在matlab中以下代码有什么问题load('train.mat'); X = train(:,1:9).'; Y = train(:,10).'; net = newff(X,Y,[12 12],{'logsig' 'tansig'},'traingdm'); net.trainParam.epochs = 1000; net.trainParam.min_grad =1e-24; net.trainParam.lr = 0.01; net = train(net,X,Y);
时间: 2023-06-26 07:07:03 浏览: 36
这段代码本身没有语法错误,但可能存在以下问题:
1. 数据加载问题:`train.mat`文件是否存在,且其中的数据格式是否正确?
2. 神经网络结构问题:神经网络的结构是否合理?输入层节点数为9,输出层节点数为1,但是隐含层的节点数为12,不一定是最优的选择。需要根据具体的问题和数据集来选择合适的网络结构。
3. 训练参数设置问题:`min_grad`参数设置为1e-24可能过于小,导致训练过程很难收敛;`lr`参数设置为0.01可能过于大,导致训练过程波动较大。需要根据具体的问题和数据集来调整训练参数。
4. 训练数据划分问题:代码中没有对数据进行划分,可能会导致模型的过拟合。应该将数据集划分为训练集、验证集和测试集,以评估模型的泛化能力。
因此,建议在使用该代码时,根据具体的问题和数据集进行适当的修改和调整。
相关问题
写一个适用于一下代码的能运行出matlab图的代码% 加载数据 load fisheriris.mat X = meas'; Y = zeros(3,length(species)); for i = 1:length(species) if strcmp(species{i},'setosa') Y(1,i) = 1; elseif strcmp(species{i},'versicolor') Y(2,i) = 1; elseif strcmp(species{i},'virginica') Y(3,i) = 1; end end % 将数据集分为训练集和测试集 [trainInd,testInd] = dividerand(length(species),0.7,0.3); X_train = X(:,trainInd); Y_train = Y(:,trainInd); X_test = X(:,testInd); Y_test = Y(:,testInd); % 设置神经网络参数 inputSize = size(X_train,1); hiddenSize = 10; outputSize = size(Y_train,1); net = feedforwardnet(hiddenSize); % 训练神经网络 net.trainFcn = 'trainlm'; net.trainParam.epochs = 100; net.trainParam.goal = 0.01; net = train(net,X_train,Y_train); % 测试神经网络 Y_pred = net(X_test); [~,Y_pred] = max(Y_pred); [~,Y_test] = max(Y_test); % 计算准确率 accuracy = sum(Y_pred == Y_test)/length(Y_test); disp(['Accuracy: ',num2str(accuracy*100),'%']);
以下是适用于上述代码的 MATLAB 代码,可以生成分类结果的可视化图表:
```
% 加载数据
load fisheriris.mat
X = meas';
Y = zeros(3,length(species));
for i = 1:length(species)
if strcmp(species{i},'setosa')
Y(1,i) = 1;
elseif strcmp(species{i},'versicolor')
Y(2,i) = 1;
elseif strcmp(species{i},'virginica')
Y(3,i) = 1;
end
end
% 将数据集分为训练集和测试集
[trainInd,testInd] = dividerand(length(species),0.7,0.3);
X_train = X(:,trainInd);
Y_train = Y(:,trainInd);
X_test = X(:,testInd);
Y_test = Y(:,testInd);
% 设置神经网络参数
inputSize = size(X_train,1);
hiddenSize = 10;
outputSize = size(Y_train,1);
net = feedforwardnet(hiddenSize);
% 训练神经网络
net.trainFcn = 'trainlm';
net.trainParam.epochs = 100;
net.trainParam.goal = 0.01;
net = train(net,X_train,Y_train);
% 测试神经网络
Y_pred = net(X_test);
[~,Y_pred] = max(Y_pred);
[~,Y_test] = max(Y_test);
% 计算准确率
accuracy = sum(Y_pred == Y_test)/length(Y_test);
disp(['Accuracy: ',num2str(accuracy*100),'%']);
% 绘制分类结果图表
figure;
gscatter(X_test(1,:),X_test(2,:),Y_pred);
hold on;
gscatter(X_test(1,:),X_test(2,:),Y_test,'k','o',8);
xlabel('Sepal length');
ylabel('Sepal width');
legend('Predicted Setosa','Predicted Versicolor','Predicted Virginica','Actual Setosa','Actual Versicolor','Actual Virginica','Location','best');
```
这段代码会生成一个散点图,其中预测的分类结果用不同颜色的点表示,实际分类结果用黑色圆圈表示。图表的横坐标和纵坐标分别表示花萼长度和花萼宽度。图表还包括一个图例,显示了预测的三个分类和实际的三个分类。
MATLAB中没有mnist_train.mat的文件怎么下载
您可以从以下网址下载MNIST数据集:
http://yann.lecun.com/exdb/mnist/
该网站包含四个文件:train-images-idx3-ubyte.gz,train-labels-idx1-ubyte.gz,t10k-images-idx3-ubyte.gz和t10k-labels-idx1-ubyte.gz。这些文件包含了60,000个训练样本和10,000个测试样本的手写数字图像和对应的标签。
您需要下载这四个文件并解压缩它们。然后,您可以使用MATLAB中的load命令加载数据集。例如,如果您将数据集文件保存在路径C:\MNIST中,可以使用以下命令加载训练数据:
```matlab
load('C:\MNIST\train-images-idx3-ubyte', '-mat');
load('C:\MNIST\train-labels-idx1-ubyte', '-mat');
```
请注意,您需要将路径替换为包含数据集文件的实际路径。
一旦您加载了数据集,您就可以使用MATLAB中的其他函数来处理和分析数据。例如,您可以使用imshow函数显示图像,使用plot函数绘制图表,使用hist函数绘制直方图等等。
相关推荐
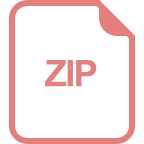
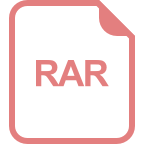
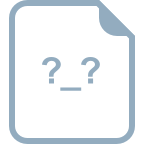












