax 被设置为 True,则会在计算输出时使用 softmax 函数。否则,将直接输出模型的线性输出,不进行任何激活函数的处理。
相关问题
这段代码有什么错误def forward(self,x): num_nodes = x.size(1) # sub_graph size batch_size = x.size(0) W = torch.cat([self.W] * batch_size, dim=0) representation = torch.matmul(x, W) r_sum = torch.sum(representation, dim=-1, keepdim=False) b = torch.zeros([batch_size, num_nodes]) b = Variable(b) one = torch.ones_like(r_sum) zero = torch.zeros_like(r_sum) label = torch.clone(r_sum) label = torch.where(label == 0, one, zero) b.data.masked_fill_(label.bool(), -float('inf')) num_iterations = 3 for i in range(num_iterations): c = torch.nn.functional.softmax(b, dim=-1) weight_coeff = c.unsqueeze(dim=1) representation_global = torch.matmul(weight_coeff, representation) representation_global_all = torch.cat([representation_global] * num_nodes, dim=1) representation_similarity = torch.nn.functional.cosine_similarity(representation, representation_global_all, dim=-1) representation_similarity.data.masked_fill_(label.bool(), -float('inf')) b = representation_similarity return representation_global.squeeze(dim=1)
这段代码中存在一个错误。在for循环中,代码更新了变量b,但是在更新后没有再次进行softmax操作,导致后续的计算结果不正确。因此,需要在for循环中,在更新b后再次进行softmax操作,如下所示:
```
for i in range(num_iterations):
c = torch.nn.functional.softmax(b, dim=-1)
weight_coeff = c.unsqueeze(dim=1)
representation_global = torch.matmul(weight_coeff, representation)
representation_global_all = torch.cat([representation_global] * num_nodes, dim=1)
representation_similarity = torch.nn.functional.cosine_similarity(representation, representation_global_all, dim=-1)
representation_similarity.data.masked_fill_(label.bool(), -float('inf'))
b = torch.nn.functional.softmax(representation_similarity, dim=-1) # 进行softmax操作
```
这样修改后,该段代码的功能应该能够正常运行。
informer模型代码
以下是一个简单的informer模型的PyTorch代码实现:
```python
import torch
import torch.nn as nn
import torch.nn.functional as F
class Informer(nn.Module):
def __init__(self, enc_in, dec_in, out_seq_len, n_heads, e_layers, d_layers, d_ff, dropout, activation='relu'):
super(Informer, self).__init__()
self.encoder = Encoder(enc_in, n_heads, e_layers, d_ff, dropout, activation)
self.decoder = Decoder(dec_in, out_seq_len, n_heads, d_layers, d_ff, dropout, activation)
self.out = nn.Linear(dec_in, out_seq_len)
def forward(self, x):
enc_out, attn = self.encoder(x)
dec_out = self.decoder(enc_out, attn)
out = self.out(dec_out)
return out
class Encoder(nn.Module):
def __init__(self, input_dim, n_heads, n_layers, d_ff, dropout, activation):
super(Encoder, self).__init__()
self.layers = nn.ModuleList()
for i in range(n_layers):
self.layers.append(EncoderLayer(input_dim, n_heads, d_ff, dropout, activation))
def forward(self, x):
attn_weights = []
for layer in self.layers:
x, attn_weight = layer(x)
attn_weights.append(attn_weight)
return x, attn_weights
class EncoderLayer(nn.Module):
def __init__(self, input_dim, n_heads, d_ff, dropout, activation):
super(EncoderLayer, self).__init__()
self.self_attn = MultiHeadAttention(n_heads, input_dim, input_dim, dropout)
self.feed_forward = FeedForward(input_dim, d_ff, activation, dropout)
self.norm1 = nn.LayerNorm(input_dim)
self.norm2 = nn.LayerNorm(input_dim)
self.dropout1 = nn.Dropout(dropout)
self.dropout2 = nn.Dropout(dropout)
def forward(self, x):
# self-attention
residual = x
x, attn_weight = self.self_attn(x, x, x)
x = self.norm1(residual + self.dropout1(x))
# feed forward
residual = x
x = self.feed_forward(x)
x = self.norm2(residual + self.dropout2(x))
return x, attn_weight
class Decoder(nn.Module):
def __init__(self, input_dim, out_seq_len, n_heads, n_layers, d_ff, dropout, activation):
super(Decoder, self).__init__()
self.layers = nn.ModuleList()
for i in range(n_layers):
self.layers.append(DecoderLayer(input_dim, n_heads, d_ff, dropout, activation))
self.out_seq_len = out_seq_len
self.linear = nn.Linear(input_dim, out_seq_len)
def forward(self, enc_out, attn_weights):
