ValueError: Weights for model sequential have not yet been created. Weights are created when the Model is first called on inputs or `build()` is called with an `input_shape`.
时间: 2023-11-27 10:52:08 浏览: 464
这个错误通常是由于在模型调用之前没有设置模型的输入形状或者没有调用 `build()` 方法来创建模型权重。你需要确保在调用模型之前设置好模型的输入形状并调用 `build()` 方法创建模型权重。例如:
```python
import tensorflow as tf
model = tf.keras.Sequential([
tf.keras.layers.Dense(10, input_shape=(2,))
])
model.build(input_shape=(None, 2)) # 设置模型输入形状并创建模型权重
model.summary() # 打印模型结构
```
如果你使用的是函数式 API 或者子类化 API,你需要确保在调用 `Model` 类实例的 `__call__` 方法之前设置好模型的输入形状并创建模型权重。例如:
```python
import tensorflow as tf
inputs = tf.keras.Input(shape=(2,))
outputs = tf.keras.layers.Dense(10)(inputs)
model = tf.keras.Model(inputs=inputs, outputs=outputs)
model.summary() # 打印模型结构
```
如果你仍然遇到问题,请提供更多的代码和错误信息,以便我更好地帮助你。
相关问题
from tensorflow.keras import layers, Sequential mobile = tf.keras.applications.MobileNetV2(input_shape=(224, 224, 3), include_top=False, weights='imagenet') # Define your new input layer with the desired shape new_input = layers.Input(shape=(100, 125, 3)) # Create a new model by adding the new input layer to the old model new_model = Sequential([new_input] + mobile.layers[1:]) # Compile the new model new_model.compile(optimizer='adam', loss='categorical_crossentropy', metrics=['accuracy']) # Print the summary of the new model new_model.summary()这一段代码报错ValueError: A merge layer should be called on a list of inputs.
这个错误通常出现在使用 Keras 中的 merge 层时,它需要将多个张量作为输入,并将它们合并成一个张量。在这段代码中,可能是由于 MobileNetV2 模型中存在 merge 层,而将其合并到新模型中时出现了问题。
你可以尝试使用 Functional API 来构建模型,这样可以更灵活地处理模型的输入和输出。下面是一种修改方法,你可以试试看:
```
from tensorflow.keras import layers, models
# Define your new input layer with the desired shape
new_input = layers.Input(shape=(100, 125, 3))
# Load the MobileNetV2 model without the top layer
mobile = tf.keras.applications.MobileNetV2(input_shape=(224, 224, 3), include_top=False, weights='imagenet')
# Freeze the weights of the MobileNetV2 layers
for layer in mobile.layers:
layer.trainable = False
# Get the output of the MobileNetV2 model
x = mobile(new_input)
# Add a global average pooling layer and a dense layer for classification
x = layers.GlobalAveragePooling2D()(x)
x = layers.Dense(128, activation='relu')(x)
predictions = layers.Dense(10, activation='softmax')(x)
# Create the new model
new_model = models.Model(inputs=new_input, outputs=predictions)
# Compile the new model
new_model.compile(optimizer='adam', loss='categorical_crossentropy', metrics=['accuracy'])
# Print the summary of the new model
new_model.summary()
```
在这个修改后的代码中,我们首先定义了一个新的输入层 `new_input`,然后加载了 MobileNetV2 模型,并将其输出作为新模型的输入 `x`。在 `x` 上添加了一个全局平均池化层和一个密集层,最后是一个分类层 `predictions`。这个修改后的代码中没有使用 merge 层,因此不会出现该错误。
AttributeError: module 'tensorflow.keras.layers' has no attribute 'transformer'这个问题如何解决,添加transformer层
如果你想手动添加transformer层,可以参考以下代码实现:
```python
import tensorflow as tf
class MultiHeadSelfAttention(tf.keras.layers.Layer):
def __init__(self, embed_dim, num_heads, **kwargs):
super().__init__(**kwargs)
self.embed_dim = embed_dim
self.num_heads = num_heads
if embed_dim % num_heads != 0:
raise ValueError(
f"embedding dimension = {embed_dim} should be divisible by number of heads = {num_heads}"
)
self.projection_dim = embed_dim // num_heads
