loss_function='MultiClass'
时间: 2024-01-27 15:03:22 浏览: 164
"MultiClass" is not a commonly used loss function name in machine learning. Can you provide more context about what you are trying to accomplish with this loss function?
相关问题
loss_function='MultiClass'什么意思
`loss_function='MultiClass'` 表示多分类任务的损失函数。在这种情况下,模型需要将输入分为多个可能的类别中的一个。通常使用的损失函数是交叉熵损失函数,它在神经网络中被广泛使用。它可以测量模型预测的概率分布与真实概率分布之间的差异,并将其最小化,以便模型能够更好地预测类别。在多分类任务中,我们通常使用softmax函数将输出转化为概率分布。
if loss == "SoftCE_dice": DiceLoss_fn = DiceLoss(mode='multiclass') SoftCrossEntropy_fn = SoftCrossEntropyLoss(smooth_factor=0.1) loss_fn = lo.JointLoss().to(DEVICE) else: LovaszLoss_fn = LovaszLoss(mode='multiclass') SoftCrossEntropy_fn = SoftCrossEntropyLoss(smooth_factor=0.1) loss_fn = lo.JointLoss().to(DEVICE)
这段代码是一个条件语句,根据`loss`变量的值选择不同的损失函数。
如果`loss`等于`"SoftCE_dice"`,则选择Soft Cross Entropy Dice Loss。这个损失函数由两个部分组成:Soft Cross Entropy Loss和Dice Loss。其中,Soft Cross Entropy Loss是一种常规的交叉熵损失函数,用于多分类问题。Dice Loss是一种基于Dice系数的损失函数,用于处理分割问题。两个损失函数被结合在一起,以帮助提高模型的性能。
如果`loss`不等于`"SoftCE_dice"`,则选择Lovasz Softmax Loss。这个损失函数是一种对称交叉熵损失函数,用于多标签分类问题。它基于Lovasz扩展,能够更好地处理不完整分割问题。和Soft Cross Entropy Loss一样,它也被结合在一起,以帮助提高模型的性能。
无论选择哪种损失函数,都会使用Soft Cross Entropy Loss作为其中一个组成部分,并使用Joint Loss将多个损失函数结合在一起。最终的损失函数被赋值给`loss_fn`变量,并移动到计算设备上(通常是GPU)。
希望能够解答您的问题!
阅读全文
相关推荐
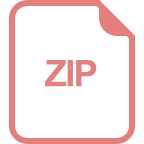
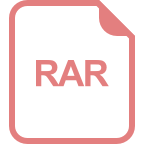
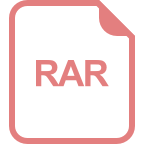












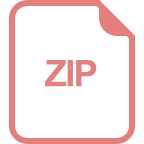