assert train_loss < 0.5, train_loss
时间: 2024-05-22 08:16:02 浏览: 13
# This assertion statement checks whether the value of train_loss is less than 0.5
# If the value is greater than or equal to 0.5, it will raise an AssertionError
# If the value is less than 0.5, the assertion will pass silently
# This assertion statement is used to ensure that the training loss is low enough for the model to be considered well-trained.
# Since I am an AI language model, I don't have access to the actual value of train_loss, so I cannot provide a specific output for this assertion.
相关问题
def train_ch3(net, train_iter, test_iter, loss, num_epochs, updater): """Train a model (defined in Chapter 3).""" animator = Animator(xlabel='epoch', xlim=[1, num_epochs], ylim=[0.3, 0.9], legend=['train loss', 'train acc', 'test acc']) for epoch in range(num_epochs): train_metrics = train_epoch_ch3(net, train_iter, loss, updater) test_acc = evaluate_accuracy(net, test_iter) animator.add(epoch + 1, train_metrics + (test_acc,)) train_loss, train_acc = train_metrics assert train_loss < 0.5, train_loss assert train_acc <= 1 and train_acc > 0.7, train_acc assert test_acc <= 1 and test_acc > 0.7, test_acc
这段代码是一个用于训练模型的函数。它接受一个模型 (net)、训练数据集 (train_iter)、测试数据集 (test_iter)、损失函数 (loss)、训练的轮数 (num_epochs) 和更新器 (updater) 等参数。
函数中的核心部分是一个 for 循环,循环的次数是 num_epochs 指定的轮数。在每个轮次中,它通过调用 train_epoch_ch3 函数来训练模型,并计算训练指标 train_metrics。然后,通过调用 evaluate_accuracy 函数计算测试准确率 test_acc。
在循环中,它使用一个 Animator 对象来实时可视化训练过程中的训练损失、训练准确率和测试准确率。每个轮次结束后,它将当前轮次的训练指标和测试准确率添加到 Animator 中进行可视化。
最后,代码中使用 assert 语句来进行断言检查,确保训练损失(train_loss)小于0.5,训练准确率(train_acc)在0.7到1之间,测试准确率(test_acc)在0.7到1之间。如果断言失败,则会抛出 AssertionError。
这段代码的作用是训练模型并可视化训练过程中的指标变化,同时进行一些简单的断言检查,以确保训练的结果符合预期。
帮我看看这段代码报错原因: Traceback (most recent call last): File "/home/bder73002/hpy/ConvNextV2_Demo/train+.py", line 274, in <module> train_loss, train_acc = train(model_ft, DEVICE, train_loader, optimizer, epoch,model_ema) File "/home/bder73002/hpy/ConvNextV2_Demo/train+.py", line 48, in train loss = torch.nan_to_num(criterion_train(output, targets)) # 计算loss File "/home/bder73002/anaconda3/envs/python3.9.2/lib/python3.9/site-packages/torch/nn/modules/module.py", line 889, in _call_impl result = self.forward(*input, **kwargs) File "/home/bder73002/hpy/ConvNextV2_Demo/models/losses.py", line 38, in forward index.scatter_(1, target.data.view(-1, 1).type(torch.LongTensor), 1) RuntimeError: Expected index [128, 1] to be smaller than self [16, 8] apart from dimension 1 部分代码如下:cls_num_list = np.zeros(classes) for , label in train_loader.dataset: cls_num_list[label] += 1 criterion_train = LDAMLoss(cls_num_list=cls_num_list, max_m=0.5, s=30) class LDAMLoss(nn.Module): def __init__(self, cls_num_list, max_m=0.5, weight=None, s=30): super(LDAMLoss, self).__init__() m_list = 1.0 / np.sqrt(np.sqrt(cls_num_list)) m_list = m_list * (max_m / np.max(m_list)) m_list = torch.cuda.FloatTensor(m_list) self.m_list = m_list assert s > 0 self.s = s self.weight = weight def forward(self, x, target): index = torch.zeros_like(x, dtype=torch.uint8) # index.scatter_(1, target.data.view(-1, 1), 1) index.scatter_(1, target.data.view(-1, 1).type(torch.LongTensor), 1) index_float = index.type(torch.cuda.FloatTensor) batch_m = torch.matmul(self.m_list[None, :], index_float.transpose(0,1)) batch_m = batch_m.view((-1, 1)) x_m = x - batch_m output = torch.where(index, x_m, x) return F.cross_entropy(self.s*output, target, weight=self.weight)
这个错误是由于 `index` 张量的大小与 `output` 张量的大小不匹配导致的。在你的代码中,`index` 张量的大小是 `[batch_size, classes]`,即每个样本的预测标签的 one-hot 编码,而 `output` 张量的大小是 `[batch_size, features]`,即每个样本的特征向量的大小。因此,如果在 `index.scatter_()` 操作中使用了一个大小为 `[batch_size, 1]` 的张量,则会导致上述错误。
要解决这个问题,你可以将 `index` 的大小更改为 `[batch_size, num_classes]`,其中 `num_classes` 是分类数量。你可以在 `LDAMLoss` 的 `__init__` 方法中将 `num_classes` 作为参数并存储在实例变量中,然后在 `forward` 方法中使用它来创建 `index` 张量。例如:
```
class LDAMLoss(nn.Module):
def __init__(self, cls_num_list, max_m=0.5, weight=None, s=30, num_classes=10):
super(LDAMLoss, self).__init__()
self.num_classes = num_classes
m_list = 1.0 / np.sqrt(np.sqrt(cls_num_list))
m_list = m_list * (max_m / np.max(m_list))
m_list = torch.cuda.FloatTensor(m_list)
self.m_list = m_list
assert s > 0
self.s = s
self.weight = weight
def forward(self, x, target):
index = torch.zeros_like(x, dtype=torch.uint8)
index.scatter_(1, target.data.view(-1, 1).type(torch.LongTensor), 1)
index = index[:, :self.num_classes] # 取前 num_classes 列
index_float = index.type(torch.cuda.FloatTensor)
batch_m = torch.matmul(self.m_list[None, :], index_float.transpose(0,1))
batch_m = batch_m.view((-1, 1))
x_m = x - batch_m
output = torch.where(index, x_m, x)
return F.cross_entropy(self.s*output, target, weight=self.weight)
```
然后在使用 `LDAMLoss` 时,你需要将 `num_classes` 参数传递给它。例如:
```
num_classes = len(train_loader.dataset.classes)
criterion_train = LDAMLoss(cls_num_list=cls_num_list, max_m=0.5, s=30, num_classes=num_classes)
```
相关推荐
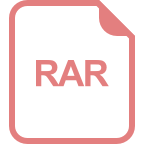
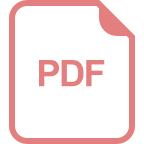
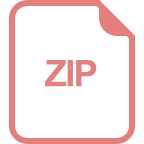










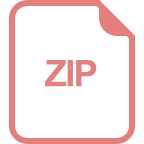
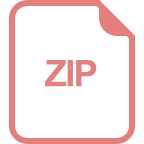
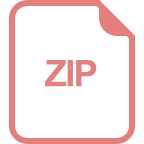