定义能够使用edgeconnect的InpaintingModel_gen.pth预训练模型的生成器和代码:
时间: 2023-06-18 16:01:53 浏览: 115
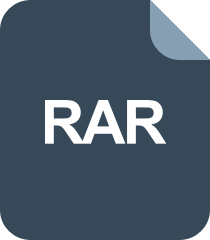
基于python的中文预训练生成模型代码实现

首先,我们需要安装以下依赖库:
- PyTorch
- torchvision
- numpy
- opencv-python
- pillow
然后,我们可以使用以下代码定义生成器模型和使用预训练模型进行图像修复的代码:
```python
import torch
import torch.nn as nn
import numpy as np
import cv2
from PIL import Image
import torchvision.transforms as transforms
class InpaintingGenerator(nn.Module):
def __init__(self):
super(InpaintingGenerator, self).__init__()
self.encoder = nn.Sequential(
nn.Conv2d(3, 64, kernel_size=5, stride=1, padding=2),
nn.BatchNorm2d(64),
nn.ReLU(inplace=True),
nn.Conv2d(64, 128, kernel_size=3, stride=2, padding=1),
nn.BatchNorm2d(128),
nn.ReLU(inplace=True),
nn.Conv2d(128, 256, kernel_size=3, stride=2, padding=1),
nn.BatchNorm2d(256),
nn.ReLU(inplace=True),
nn.Conv2d(256, 512, kernel_size=3, stride=2, padding=1),
nn.BatchNorm2d(512),
nn.ReLU(inplace=True),
nn.Conv2d(512, 512, kernel_size=3, stride=2, padding=1),
nn.BatchNorm2d(512),
nn.ReLU(inplace=True),
nn.Conv2d(512, 512, kernel_size=3, stride=2, padding=1),
nn.BatchNorm2d(512),
nn.ReLU(inplace=True)
)
self.decoder = nn.Sequential(
nn.ConvTranspose2d(512, 512, kernel_size=4, stride=2, padding=1),
nn.BatchNorm2d(512),
nn.ReLU(inplace=True),
nn.ConvTranspose2d(512, 512, kernel_size=4, stride=2, padding=1),
nn.BatchNorm2d(512),
nn.ReLU(inplace=True),
nn.ConvTranspose2d(512, 256, kernel_size=4, stride=2, padding=1),
nn.BatchNorm2d(256),
nn.ReLU(inplace=True),
nn.ConvTranspose2d(256, 128, kernel_size=4, stride=2, padding=1),
nn.BatchNorm2d(128),
nn.ReLU(inplace=True),
nn.ConvTranspose2d(128, 64, kernel_size=4, stride=2, padding=1),
nn.BatchNorm2d(64),
nn.ReLU(inplace=True),
nn.ConvTranspose2d(64, 3, kernel_size=5, stride=1, padding=2),
nn.Tanh()
)
def forward(self, x):
x = self.encoder(x)
x = self.decoder(x)
return x
# 使用预训练的模型进行图像修复
def inpaint_image(image_path, mask_path, model_path):
device = torch.device("cuda" if torch.cuda.is_available() else "cpu")
model = InpaintingGenerator().to(device)
model.load_state_dict(torch.load(model_path))
transform = transforms.Compose([
transforms.Resize((256, 256)),
transforms.ToTensor(),
transforms.Normalize(mean=[0.5, 0.5, 0.5], std=[0.5, 0.5, 0.5])
])
image = Image.open(image_path).convert("RGB")
mask = cv2.imread(mask_path, 0)
mask = cv2.resize(mask, (256, 256))
mask = (mask != 0).astype(np.uint8)
mask = cv2.merge([mask]*3)
image = transform(image).unsqueeze(0).to(device)
mask = torch.from_numpy(mask).permute(2, 0, 1).unsqueeze(0).float().to(device)
with torch.no_grad():
output = model(image * mask)
output = (output * 0.5) + 0.5
output = output.squeeze(0).permute(1, 2, 0).cpu().numpy()
output = (output * 255).astype(np.uint8)
output = cv2.cvtColor(output, cv2.COLOR_BGR2RGB)
return output
```
使用以上代码,我们可以将预训练模型 InpaintingModel_gen.pth 用于图像修复。我们只需要调用 `inpaint_image` 函数,并传入需要修复的图像路径、遮挡图路径和预训练模型路径即可。
阅读全文
相关推荐
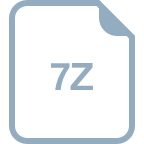
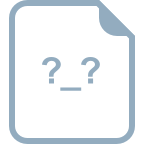







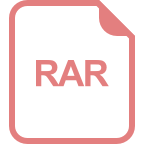
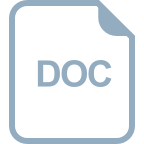




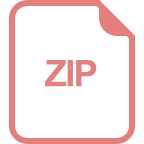