请分析一下下面代码from scipy.sparse import csgraph from scipy import sparse w = sparse.dok_matrix((4,4)) edges = [(0, 1, 10), (1, 2, 5), (0, 2, 3), (2, 3, 7), (3, 0, 4), (3, 2, 6)] for i, j, v in edges: w[i, j] = v d, p = csgraph.dijkstra(csgraph=w, directed=True, indices=0, return_predecessors=True) print(d) print(p)
时间: 2023-09-19 21:09:10 浏览: 42
这段代码使用了Scipy库来实现有向图的最短路径搜索。其中,使用了稀疏矩阵(dok_matrix)来存储图的边权重,edges列表则存储了边的起始点和终止点,以及它们之间的权重。在循环中,将每个边的权重存储到稀疏矩阵w中。
接着,使用了Scipy库中的csgraph.dijkstra函数来实现有向图的最短路径搜索。这个函数接受的参数包括:csgraph参数表示图的邻接矩阵,directed参数表示图是否为有向图,indices参数表示起点的索引,return_predecessors参数表示是否返回前驱节点。函数返回一个包含最短路径长度的一维数组d和一个包含最短路径前驱节点索引的二维数组p。
最后,打印出最短路径长度数组d和最短路径前驱节点索引数组p。
相关问题
from scipy.sparse import csr_matrix,hstack
您好!您提到了 `scipy.sparse` 的 `csr_matrix` 和 `hstack`。这两个函数都是用于处理稀疏矩阵的。
`csr_matrix` 是 `scipy.sparse` 中的一个函数,用于创建一个压缩稀疏行(Compressed Sparse Row)矩阵。这种矩阵存储方式主要适用于行操作更频繁的情况。通过使用 `csr_matrix`,可以有效地存储和操作大规模的稀疏矩阵。
`hstack` 是 `scipy.sparse` 中的另一个函数,用于将稀疏矩阵按水平方向堆叠起来。它接受一个包含稀疏矩阵的元组或列表,并返回堆叠后的稀疏矩阵。堆叠后的矩阵将具有与输入矩阵相同的行数,并且列数等于所有输入矩阵的列数之和。
这些函数的使用可以帮助您在处理大规模稀疏数据时进行高效计算和存储。如果您对这些函数有进一步的问题或需要更多帮助,请随时提问!
isomap_approx = scipy.sparse.csgraph.shortest_path(isomap_approx, directed=False) isomap_true = scipy.sparse.csgraph.shortest_path(isomap_true, directed=False) isomap_measured = scipy.sparse.csgraph.shortest_path(isomap_measured, directed=False)转matlab
在 MATLAB 中,你可以使用 `graphallshortestpaths` 函数来实现类似的功能。下面是将上述代码转换为 MATLAB 语法的方式:
```matlab
isomap_approx = graphallshortestpaths(sparse(isomap_approx));
isomap_true = graphallshortestpaths(sparse(isomap_true));
isomap_measured = graphallshortestpaths(sparse(isomap_measured));
```
在 MATLAB 中,`graphallshortestpaths` 函数用于计算图中所有节点之间的最短路径。在这里,我们使用 `sparse` 函数将稀疏矩阵 `isomap_approx`、`isomap_true` 和 `isomap_measured` 转换为稀疏图,然后将其作为参数传递给 `graphallshortestpaths` 函数。结果会覆盖原始变量 `isomap_approx`、`isomap_true` 和 `isomap_measured`。请确保你已经加载了 MATLAB 中的 Graph Theory Toolbox,以便使用 `graphallshortestpaths` 函数。
相关推荐
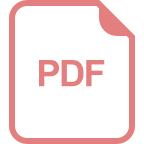
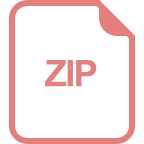
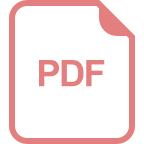












