train_score.append(clf.score(x_train, y_train))
时间: 2024-03-07 08:51:44 浏览: 34
这也是一个Python代码行,它将分类器(clf)在训练数据集(x_train和y_train)上的得分(train_score)添加到一个列表中(append)。与前一个问题中的测试得分类似,这个得分通常用于评估模型在训练数据集上的表现,可以用来判断模型是否过拟合(overfitting)或者欠拟合(underfitting)。
相关问题
score = [] train_score = [] a = np.linspace(0.5, 2, 100) for C in a: x_train, x_test, y_train, y_test = train_test_split(result_x, y_0, test_size=0.3, random_state=0) # clf = SVC(C=C, kernel='rbf', gamma='auto', cache_size=5000).fit(x_train, y_train) score.append(clf.score(x_test, y_test)) train_score.append(clf.score(x_train, y_train)) print(a[score.index(max(score))])
这段代码使用了支持向量机(SVM)算法对数据进行分类,并通过网格搜索调整SVM算法中的超参数C的值,以使得算法在测试集上的分类准确率最高。具体来说,代码首先定义了一个等差数列a,用于存储不同的C值。然后,代码使用for循环遍历a中的每个C值,对每个C值都执行以下操作:
1. 使用train_test_split函数将数据集分为训练集和测试集,其中测试集占比为0.3。
2. 创建一个SVC实例clf,并使用fit函数对训练集进行训练。
3. 使用clf.score函数计算测试集和训练集上的分类准确率,并将其分别存储在score和train_score列表中。
在所有的C值上训练完成后,代码通过score.index(max(score))找到测试集上分类准确率最高的C值,然后将其输出。其中,max(score)表示测试集上最高的分类准确率,score.index(max(score))表示该分类准确率对应的C值在a中的索引。
我已经用数据训练好决策树和随机森林,现在我需要设置一组数据分别测试决策树和随机森林,预测这组数据描述的病人是否患有心脏病,请给我测试的代码。下面是我的训练过程import pandas as pd from sklearn import tree from sklearn.ensemble import RandomForestClassifier import matplotlib.pyplot as plt import numpy as np heart=pd.read_csv("D:\Anaconda1\heart.csv") heart.head() heart.info() clf_tree = tree.DecisionTreeClassifier() from sklearn.model_selection import train_test_split x = heart.drop('target',axis = 1) y = heart['target'] x_train,x_test,y_train,y_test = train_test_split(x,y,random_state=0) clf_tree.fit(x_train,y_train) print(clf_tree.score(x_train,y_train)) print(clf_tree.score(x_test,y_test)) tree.export_graphviz(clf, out_file='tree.dot') score_list=[] for i in range(10,100,10): clf_forest = RandomForestClassifier(n_estimators = i, random_state = 0) clf_forest.fit(x_train,y_train) score_list.append(clf_forest.score(x_test,y_test)) plt.plot(range(10,100,10), score_list) plt.show() print(np.argmax(score_list)) print(np.max(score_list))
好的,可以使用以下代码对决策树和随机森林进行测试:
```
# 导入测试数据
test_data = pd.read_csv("D:\Anaconda1\test_data.csv")
# 分离特征和标签
X_test = test_data.drop('target', axis=1)
y_test = test_data['target']
# 测试决策树
tree_score = clf_tree.score(X_test, y_test)
print("决策树的准确率为:", tree_score)
# 测试随机森林
forest_score = clf_forest.score(X_test, y_test)
print("随机森林的准确率为:", forest_score)
```
其中,`test_data` 是你准备用来测试的数据集, `X_test` 是测试数据的特征, `y_test` 是测试数据的标签。`tree_score` 和 `forest_score` 分别是决策树和随机森林的准确率。
你可以将这段代码添加到你的训练代码后面,就可以进行测试了。
相关推荐












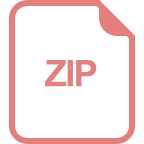