使用Tensorflow实现DCGAN来生成手写数字的详细代码
时间: 2024-03-23 13:37:55 浏览: 19
好的,以下是使用Tensorflow实现DCGAN生成手写数字的详细代码:
首先,我们需要导入必要的库和数据集。这里我们使用Tensorflow自带的MNIST数据集。
```python
import tensorflow as tf
import numpy as np
import matplotlib.pyplot as plt
from tensorflow.examples.tutorials.mnist import input_data
mnist = input_data.read_data_sets("MNIST_data/")
```
接下来,我们定义生成器和判别器的网络结构。生成器的输入是一个随机噪声,输出是一张28x28的手写数字图片。判别器的输入是一张28x28的手写数字图片,输出是一个判别得分,表示这张图片是真实图片的概率。
```python
def generator(z, reuse=None):
with tf.variable_scope('gen', reuse=reuse):
hidden1 = tf.layers.dense(inputs=z, units=128, activation=tf.nn.leaky_relu)
hidden2 = tf.layers.dense(inputs=hidden1, units=256, activation=tf.nn.leaky_relu)
output = tf.layers.dense(inputs=hidden2, units=784, activation=tf.nn.tanh)
return output
def discriminator(X, reuse=None):
with tf.variable_scope('dis', reuse=reuse):
hidden1 = tf.layers.dense(inputs=X, units=128, activation=tf.nn.leaky_relu)
hidden2 = tf.layers.dense(inputs=hidden1, units=256, activation=tf.nn.leaky_relu)
logits = tf.layers.dense(inputs=hidden2, units=1)
output = tf.sigmoid(logits)
return output, logits
```
然后,我们定义生成器和判别器的输入和损失函数。其中,生成器的损失函数是生成的假图片与真实图片的差异,判别器的损失函数是真实图片和假图片的区分度。
```python
real_images = tf.placeholder(tf.float32, shape=[None, 784])
z = tf.placeholder(tf.float32, shape=[None, 100])
G = generator(z)
D_output_real, D_logits_real = discriminator(real_images)
D_output_fake, D_logits_fake = discriminator(G, reuse=True)
def loss_func(logits_in, labels_in):
return tf.reduce_mean(tf.nn.sigmoid_cross_entropy_with_logits(logits=logits_in, labels=labels_in))
D_real_loss = loss_func(D_logits_real, tf.ones_like(D_logits_real) * 0.9)
D_fake_loss = loss_func(D_logits_fake, tf.zeros_like(D_logits_fake))
D_loss = D_real_loss + D_fake_loss
G_loss = loss_func(D_logits_fake, tf.ones_like(D_logits_fake))
```
接下来,我们定义生成器和判别器的优化器,并且训练模型。
```python
lr = 0.001
tvars = tf.trainable_variables()
d_vars = [var for var in tvars if 'dis' in var.name]
g_vars = [var for var in tvars if 'gen' in var.name]
D_trainer = tf.train.AdamOptimizer(lr).minimize(D_loss, var_list=d_vars)
G_trainer = tf.train.AdamOptimizer(lr).minimize(G_loss, var_list=g_vars)
batch_size = 100
epochs = 100
init = tf.global_variables_initializer()
samples = []
with tf.Session() as sess:
sess.run(init)
for epoch in range(epochs):
num_batches = mnist.train.num_examples // batch_size
for i in range(num_batches):
batch = mnist.train.next_batch(batch_size)
batch_images = batch[0].reshape((batch_size, 784))
batch_images = batch_images * 2 - 1
batch_z = np.random.uniform(-1, 1, size=(batch_size, 100))
_ = sess.run(D_trainer, feed_dict={real_images: batch_images, z: batch_z})
_ = sess.run(G_trainer, feed_dict={z: batch_z})
print("Epoch:", epoch)
sample_z = np.random.uniform(-1, 1, size=(1, 100))
gen_sample = sess.run(generator(z, reuse=True),feed_dict={z: sample_z})
samples.append(gen_sample)
plt.imshow(samples[0].reshape(28,28))
plt.show()
```
最后,我们可以看到生成的手写数字图片。随着训练的迭代次数增加,生成的图片会越来越真实。
相关推荐
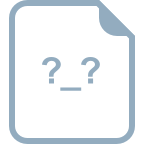














