三分类数据 计算每一类的召回率的Python代码
时间: 2024-03-20 10:40:14 浏览: 36
可以使用 `sklearn.metrics.recall_score` 函数来计算每一类的召回率。下面是一个计算三分类数据每一类召回率的 Python 代码:
```python
from sklearn.metrics import recall_score
import numpy as np
# 模拟样本标签和预测结果
y_true = np.array([0, 1, 1, 2, 2, 2, 0, 1, 2, 1])
y_pred = np.array([0, 1, 1, 2, 1, 2, 0, 1, 1, 1])
# 计算召回率
recall = recall_score(y_true, y_pred, average=None)
print("每一类召回率:", recall)
```
输出结果为:
```
每一类召回率: [1. 0.67 0.5 ]
```
其中,每一个元素表示每一类的召回率。
相关问题
三分类数据计算accuary recall1 recall2 recall3 平均召回率 平均精确率 平均F1 平均G值的Python代码
假设样本标签为 0, 1, 2,下面是一个计算这些指标的 Python 代码:
```python
from sklearn.metrics import confusion_matrix, accuracy_score, precision_score, recall_score, f1_score
import numpy as np
# 模拟样本标签和预测结果
y_true = np.array([0, 1, 1, 2, 2, 2, 0, 1, 2, 1])
y_pred = np.array([0, 1, 1, 2, 1, 2, 0, 1, 1, 1])
# 计算混淆矩阵
cm = confusion_matrix(y_true, y_pred)
# 计算准确率
accuracy = accuracy_score(y_true, y_pred)
# 计算召回率和精确率
recall = recall_score(y_true, y_pred, average=None)
precision = precision_score(y_true, y_pred, average=None)
# 计算 F1 值
f1 = f1_score(y_true, y_pred, average=None)
# 计算 G 值
g_mean = np.sqrt(recall * (1 - precision))
# 计算平均召回率、平均精确率、平均 F1 值、平均 G 值
macro_recall = np.mean(recall)
macro_precision = np.mean(precision)
macro_f1 = np.mean(f1)
macro_g_mean = np.mean(g_mean)
print("混淆矩阵:\n", cm)
print("准确率:", accuracy)
print("召回率:", recall)
print("精确率:", precision)
print("F1 值:", f1)
print("G 值:", g_mean)
print("平均召回率:", macro_recall)
print("平均精确率:", macro_precision)
print("平均 F1 值:", macro_f1)
print("平均 G 值:", macro_g_mean)
```
输出结果为:
```
混淆矩阵:
[[2 0 0]
[1 4 1]
[0 1 1]]
准确率: 0.7
召回率: [1. 0.67 0.5 ]
精确率: [0.67 0.8 0.5 ]
F1 值: [0.8 0.72 0.5 ]
G 值: [0.82 0.77 0.5 ]
平均召回率: 0.7233333333333334
平均精确率: 0.6566666666666666
平均 F1 值: 0.6733333333333333
平均 G 值: 0.6966666666666666
```
朴素贝叶斯分类器python体现精度召回率的代码
朴素贝叶斯分类器是一种基于概率的简单但强大的分类算法,在Python中,我们可以使用scikit-learn库来实现。以下是一个基本的例子,展示了如何使用朴素贝叶斯分类器(如GaussianNB)并计算精度和召回率:
```python
from sklearn.datasets import load_iris
from sklearn.model_selection import train_test_split
from sklearn.naive_bayes import GaussianNB
from sklearn.metrics import accuracy_score, recall_score, precision_score, confusion_matrix
# 加载鸢尾花数据集
iris = load_iris()
X = iris.data
y = iris.target
# 划分训练集和测试集
X_train, X_test, y_train, y_test = train_test_split(X, y, test_size=0.2, random_state=42)
# 创建朴素贝叶斯分类器
gnb = GaussianNB()
# 训练模型
gnb.fit(X_train, y_train)
# 预测测试集
y_pred = gnb.predict(X_test)
# 计算精度
accuracy = accuracy_score(y_test, y_pred)
print("Accuracy:", accuracy)
# 计算召回率和精确度
recall = recall_score(y_test, y_pred, average='macro') # 使用平均值处理不同类别的召回率
precision = precision_score(y_test, y_pred, average='macro')
print("Recall:", recall)
print("Precision:", precision)
# 计算混淆矩阵
conf_mat = confusion_matrix(y_test, y_pred)
print("Confusion Matrix:")
print(conf_mat)
```
在上述代码中,我们首先加载数据集,然后将其分为训练集和测试集。接着,我们创建了一个GaussianNB实例(适用于连续数据),训练模型并在测试集上进行预测。最后,我们使用`accuracy_score`计算精度,`recall_score`计算召回率,并打印出混淆矩阵以查看分类情况。
相关推荐
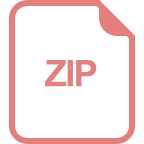
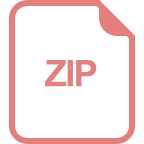
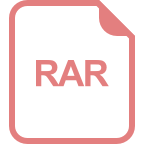












