MemoryError: Unable to allocate 9.63 GiB for an array with shape (1292820000,) and data type float64解决问题的代码
时间: 2023-07-24 08:35:36 浏览: 71
这个错误是由于需要创建的数组太大而导致内存不足。为了解决这个问题,你可以尝试使用以下两种方法之一:
1. 减少数组的大小,或者使用其他更节省内存的数据结构。
2. 为 Python 解释器分配更多的内存。
下面是第二种方法的代码示例:
```python
import numpy as np
import sys
# 设置数组大小
n = 1000000000
# 打印当前 Python 解释器的内存限制
print('Python interpreter memory limit:', sys.maxsize)
# 尝试创建一个足够大的数组
try:
arr = np.zeros(n, dtype=np.float64)
print('Array created successfully!')
except MemoryError:
print('MemoryError: Unable to allocate memory for the array. Try increasing the memory limit.')
```
这个代码示例中,我们首先设置要创建的数组的大小,然后打印当前 Python 解释器的内存限制。接着,我们尝试创建一个足够大的数组,如果内存不足,则会抛出 `MemoryError` 异常。如果创建成功,则会打印一条成功的消息。如果创建失败,则可以尝试为 Python 解释器分配更多的内存,例如在命令行中使用 `-Xmx` 参数。
相关问题
MemoryError: Unable to allocate 9.63 GiB for an array with shape (1292820000,) and data type float64
这个错误是因为你的代码尝试在内存中分配一个过大的数组,超出了系统的内存限制。可能的解决方案包括:
1. 优化你的代码,减少内存使用量。
2. 增加系统的物理内存或者虚拟内存。
3. 使用分布式计算或者分批处理数据,避免一次性处理过大的数据量。
4. 选择一种更节省内存的数据结构或算法。
你可以根据具体情况选择合适的解决方案。
MemoryError: Unable to allocate 25.2 GiB for an array with shape (58104, 58104) and data type float64
This error message occurs when the program tries to allocate more memory than the system can provide. In this case, the program is attempting to allocate 25.2 GiB of memory for an array with shape (58104, 58104) and data type float64, but the system does not have enough free memory to accommodate this request.
To resolve this error, you can try the following solutions:
1. Increase the available memory: If possible, try to free up some memory by closing other programs or processes that are running on the system. You can also consider upgrading the RAM on your computer to increase the available memory.
2. Use a more memory-efficient data type: If the data in the array does not require the precision of float64, you can consider using a lower precision data type, such as float32 or even int32 or int16, depending on the range of the data.
3. Use a sparse matrix: If the array contains mostly zeros, you can consider using a sparse matrix representation, which only stores the non-zero values and their indices. This can significantly reduce the memory requirements of the array.
4. Use a distributed computing framework: If the array is too large to fit in the memory of a single machine, you can consider using a distributed computing framework, such as Apache Spark or Dask, to distribute the computation across multiple machines.
相关推荐
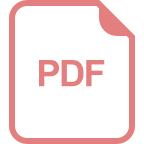
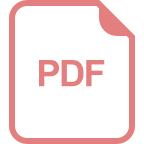
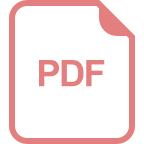












