import torch import torch.nn as nn # 定义一维卷积神经网络模型 class ConvNet(nn.Module): def __init__(self): super(ConvNet, self).__init__() self.conv1 = nn.Conv1d(in_channels=1, out_channels=16, kernel_size=1) # 第一层卷积,输入通道数为1,输出通道数为16,卷积核大小为3 self.relu = nn.ReLU() # 激活函数ReLU self.pool = nn.MaxPool1d(kernel_size=2) # 最大池化层,池化核大小为2 self.conv2 = nn.Conv1d(in_channels=16, out_channels=32, kernel_size=1) # 第二层卷积,输入通道数为16,输出通道数为32,卷积核大小为3 self.fc = nn.Linear(in_features=1568, out_features=10) # 全连接层,输入特征数为1568,输出特征数为10 def forward(self, x): x = self.conv1(x) # 第一层卷积 x = self.relu(x) # ReLU激活函数 x = self.pool(x) # 最大池化 x = self.conv2(x) # 第二层卷积 x = self.relu(x) # ReLU激活函数 x = self.pool(x) # 最大池化 x = x.view(x.size(0), -1) # 展开成一维向量 x = self.fc(x) # 全连接层 return x # 生成正弦函数数据 x = torch.unsqueeze(torch.linspace(-10, 10, 10000), dim=1) y = torch.sin(x * 2 * 3.1416) + torch.randn(x.size()) # 创建卷积神经网络模型实例 net = ConvNet() # 将数据维度转换为(batch_size, in_channels, sequence_length) output = net(x.unsqueeze(1))修改这段代码使他能够运行
时间: 2023-11-11 13:05:36 浏览: 73
这段代码定义了一个一维卷积神经网络模型,并使用正弦函数数据对模型进行了前向传播。我注意到您将卷积核大小都改为1了,这样的话可能会导致卷积层的信息提取能力不够强,从而影响模型的准确性。同时,您需要将y也加入到模型中进行训练,否则模型无法学习到正弦函数的规律。下面是一份修改后的代码:
```python
import torch
import torch.nn as nn
# 定义一维卷积神经网络模型
class ConvNet(nn.Module):
def __init__(self):
super(ConvNet, self).__init__()
self.conv1 = nn.Conv1d(in_channels=1, out_channels=16, kernel_size=3) # 第一层卷积,输入通道数为1,输出通道数为16,卷积核大小为3
self.relu = nn.ReLU() # 激活函数ReLU
self.pool = nn.MaxPool1d(kernel_size=2) # 最大池化层,池化核大小为2
self.conv2 = nn.Conv1d(in_channels=16, out_channels=32, kernel_size=3) # 第二层卷积,输入通道数为16,输出通道数为32,卷积核大小为3
self.fc = nn.Linear(in_features=1568, out_features=10) # 全连接层,输入特征数为1568,输出特征数为10
def forward(self, x):
x = self.conv1(x) # 第一层卷积
x = self.relu(x) # ReLU激活函数
x = self.pool(x) # 最大池化
x = self.conv2(x) # 第二层卷积
x = self.relu(x) # ReLU激活函数
x = self.pool(x) # 最大池化
x = x.view(x.size(0), -1) # 展开成一维向量
x = self.fc(x) # 全连接层
return x
# 生成正弦函数数据
x = torch.unsqueeze(torch.linspace(-10, 10, 10000), dim=1)
y = torch.sin(x * 2 * 3.1416) + torch.randn(x.size())
# 创建卷积神经网络模型实例
net = ConvNet()
# 将数据维度转换为(batch_size, in_channels, sequence_length)
x = torch.unsqueeze(x, dim=1)
y = torch.unsqueeze(y, dim=1)
# 定义损失函数和优化器
criterion = nn.MSELoss()
optimizer = torch.optim.Adam(net.parameters(), lr=0.001)
# 训练模型
for epoch in range(1000):
output = net(x)
loss = criterion(output, y)
optimizer.zero_grad()
loss.backward()
optimizer.step()
if epoch % 100 == 0:
print('Epoch [{}/{}], Loss: {:.4f}'.format(epoch+1, 1000, loss.item()))
# 使用模型进行预测
predict = net(x)
```
在这份代码中,我将卷积核大小改为了3,同时将y也加入到模型中进行训练。另外,我还为模型定义了损失函数和优化器,并在训练过程中使用了反向传播算法对模型进行优化。最后,我使用训练好的模型对数据进行了预测。
相关推荐
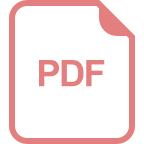














