class ImageDataset(Dataset): def init( self, resolution, image_paths, classes=None, shard=0, num_shards=1, random_crop=False, random_flip=False, ): super().init() self.resolution = resolution self.local_images = image_paths[shard:][::num_shards] self.local_classes = None if classes is None else classes[shard:][::num_shards] self.random_crop = random_crop # 随机裁剪 self.random_flip = random_flip # 随机翻转 def len(self): return len(self.local_images) # 获取数据集的数量,对于类而言,len()函数是没有办法直接计算类的长度的,如果在类中没有定义__len__()方法 # 来指明程序到底该计算哪个属性的长度时,在终端我们必须采用len(对象.属性)才能得到我们想要的结果。 def getitem(self, idx): path = self.local_images[idx] with bf.BlobFile(path, "rb") as f: pil_image = Image.open(f) pil_image.load() pil_image = pil_image.convert("RGB") if self.random_crop: arr = random_crop_arr(pil_image, self.resolution) else: arr = center_crop_arr(pil_image, self.resolution) if self.random_flip and random.random() < 0.5: arr = arr[:, ::-1] arr = arr.astype(np.float32) / 127.5 - 1 out_dict = {} if self.local_classes is not None: out_dict["y"] = np.array(self.local_classes[idx], dtype=np.int64) return np.transpose(arr, [2, 0, 1]), out_dict,调用ImageDataset类时如果 dataset = ImageDataset( image_size, all_files, classes=classes, shard=MPI.COMM_WORLD.Get_rank(), num_shards=MPI.COMM_WORLD.Get_size(), random_crop=random_crop, random_flip=random_flip, )这样调用,getitem方法中的索引idx是什么
时间: 2023-06-25 16:05:01 浏览: 40
在调用ImageDataset类时,idx是指要获取的数据的索引,也就是在整个数据集中要获取哪个数据的信息。getitem方法会根据这个索引来读取对应的图片信息,并进行预处理操作。在这个方法中,首先会读取对应索引的图片路径,然后使用Pillow库中的Image.open()方法读取图片信息,接着进行随机裁剪和随机翻转等预处理操作,最后返回处理后的图片信息和对应的标签信息(如果有标签的话)。
相关问题
class ImageDataset(Dataset): def init( self, resolution, image_paths, classes=None, shard=0, num_shards=1, random_crop=False, random_flip=True, ): super().init() self.resolution = resolution self.local_images = image_paths[shard:][::num_shards] self.local_classes = None if classes is None else classes[shard:][::num_shards] self.random_crop = random_crop self.random_flip = random_flip 里面的self.resolution如何实例化
`self.resolution` 是在 `ImageDataset` 类的构造函数中传递的参数,因此需要在创建 `ImageDataset` 对象时进行实例化。例如:
```
dataset = ImageDataset(resolution=(256, 256), image_paths=image_paths, classes=classes, shard=0, num_shards=1, random_crop=True, random_flip=True)
```
在这个例子中,`resolution` 被实例化为一个元组 `(256, 256)`,并传递给了 `ImageDataset` 的构造函数。
class ImageDataset(Dataset): def __init__(self, image_paths): super().__init__() self.image_paths = image_paths self.transform = albumentations.Compose([ albumentations.RandomCrop(height=128, width=128) ]) self.cutout = albumentations.Cutout(num_holes=12, max_h_size=24, max_w_size=24, p=1.0, fill_value=1.0) def __len__(self): return len(self.image_paths) def __getitem__(self, index): image = cv2.imread(self.image_paths[index]) image = cv2.cvtColor(image, cv2.COLOR_BGR2GRAY) / 255.0 image = self.transform(image=image)['image'] image_cutout = self.cutout(image=image)['image'] image = image.reshape((1, image.shape[0], image.shape[1])) image_cutout = image_cutout.reshape((1, image_cutout.shape[0], image_cutout.shape[1])) mask = (image_cutout != 1.0) * 1.0 return image, image_cutout, mask
这段代码是一个PyTorch中的自定义数据集类(ImageDataset),用于加载图像数据并进行数据增强操作。以下是对每个方法的解释:
1. `__init__(self, image_paths)`:构造函数,接收一个包含所有图像路径的列表作为输入参数,并将其存储在成员变量`self.image_paths`中。
2. `__len__(self)`:返回数据集中图像的数量。
3. `__getitem__(self, index)`:根据给定的索引,加载对应路径的图像,并进行数据增强操作。具体步骤如下:
- 使用OpenCV的`cv2.imread()`函数加载图像。
- 将图像从BGR格式转换为灰度图像,并将像素值归一化到0到1之间。
- 使用albumentations库中的`Compose()`函数创建一个数据增强管道,其中包含一个随机裁剪操作(RandomCrop)。
- 使用`Cutout()`函数在图像中随机遮挡12个小区域,模拟数据缺失的情况,从而增强模型的泛化性能。
- 将图像和遮挡后的图像reshape为单通道的张量,并创建一个二值掩码(mask)表示遮挡的区域。
- 返回原图、遮挡后的图像和二值掩码。
相关推荐
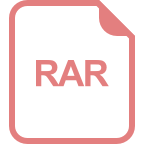














