class Conv1D(nn.Conv1d): #对由几个输入平面组成的输入信号应用1D卷积。 def __init__(self, *args, **kwargs): super(Conv1D, self).__init__(*args, **kwargs) def forward(self, x, squeeze=False): print(x.shape) # x: N x C x L if x.dim() not in [2, 3]: raise RuntimeError("{} accept 2/3D tensor as input".format( self.__name__)) x = super().forward(x if x.dim() == 3 else torch.unsqueeze(x, 1))#增加一个维度 print(x.shape) if squeeze: x = torch.squeeze(x) print(x.shape) return x
时间: 2023-12-04 18:03:46 浏览: 127
这是一个使用 PyTorch 实现的 1D 卷积神经网络的类定义。这个类继承自 `nn.Conv1d`。在 `__init__` 方法中,它调用了父类的构造函数。在 `forward` 方法中,它首先检查输入的张量维度是否是 2 或 3,如果不是则抛出一个异常。接着它调用了父类的 `forward` 方法进行卷积操作,如果输入张量的维度是 2,则需要在第二维上增加一个维度。最后根据参数 `squeeze` 是否为 True 来决定是否要去除张量中维度为 1 的维度。该方法返回处理后的张量。
相关问题
解释class Bottlrneck(torch.nn.Module): def __init__(self,In_channel,Med_channel,Out_channel,downsample=False): super(Bottlrneck, self).__init__() self.stride = 1 if downsample == True: self.stride = 2 self.layer = torch.nn.Sequential( torch.nn.Conv1d(In_channel, Med_channel, 1, self.stride), torch.nn.BatchNorm1d(Med_channel), torch.nn.ReLU(), torch.nn.Conv1d(Med_channel, Med_channel, 3, padding=1), torch.nn.BatchNorm1d(Med_channel), torch.nn.ReLU(), torch.nn.Conv1d(Med_channel, Out_channel, 1), torch.nn.BatchNorm1d(Out_channel), torch.nn.ReLU(), ) if In_channel != Out_channel: self.res_layer = torch.nn.Conv1d(In_channel, Out_channel,1,self.stride) else: self.res_layer = None def forward(self,x): if self.res_layer is not None: residual = self.res_layer(x) else: residual = x return self.layer(x)+residual
这是一个使用 1x1、3x3、1x1 卷积核的瓶颈块(Bottleneck Block)。它的作用是减少参数数量并增加网络深度,同时减少梯度消失问题。具体来说,它的结构如下:
- 输入 In_channel 经过一个 1x1 的卷积核,输出通道数变为 Med_channel。
- 经过 Batch Normalization 和 ReLU 激活函数。
- 再经过一个 3x3 的卷积核,输出通道数还是 Med_channel。
- 经过 Batch Normalization 和 ReLU 激活函数。
- 最后经过一个 1x1 的卷积核,输出通道数变为 Out_channel。
- 经过 Batch Normalization 和 ReLU 激活函数。
如果 downsample 设置为 True,表示需要对输入进行下采样,此时会在第一个 1x1 卷积层后加一个步长为 2 的卷积操作。
同时,为了保证输入输出通道数相同,如果 In_channel 不等于 Out_channel,则会在最后加上一个 1x1 的卷积层将输入通道数转化为输出通道数,否则不需要进行这样的操作。
forward 函数中,首先判断是否需要进行输入通道数与输出通道数的转换,然后将输入 x 经过瓶颈块的处理得到的结果与 residual 相加作为最终输出。其中 residual 表示输入 x 经过最后的 1x1 卷积层得到的结果。
叙述下列代码的实现流程class Bottlrneck(torch.nn.Module): def __init__(self,In_channel,Med_channel,Out_channel,downsample=False): super(Bottlrneck, self).__init__() self.stride = 1 if downsample == True: self.stride = 2 self.layer = torch.nn.Sequential( torch.nn.Conv1d(In_channel, Med_channel, 1, self.stride), torch.nn.BatchNorm1d(Med_channel), torch.nn.ReLU(), torch.nn.Conv1d(Med_channel, Med_channel, 3, padding=1), torch.nn.BatchNorm1d(Med_channel), torch.nn.ReLU(), torch.nn.Conv1d(Med_channel, Out_channel, 1), torch.nn.BatchNorm1d(Out_channel), torch.nn.ReLU(), ) if In_channel != Out_channel: self.res_layer = torch.nn.Conv1d(In_channel, Out_channel,1,self.stride) else: self.res_layer = None def forward(self,x): if self.res_layer is not None: residual = self.res_layer(x) else: residual = x return self.layer(x)+residual
这段代码实现了一个 Bottleneck 模块,用于卷积神经网络中的特征提取。它包含了三个 1x1、3x3、1x1 的卷积层,以及对应的 Batch Normalization 和 ReLU 激活函数。
在初始化时,输入参数包括输入通道数 In_channel、中间通道数 Med_channel、输出通道数 Out_channel,以及一个 downsample 布尔值,用于表示是否需要下采样。在初始化过程中,会根据 downsample 值来确定是否需要下采样,如果需要则将步长 stride 设为 2,否则设为默认值 1。
接下来,会创建一个 Sequential 模块,作为 Bottleneck 模块的核心部分。该 Sequential 模块包含了三个卷积层,分别是一个 1x1 卷积层、一个 3x3 卷积层、以及另一个 1x1 卷积层。这三个卷积层之间都添加了 Batch Normalization 和 ReLU 激活函数,用于提高模型的性能。
如果输入通道数 In_channel 不等于输出通道数 Out_channel,则还需要添加一个 1x1 卷积层,将输入数据的通道数调整为输出通道数。这个卷积层也被称为残差连接,用于解决深度神经网络训练过程中的梯度消失问题。
最后,通过 forward 方法来实现前向传播。如果存在残差连接,则首先通过 1x1 卷积层将输入数据进行通道数调整,然后将调整后的数据与 Bottleneck 模块的输出数据相加,并返回相加后的结果。如果不存在残差连接,则直接返回 Bottleneck 模块的输出数据。
阅读全文
相关推荐

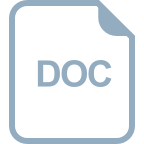














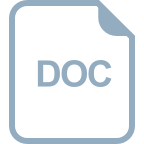