$.ajax({ type: "POST", url: "post_computation_analogy", data: {"y":y,"x":x}, traditional: true, // async: false, success: function (rtn) { // alert("计算结果为:"+rtn); console.log(rtn) // data[0]=rtn[0] // data[1]=rtn[1] }, // result0=data[0]*input+data[1], });想要在Ajax之外访问rtn的返回,如何做
时间: 2024-01-27 21:05:23 浏览: 34
在Ajax之外访问Ajax返回的数据可以使用回调函数的方式。在Ajax请求中,可以将成功返回后的数据通过回调函数的形式传递给其他函数进行处理。例如:
```
function ajaxRequest(callback) {
$.ajax({
type: "POST",
url: "post_computation_analogy",
data: {"y":y,"x":x},
traditional: true,
success: function (rtn) {
callback(rtn);
}
});
}
// 调用ajaxRequest函数,并将回调函数作为参数传递
ajaxRequest(function(rtn) {
console.log(rtn); // 在回调函数中访问返回的数据
// 在这里对返回的数据进行处理
});
```
在这个例子中,`ajaxRequest`函数接收一个回调函数作为参数,并在Ajax请求成功后将返回的数据通过回调函数传递出去。调用`ajaxRequest`函数时,将需要执行的函数作为参数传递给它。这样,在Ajax请求成功时,就可以通过回调函数访问返回的数据并进行处理。
相关问题
calculate(){ const limit=this.tableData.length; let y=[]; let x=[]; for(let i=0;i<=limit-1;i++){ y.push(parseFloat(this.tableData[i].flux)); x.push(parseFloat(this.tableData[i].area)); } $.ajax({ type: "POST", url: "post_computation_analogy", data: {"y":y,"x":x}, traditional: true, // async: false, success: function (rtn) { let result0 = rtn[0] * parseFloat(this.input) + rtn[1]; flow.innerHTML="涌水量为"+result0+"涌水量单位" }, }); }这段代码中id为flow的<p>无法正常显示,是什么问题
这段代码中的 `flow` 对象没有被正确引用。可能的原因是在 `$.ajax()` 函数中,`this` 关键字已经不再指向原来的对象,而是指向了 `$.ajax()` 函数本身。解决这个问题的方法是将 `this` 关键字存储到另一个变量中,然后在 `$.ajax()` 函数中使用该变量。
你可以尝试这样修改代码:
```
calculate() {
const limit = this.tableData.length;
let y = [];
let x = [];
for (let i = 0; i <= limit - 1; i++) {
y.push(parseFloat(this.tableData[i].flux));
x.push(parseFloat(this.tableData[i].area));
}
const self = this; // 将 this 存储到 self 变量中
$.ajax({
type: "POST",
url: "post_computation_analogy",
data: { "y": y, "x": x },
traditional: true,
success: function (rtn) {
let result0 = rtn[0] * parseFloat(self.input) + rtn[1];
const flow = document.getElementById("flow"); // 获取 flow 元素
flow.innerHTML = "涌水量为 " + result0 + " 涌水量单位"; // 修改 flow 元素的内容
},
});
}
```
这样应该就可以正常显示 `flow` 元素了。
ModuleNotFoundError: No module named 'score_computation_cuda'
这个错误通常是由于缺少名为 'score_computation_cuda' 的模块导致的。你可以尝试以下几种方法解决这个问题:
1. 确保你已经安装了 'score_computation_cuda' 模块。你可以使用以下命令来安装它:
```
pip install score_computation_cuda
```
2. 如果你已经安装了 'score_computation_cuda' 模块,但仍然出现错误,可能是因为模块的路径没有正确添加到 Python 解释器的搜索路径中。你可以尝试手动将模块路径添加到系统路径中,例如:
```python
import sys
sys.path.append('/path/to/score_computation_cuda')
```
3. 如果你使用的是虚拟环境,请确保你在正确的环境中安装和导入模块。
4. 如果以上方法都不起作用,可能是由于模块没有正确安装或存在其他依赖关系问题。你可以查看 'score_computation_cuda' 模块的文档或寻求开发者的帮助来解决问题。
如果你提供更多的上下文和详细的错误信息,我可能能够给出更准确的建议。
相关推荐
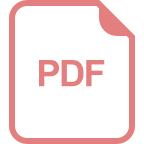
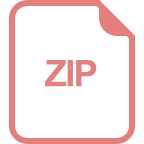
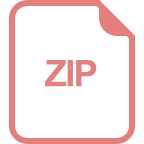









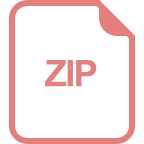
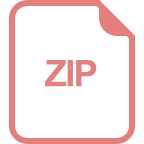