写一篇关于The similarities of affixation in English and Chinese的2000单词论文
时间: 2023-02-27 07:43:49 浏览: 139
尊敬的用户,很高兴为您回答。
首先,语言学中的词缀是一种形式,可以通过在词根或基础词上添加前缀或后缀来构建新词。英语和汉语都是两种大量使用词缀的语言。
在英语中,词缀通常用于改变词的词性,例如-ness表示名词性,而-ly表示副词性。此外,英语还使用词缀来表示某种语法关系,例如un-表示否定,dis-表示分离。
与英语相似,汉语中的词缀也是一种常用的语言形式,用于表示词性、语法关系等。汉语中的词缀通常是汉字,例如“的”可以用来表示名词性,而“地”可以用来表示副词性。此外,汉语还使用词缀来表示语气和语法关系,例如“不”表示否定,“得”表示方式。
因此,可以发现英语和汉语的词缀存在着很多相似的特点。例如,两种语言都使用词缀来改变词的词性,以及表示语气和语法关系等。
在总结上,英语和汉语的词缀存在着相似性,因为它们都是两种大量使用
相关问题
请以On the Criterion of Substantial Similarity of Artistic Works in American Law and the Controversy of Its Infringement Boundary为题用英语写一篇论文
On the Criterion of Substantial Similarity of Artistic Works in American Law and the Controversy of Its Infringement BoundaryAmerican law has long recognized that copyright protects creators from the unauthorized use of their artistic works. The law requires that there be a substantial similarity between the original work and any potential infringing work before the infringing work can be held liable for copyright infringement. In this paper, I will discuss the criteria used to determine substantial similarity in American law, the controversy surrounding its infringement boundary, and the implications of these issues for creators.To determine substantial similarity in American law, courts consider a variety of factors, including the purpose and character of the work, the similarity of the works, and the amount of copying that has taken place. The purpose and character of the work is generally determined by examining the source material, the original expression of ideas, and the similarities between the two works. The similarity of the works is assessed by considering the amount of copying that has occurred, the similarities in the subject matter, and the degree of similarity. Finally, the amount of copying is weighed by considering whether the work's substantial elements were copied, and how much of the work was copied.The controversy surrounding the substantial similarity of artistic works in American law is largely due to the fact that it is difficult to determine where to draw the line between legal and illegal copying. This is especially problematic for creators as it is often difficult to prove that their work has been copied by another artist. Additionally, the amount of copying that is considered to be infringing can vary from case to case, resulting in inconsistencies in the law.The implications of the substantial similarity of artistic works in American law are far-reaching. On one hand, it allows creators to protect their works by preventing others from using their ideas without permission. On the other hand, it can be used to stifle creativity by preventing new works from being created. Additionally, it can be used to prevent the dissemination of information, which can have a negative impact on the public's right to access knowledge and information.In conclusion, the substantial similarity of artistic works in American law is an important and complex issue. It is important for creators to be aware of the criteria used to determine substantial similarity and the controversy surrounding its infringement boundary. Additionally, they should be mindful of the implications of the substantial similarity of artistic works in American law and take steps to protect their works from potential infringement.
how can you classify titles by the similarities of titles via python
There are several ways to classify titles by their similarities using Python. Here are a few approaches:
1. Cosine Similarity:
Cosine similarity measures the similarity between two non-zero vectors by computing the cosine of the angle between them. In the case of text data, we can represent each title as a vector using techniques like TF-IDF or CountVectorizer. We can then compute the cosine similarity between all pairs of vectors and group the titles that have a high similarity score.
2. Word Embeddings:
Word embeddings are dense vector representations of words that capture their semantic meaning. We can use pre-trained word embeddings like Word2Vec or GloVe to represent each title as a vector. We can then compute the similarity between all pairs of vectors and group the titles that have a high similarity score.
3. Topic Modeling:
Topic modeling is a technique that identifies the underlying topics in a set of documents. We can apply topic modeling to the titles and group them based on the topics they belong to. We can use techniques like Latent Dirichlet Allocation (LDA) to identify the topics and assign each title to a topic.
4. Clustering:
Clustering is a technique that groups similar data points together. We can apply clustering algorithms like KMeans or Hierarchical Clustering to the titles and group them based on their similarity. We can use features like TF-IDF or word embeddings to represent each title as a vector and then apply the clustering algorithm to group the titles.
Overall, the approach we choose will depend on the nature of the data and the problem we are trying to solve.
阅读全文
相关推荐
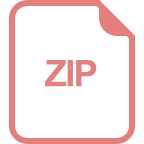
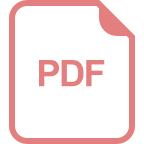
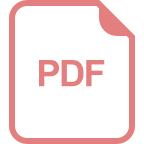













