MemoryError: Unable to allocate 12.0 GiB for an array with shape (664845, 11, 11, 20) and data type float64
时间: 2023-11-12 22:16:44 浏览: 27
这个错误表明你的计算机没有足够的内存来分配一个大小为12.0 GiB的数组。你可以尝试一些解决方法,如:
1. 使用更小的数据集或减少输入数据的维度。
2. 优化代码,减少内存使用量。
3. 使用更高内存的计算机或云计算平台。
4. 尝试使用数据增强或降维技术来减小数据集的大小。
你可以根据具体情况采取适当的方法来解决这个问题。
相关问题
MemoryError: Unable to allocate 25.2 GiB for an array with shape (58104, 58104) and data type float64
This error message occurs when the program tries to allocate more memory than the system can provide. In this case, the program is attempting to allocate 25.2 GiB of memory for an array with shape (58104, 58104) and data type float64, but the system does not have enough free memory to accommodate this request.
To resolve this error, you can try the following solutions:
1. Increase the available memory: If possible, try to free up some memory by closing other programs or processes that are running on the system. You can also consider upgrading the RAM on your computer to increase the available memory.
2. Use a more memory-efficient data type: If the data in the array does not require the precision of float64, you can consider using a lower precision data type, such as float32 or even int32 or int16, depending on the range of the data.
3. Use a sparse matrix: If the array contains mostly zeros, you can consider using a sparse matrix representation, which only stores the non-zero values and their indices. This can significantly reduce the memory requirements of the array.
4. Use a distributed computing framework: If the array is too large to fit in the memory of a single machine, you can consider using a distributed computing framework, such as Apache Spark or Dask, to distribute the computation across multiple machines.
MemoryError: Unable to allocate 9.63 GiB for an array with shape (1292820000,) and data type float64
这个错误是因为你的代码尝试在内存中分配一个过大的数组,超出了系统的内存限制。可能的解决方案包括:
1. 优化你的代码,减少内存使用量。
2. 增加系统的物理内存或者虚拟内存。
3. 使用分布式计算或者分批处理数据,避免一次性处理过大的数据量。
4. 选择一种更节省内存的数据结构或算法。
你可以根据具体情况选择合适的解决方案。
相关推荐
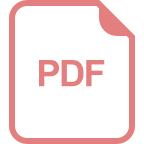
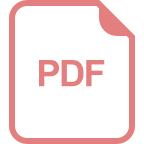
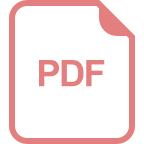