# mask future positions
mask = torch.triu(torch.ones(self.out_seq_len, self.out_seq_len), diagonal=1)
mask = mask.unsqueeze(0).bool().to(enc_out.device)
# self-attention
x = torch.zeros(enc_out.shape[0], self.out_seq_len, enc_out.shape[-1]).to(enc_out.device)
for i in range(self.out_seq_len):
residual = x[:, i, :]
x[:, i, :], attn_weight = self.layers[i](x[:, :i+1, :], enc_out, mask, attn_weights)
x[:, i, :] = residual + x[:, i, :]
# linear
out = self.linear(x)
return out
class DecoderLayer(nn.Module):
def __init__(self, input_dim, n_heads, d_ff, dropout, activation):
super(DecoderLayer, self).__init__()
self.self_attn = MultiHeadAttention(n_heads, input_dim, input_dim, dropout)
self.enc_attn = MultiHeadAttention(n_heads, input_dim, input_dim, dropout)
self.feed_forward = FeedForward(input_dim, d_ff, activation, dropout)
self.norm1 = nn.LayerNorm(input_dim)
self.norm2 = nn.LayerNorm(input_dim)
self.norm3 = nn.LayerNorm(input_dim)
self.dropout1 = nn.Dropout(dropout)
self.dropout2 = nn.Dropout(dropout)
self.dropout3 = nn.Dropout(dropout)
def forward(self, x, enc_out, mask, attn_weights):
# self-attention
residual = x[:, -1, :]
x[:, -1, :], attn_weight1 = self.self_attn(x[:, -1:, :], x[:, -1:, :], x[:, -1:, :], mask)
x[:, -1, :] = residual + self.dropout1(x[:, -1, :])
# encoder-decoder attention
residual = x[:, -1, :]
x[:, -1, :], attn_weight2 = self.enc_attn(x[:, -1:, :], enc_out, enc_out)
x[:, -1, :] = residual + self.dropout2(x[:, -1, :])
# feed forward
residual = x[:, -1, :]
x[:, -1, :] = self.feed_forward(x[:, -1, :])
x[:, -1, :] = residual + self.dropout3(x[:, -1, :])
attn_weights.append(torch.cat([attn_weight1, attn_weight2], dim=1))
return x, attn_weights
class MultiHeadAttention(nn.Module):
def __init__(self, n_heads, q_dim, k_dim, dropout):
super(MultiHeadAttention, self).__init__()
self.n_heads = n_heads
self.q_dim = q_dim
self.k_dim = k_dim
self.query = nn.Linear(q_dim, q_dim * n_heads)
self.key = nn.Linear(k_dim, k_dim * n_heads)
self.value = nn.Linear(k_dim, k_dim * n_heads)
self.out = nn.Linear(k_dim * n_heads, q_dim)
self.dropout = nn.Dropout(dropout)
def forward(self, query, key, value, mask=None):
batch_size = query.shape[0]
# linear
query = self.query(query).view(batch_size, -1, self.n_heads, self.q_dim // self.n_heads).transpose(1, 2)
key = self.key(key).view(batch_size, -1, self.n_heads, self.k_dim // self.n_heads).transpose(1, 2)
value = self.value(value).view(batch_size, -1, self.n_heads, self.k_dim // self.n_heads).transpose(1, 2)
# dot product attention
attn_weight = torch.matmul(query, key.transpose(-2, -1)) / torch.sqrt(torch.tensor(self.k_dim // self.n_heads).float().to(query.device))
if mask is not None:
attn_weight = attn_weight.masked_fill(mask == False, -1e9)
attn_weight = F.softmax(attn_weight, dim=-1)
attn_weight = self.dropout(attn_weight)
# linear
output = torch.matmul(attn_weight, value).transpose(1, 2).contiguous().view(batch_size, -1, self.q_dim)
output = self.out(output)
return output, attn_weight
class FeedForward(nn.Module):
def __init__(self, input_dim, hidden_dim, activation, dropout):
super(FeedForward, self).__init__()
self.linear1 = nn.Linear(input_dim, hidden_dim)
self.linear2 = nn.Linear(hidden_dim, input_dim)
self.activation = getattr(F, activation)
self.dropout = nn.Dropout(dropout)
def forward(self, x):
x = self.linear1(x)
x = self.activation(x)
x = self.dropout(x)
x = self.linear2(x)
return x
```
这里实现了一个简单的Informer模型,包括Encoder、Decoder和MultiHeadAttention等模块。你可以根据具体的任务和数据来调整模型的参数和结构,以获得更好的性能。
相关推荐
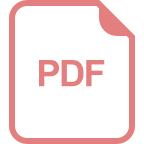
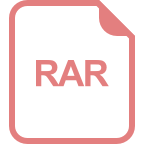
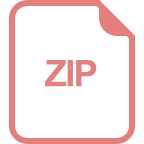







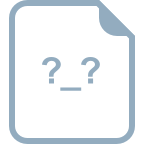
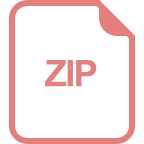
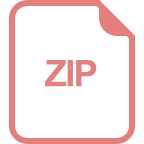
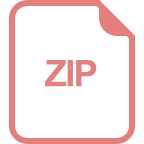
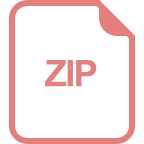
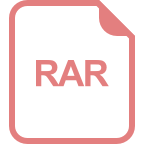