NameError: name 'input_data' is not defined
时间: 2023-10-10 08:11:25 浏览: 143
这个错误通常是因为代码中使用了一个没有定义的变量或函数。在这种情况下,Python 解释器无法识别该变量或函数,并且会抛出 NameError。
在你提供的信息中,错误信息显示了名为 'input_data' 的变量未定义。可能的原因包括:
1. 拼写错误:可能是变量名的拼写错误,导致 Python 无法识别该变量。
2. 变量作用域:可能是变量在当前作用域中未定义,或者在其他函数或类中定义,而没有被正确引用。
3. 模块导入:可能是该变量在另一个模块中定义,但还没有被正确导入。
解决这个问题的方法是找出变量或函数的定义位置,并确保在使用它们之前进行正确的定义或导入。如果是拼写错误,则需要修正拼写错误。如果是变量作用域问题,则需要确认变量的作用域和引用方式是否正确。如果是模块导入问题,则需要正确导入相关模块。
阅读全文
相关推荐
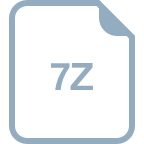
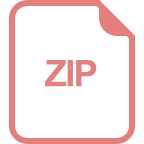
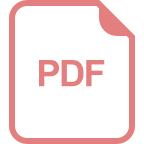
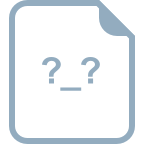
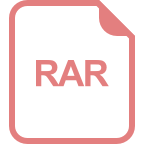
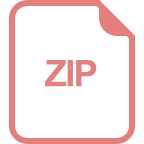
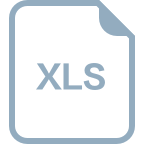
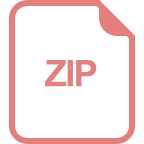
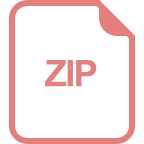
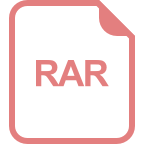
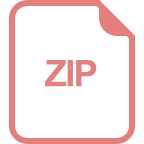
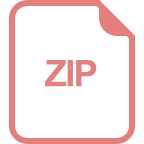
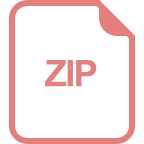
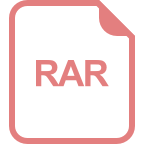