np.linalg.svd参数
时间: 2023-11-02 10:57:53 浏览: 105
np.linalg.svd函数的参数如下:
```python
np.linalg.svd(a, full_matrices=True, compute_uv=True)
```
其中,参数说明如下:
- `a`:输入的矩阵,可以是二维或多维数组。
- `full_matrices`:一个布尔值,控制是否返回完整的奇异值分解结果。当为`True`时,返回完整的结果;当为`False`时,返回经过截断的结果,默认为`True`。
- `compute_uv`:一个布尔值,控制是否计算左右奇异向量。当为`True`时,返回奇异值、左奇异向量和右奇异向量;当为`False`时,只返回奇异值。默认为`True`。
注意:在对大规模矩阵进行奇异值分解时,选择`full_matrices=False`和`compute_uv=False`可以提高计算效率。
相关问题
np.linalg.svd
np.linalg.svd is a function provided by the NumPy library in Python. It stands for Singular Value Decomposition and is used for matrix factorization. SVD decomposes a matrix into three separate matrices, U, S, and V.
Here's an example of how to use np.linalg.svd:
```python
import numpy as np
# Create a matrix
A = np.array([[1, 2, 3], [4, 5, 6], [7, 8, 9]])
# Perform SVD
U, S, V = np.linalg.svd(A)
# U: Left singular vectors
# S: Singular values
# V: Right singular vectors
print("U:")
print(U)
print("S:")
print(S)
print("V:")
print(V)
```
The output will be the U, S, and V matrices. U contains the left singular vectors, S contains the singular values, and V contains the right singular vectors.
np.linalg.svd省略
np.linalg.svd 的省略形式是 np.svd。这是因为在使用 np.linalg 模块时,可以直接调用 svd 函数,而不需要使用 np.linalg.svd。
下面是使用 np.svd 进行奇异值分解的示例代码:
```python
import numpy as np
A = np.array([[1, 2], [3, 4], [5, 6]]) # 待分解的矩阵
U, S, VT = np.svd(A) # 进行奇异值分解
print("U:", U)
print("S:", S)
print("VT:", VT)
```
与之前的代码示例相比,这里直接调用了 np.svd 函数,其余部分保持不变。np.svd 函数与 np.linalg.svd 函数的功能完全相同,只是调用方式略有不同。
阅读全文
相关推荐
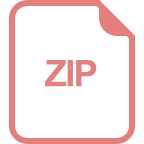
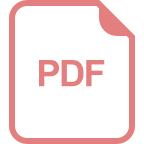
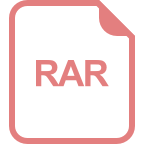












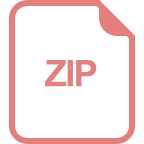
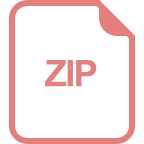