from utils.common_config import get_criterion, get_model, get_train_dataset,\ get_val_dataset, get_train_dataloader,\ get_val_dataloader, get_train_transformations,\ get_val_transformations, get_optimizer,\ adjust_learning_rate
时间: 2024-04-28 15:26:13 浏览: 98
这行代码导入了一个Python模块或函数集合`utils.common_config`,并分别从中导入了多个函数,包括:
- `get_criterion`:用于获取损失函数
- `get_model`:用于获取模型
- `get_train_dataset`:用于获取训练集数据
- `get_val_dataset`:用于获取验证集数据
- `get_train_dataloader`:用于获取训练集数据加载器
- `get_val_dataloader`:用于获取验证集数据加载器
- `get_train_transformations`:用于获取训练集数据增强方法
- `get_val_transformations`:用于获取验证集数据增强方法
- `get_optimizer`:用于获取优化器
- `adjust_learning_rate`:用于调整学习率
这些函数很可能是用于搭建深度学习模型训练流程的工具函数,通过调用这些函数,可以方便地获取模型、数据、优化器等实现模型训练所需的组件。这样可以大大简化训练代码的编写,并提高代码的可读性和可维护性。
相关问题
import argparse import os import torch import numpy as np from utils.config import create_config from utils.common_config import get_criterion, get_model, get_train_dataset,\ get_val_dataset, get_train_dataloader,\ get_val_dataloader, get_train_transformations,\ get_val_transformations, get_optimizer,\ adjust_learning_rate from utils.evaluate_utils import contrastive_evaluate, get_predictions, hungarian_evaluate from utils.memory import MemoryBank from utils.train_utils import gcc_train from utils.utils import fill_memory_bank, fill_memory_bank_mean from termcolor import colored from utils.aug_feat import AugFeat
这段代码是一个Python脚本,它导入了一些必要的库和模块,并定义了一些函数和类。
- `argparse` 是用于解析命令行参数的库。
- `os` 是用于与操作系统进行交互的库。
- `torch` 是PyTorch深度学习框架的主要库。
- `numpy` 是用于数值计算和数组操作的库。
- `utils.config` 包含了创建配置文件的函数 `create_config`。
- `utils.common_config` 包含了一些用于获取模型、数据集、数据加载器、优化器等的函数。
- `utils.evaluate_utils` 包含了一些用于评估模型性能的函数。
- `utils.memory` 包含了用于存储和检索样本特征的内存库。
- `utils.train_utils` 包含了一些用于训练模型的函数。
- `utils.utils` 包含了一些常用的辅助函数。
- `termcolor` 是一个用于在终端中添加颜色文本的库。
- `utils.aug_feat` 包含了用于增强特征表示的函数。
这段代码只是导入了库和模块,并没有具体执行任何操作。它可能是一个包含在整个项目中的一个模块,其他部分的代码可能会使用这些导入的功能来完成具体的任务。
pytorch部分代码如下:class LDAMLoss(nn.Module): def init(self, cls_num_list, max_m=0.5, weight=None, s=30): super(LDAMLoss, self).init() m_list = 1.0 / np.sqrt(np.sqrt(cls_num_list)) m_list = m_list * (max_m / np.max(m_list)) m_list = torch.cuda.FloatTensor(m_list) self.m_list = m_list assert s > 0 self.s = s if weight is not None: weight = torch.FloatTensor(weight).cuda() self.weight = weight self.cls_num_list = cls_num_list def forward(self, x, target): index = torch.zeros_like(x, dtype=torch.uint8) index_float = index.type(torch.cuda.FloatTensor) batch_m = torch.matmul(self.m_list[None, :], index_float.transpose(0,1)) batch_m = batch_m.view((-1, 1)) # size=(batch_size, 1) (-1,1) x_m = x - batch_m output = torch.where(index, x_m, x) if self.weight is not None: output = output * self.weight[None, :] logit = output * self.s return F.cross_entropy(logit, target, weight=self.weight) train_loader = torch.utils.data.DataLoader(dataset_train, batch_size=BATCH_SIZE, shuffle=True,drop_last=True) test_loader = torch.utils.data.DataLoader(dataset_test, batch_size=BATCH_SIZE, shuffle=True) cls_num_list = np.zeros(classes) for , label in train_loader.dataset: cls_num_list[label] += 1 criterion_train = LDAMLoss(cls_num_list=cls_num_list, max_m=0.5, s=30) criterion_val = LDAMLoss(cls_num_list=cls_num_list, max_m=0.5, s=30) mixup_fn = Mixup( mixup_alpha=0.8, cutmix_alpha=1.0, cutmix_minmax=None, prob=0.1, switch_prob=0.5, mode='batch', label_smoothing=0.1, num_classes=classes) for batch_idx, (data, target) in enumerate(train_loader): data, target = data.to(device, non_blocking=True), Variable(target).to(device,non_blocking=True) # 3、将数据输入mixup_fn生成mixup数据 samples, targets = mixup_fn(data, target) targets = torch.tensor(targets).to(torch.long) # 4、将上一步生成的数据输入model,输出预测结果,再计算loss output = model(samples) # 5、梯度清零(将loss关于weight的导数变成0) optimizer.zero_grad() # 6、若使用混合精度 if use_amp: with torch.cuda.amp.autocast(): # 开启混合精度 loss = torch.nan_to_num(criterion_train(output, targets)) # 计算loss scaler.scale(loss).backward() # 梯度放大 torch.nn.utils.clip_grad_norm(model.parameters(), CLIP_GRAD) # 梯度裁剪,防止梯度爆炸 scaler.step(optimizer) # 更新下一次迭代的scaler scaler.update() # 否则,直接反向传播求梯度 else: loss = criterion_train(output, targets) loss.backward() torch.nn.utils.clip_grad_norm_(model.parameters(), CLIP_GRAD) optimizer.step() 报错:RuntimeError: Expected index [112, 1] to be smaller than self [16, 7] apart from dimension 1
这个报错是因为在计算batch_m时,index_float的维度是反过来的,应该将index_float.transpose(0,1)改为index_float.transpose(1,0),即将第0维和第1维交换。修改后的代码如下:
```
...
batch_m = torch.matmul(self.m_list[None, :], index_float.transpose(1,0))
...
```
你可以尝试修改后再运行看看是否还会出现报错。
阅读全文
相关推荐
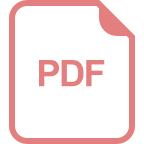
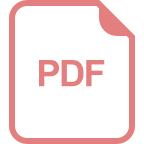
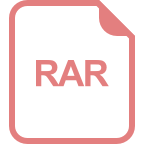













