def make_data(sentences): """把单词序列转换为数字序列""" enc_inputs, dec_inputs, dec_outputs = [], [], [] for i in range(len(sentences)): enc_input = [[src_vocab[n] for n in sentences[i][0].split()]] dec_input = [[tgt_vocab[n] for n in sentences[i][1].split()]] dec_output = [[tgt_vocab[n] for n in sentences[i][2].split()]] # [[1, 2, 3, 4, 5, 6, 7, 0], [1, 2, 8, 4, 9, 6, 7, 0], [1, 2, 3, 4, 10, 6, 7, 0]] enc_inputs.extend(enc_input) # [[9, 1, 2, 3, 4, 5, 11], [9, 1, 2, 6, 7, 5, 11], [9, 1, 2, 3, 8, 5, 11]] dec_inputs.extend(dec_input) # [[1, 2, 3, 4, 5, 11, 10], [1, 2, 6, 7, 5, 11, 10], [1, 2, 3, 8, 5, 11, 10]] dec_outputs.extend(dec_output) return torch.LongTensor(enc_inputs), torch.LongTensor(dec_inputs), torch.LongTensor(dec_outputs)
时间: 2023-11-30 20:50:02 浏览: 79
这是一个Python函数,用于将单词序列转换为数字序列。其中,输入参数sentences是一个列表,包含若干个元素,每个元素都是一个三元组,表示一组源语言句子、目标语言句子和目标语言句子的标签。该函数的输出是三个torch.LongTensor类型的变量,分别表示源语言句子、目标语言句子和目标语言句子标签的数字表示。
具体来说,该函数首先定义了三个空列表enc_inputs、dec_inputs和dec_outputs,用于存储数字序列。然后,对于sentences中的每个元素,分别将源语言句子、目标语言句子和目标语言句子标签转换为数字序列,并将其分别添加到enc_inputs、dec_inputs和dec_outputs中。最后,将enc_inputs、dec_inputs和dec_outputs分别转换为torch.LongTensor类型的变量,并作为函数的输出。这个函数是用于构建神经机器翻译模型的数据预处理的一部分。
相关问题
def beam_decode(decoder, decoder_hidden, encoder_outputs, voc, beam_size, max_length=MAX_LENGTH): terminal_sentences, prev_top_sentences, next_top_sentences = [], [], [] prev_top_sentences.append(Sentence(decoder_hidden)) for i in range(max_length): for sentence in prev_top_sentences: decoder_input = torch.LongTensor([[sentence.last_idx]]) decoder_input = decoder_input.to(device) decoder_hidden = sentence.decoder_hidden decoder_output, decoder_hidden, _ = decoder( decoder_input, decoder_hidden, encoder_outputs ) topv, topi = decoder_output.topk(beam_size) term, top = sentence.addTopk(topi, topv, decoder_hidden, beam_size, voc) terminal_sentences.extend(term) next_top_sentences.extend(top) next_top_sentences.sort(key=lambda s: s.avgScore(), reverse=True) prev_top_sentences = next_top_sentences[:beam_size] next_top_sentences = [] terminal_sentences += [sentence.toWordScore(voc) for sentence in prev_top_sentences] terminal_sentences.sort(key=lambda x: x[1], reverse=True) n = min(len(terminal_sentences), 15) return terminal_sentences[:n]
这段代码实现了一个 beam search 解码函数 `beam_decode`,用于在序列到序列模型中生成输出序列。下面是对该函数的解释:
- `beam_decode` 函数接受以下参数:
- `decoder`:解码器模型
- `decoder_hidden`:解码器的初始隐藏状态
- `encoder_outputs`:编码器的输出
- `voc`:词汇表对象,用于将索引转换为单词
- `beam_size`:束搜索的大小,即每个时间步保留的候选序列数
- `max_length`:生成序列的最大长度,默认为预定义的最大长度 `MAX_LENGTH`
- 首先,创建三个列表:`terminal_sentences` 用于存储终止的句子,`prev_top_sentences` 用于存储上一步的候选句子,`next_top_sentences` 用于存储下一步的候选句子。
- 然后,将初始隐藏状态封装在 `Sentence` 对象中,并将其添加到 `prev_top_sentences` 列表中。
- 接下来,使用一个循环来生成序列。在每个时间步中,对于每个候选句子,执行以下操作:
- 创建一个张量 `decoder_input`,将上一步的最后一个索引作为输入。