self.query_dense = tf.keras.layers.Dense(embed_dim)
self.key_dense = tf.keras.layers.Dense(embed_dim)
self.value_dense = tf.keras.layers.Dense(embed_dim)
self.combine_heads = tf.keras.layers.Dense(embed_dim)
def attention(self, query, key, value):
score = tf.matmul(query, key, transpose_b=True)
dim_key = tf.cast(tf.shape(key)[-1], tf.float32)
scaled_score = score / tf.math.sqrt(dim_key)
weights = tf.nn.softmax(scaled_score, axis=-1)
output = tf.matmul(weights, value)
return output, weights
def separate_heads(self, x, batch_size):
x = tf.reshape(x, (batch_size, -1, self.num_heads, self.projection_dim))
return tf.transpose(x, perm=[0, 2, 1, 3])
def call(self, inputs):
batch_size = tf.shape(inputs)[0]
query = self.query_dense(inputs)
key = self.key_dense(inputs)
value = self.value_dense(inputs)
query = self.separate_heads(query, batch_size)
key = self.separate_heads(key, batch_size)
value = self.separate_heads(value, batch_size)
attention, weights = self.attention(query, key, value)
attention = tf.transpose(attention, perm=[0, 2, 1, 3])
concat_attention = tf.reshape(attention, (batch_size, -1, self.embed_dim))
output = self.combine_heads(concat_attention)
return output
class TransformerBlock(tf.keras.layers.Layer):
def __init__(self, embed_dim, num_heads, ff_dim, rate=0.1, **kwargs):
super().__init__(**kwargs)
self.att = MultiHeadSelfAttention(embed_dim, num_heads)
self.ffn = tf.keras.Sequential(
[tf.keras.layers.Dense(ff_dim, activation="relu"), tf.keras.layers.Dense(embed_dim),]
)
self.layernorm1 = tf.keras.layers.LayerNormalization(epsilon=1e-6)
self.layernorm2 = tf.keras.layers.LayerNormalization(epsilon=1e-6)
self.dropout1 = tf.keras.layers.Dropout(rate)
self.dropout2 = tf.keras.layers.Dropout(rate)
def call(self, inputs, training):
attn_output = self.att(inputs)
attn_output = self.dropout1(attn_output, training=training)
out1 = self.layernorm1(inputs + attn_output)
ffn_output = self.ffn(out1)
ffn_output = self.dropout2(ffn_output, training=training)
return self.layernorm2(out1 + ffn_output)
class TokenAndPositionEmbedding(tf.keras.layers.Layer):
def __init__(self, maxlen, vocab_size, embed_dim, **kwargs):
super().__init__(**kwargs)
self.token_emb = tf.keras.layers.Embedding(input_dim=vocab_size, output_dim=embed_dim)
self.pos_emb = tf.keras.layers.Embedding(input_dim=maxlen, output_dim=embed_dim)
def call(self, x):
maxlen = tf.shape(x)[-1]
positions = tf.range(start=0, limit=maxlen, delta=1)
positions = self.pos_emb(positions)
x = self.token_emb(x)
return x + positions
class Transformer(tf.keras.Model):
def __init__(self, vocab_size, maxlen, embed_dim, num_heads, ff_dim, num_layers=4, **kwargs):
super().__init__(**kwargs)
self.embedding = TokenAndPositionEmbedding(maxlen, vocab_size, embed_dim)
self.transformer_blocks = [TransformerBlock(embed_dim, num_heads, ff_dim) for _ in range(num_layers)]
self.out = tf.keras.layers.Dense(vocab_size, activation="softmax")
def call(self, inputs, training):
x = self.embedding(inputs)
for block in self.transformer_blocks:
x = block(x, training)
x = self.out(x)
return x
```
这里实现了一个基本的Transformer模型,包括多头自注意力机制(MultiHeadSelfAttention)、Transformer块(TransformerBlock)、位置编码嵌入层(TokenAndPositionEmbedding)和Transformer模型(Transformer)。你可以根据需要调整其中的参数来满足你的需求。
阅读全文
相关推荐













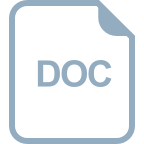