- 将 `decoder_input` 移动到适当的设备上。
- 使用解码器模型和编码器的输出计算解码器的输出、隐藏状态。
- 从解码器的输出中选择前 `beam_size` 个最高值作为候选词的索引。
- 将候选词、候选分数和隐藏状态添加到 `sentence` 对象中的 `topk` 列表中。
- 在生成完整的序列之后,将终止的句子和前 `beam_size` 个候选句子按照平均分数进行排序。
- 最后,返回前 `n` 个最高分数的句子作为最终生成的序列。
希望以上解释对您有帮助!如果您还有其他问题,请随时提问。
import fitz # PyMuPDF库 import os # 读取PDF文件的内容 def read_pdf(file_path): doc = fitz.open(file_path) content = "" for page in doc: content += page.getText("text") doc.close() return content # 去除字符串中的空格和空行 def remove_spaces(text): return "\n".join([line.strip() for line in text.split("\n") if line.strip()]) # 将字符串按中文句号分割成多个语句 def split_sentences(text): sentences = [] for sentence in text.split("。"): sentence = sentence.strip() if sentence: sentences.append(sentence + "。") return sentences # 读取指定目录下所有PDF文件的内容并合并成一个字符串 def read_all_pdfs(dir_path): all_content = "" for file_name in os.listdir(dir_path): if file_name.endswith(".pdf"): file_path = os.path.join(dir_path, file_name) content = read_pdf(file_path) content = remove_spaces(content) all_content += content return all_content # 将字符串按中文句号分割成多个语句并打印出来 def print_sentences(text): sentences = split_sentences(text) for sentence in sentences: print(sentence) # 测试 dir_path = r"D:\点宽学院" all_content = read_all_pdfs(dir_path) print_sentences(all_content)代码运行显示AttributeError: 'Page' object has no attribute 'getText'我该如何修改
这个错误可能是因为fitz的版本问题,可以尝试更新fitz库。同时,建议在读取PDF文件时,使用try-except语句来捕捉异常,以便更好地处理错误。
以下是更新fitz库和添加try-except语句的修改代码:
```python
import fitz # PyMuPDF库
import os # 读取PDF文件的内容
def read_pdf(file_path):
doc = fitz.open(file_path)
content = ""
for page in doc:
try:
content += page.getText("text")
except:
pass
doc.close()
return content
# 去除字符串中的空格和空行
def remove_spaces(text):
return "\n".join([line.strip() for line in text.split("\n") if line.strip()])
# 将字符串按中文句号分割成多个语句
def split_sentences(text):
sentences = []
for sentence in text.split("。"):
sentence = sentence.strip()
if sentence:
sentences.append(sentence + "。")
return sentences
# 读取指定目录下所有PDF文件的内容并合并成一个字符串
def read_all_pdfs(dir_path):
all_content = ""
for file_name in os.listdir(dir_path):
if file_name.endswith(".pdf"):
file_path = os.path.join(dir_path, file_name)
content = read_pdf(file_path)
content = remove_spaces(content)
all_content += content
return all_content
# 将字符串按中文句号分割成多个语句并打印出来
def print_sentences(text):
sentences = split_sentences(text)
for sentence in sentences:
print(sentence)
# 测试
dir_path = r"D:\点宽学院"
all_content = read_all_pdfs(dir_path)
print_sentences(all_content)
```
阅读全文
相关推荐
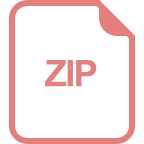
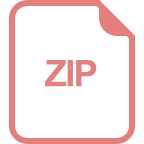
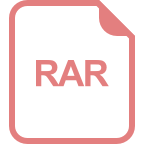
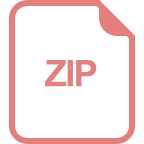










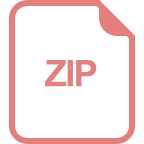
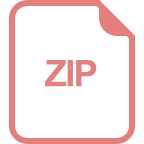