怎么理解std::vector<cv::Mat> inputs{matRGBScaled}
时间: 2024-01-21 17:03:48 浏览: 178
这段代码是使用C++中的标准库std::vector来创建一个向量(vector)对象,并初始化它的元素为一个cv::Mat对象。
具体地说,std::vector<cv::Mat> inputs表示创建了一个名为inputs的向量,其中的元素类型为cv::Mat,即OpenCV库中的图像矩阵类型。
初始化部分{matRGBScaled}表示向inputs中添加了一个元素,该元素的值为matRGBScaled。在这里,matRGBScaled是一个cv::Mat对象,可能是一个已经加载或创建的图像矩阵。
这样的初始化方式允许你在创建vector对象时直接指定初始元素,而不需要单独调用push_back()等函数添加元素。
需要注意的是,std::vector是一个动态数组,可以存储多个元素,并且具有自动调整大小的功能。因此,你可以在inputs中添加更多的cv::Mat对象,以便处理多个图像矩阵。
相关问题
怎么把这段c#转换为c++dll函数实现,并在最后把c返回给c# InferenceSession session = new InferenceSession(modelPath); Mat src_f = copy_from_mat(img); var wl = m_width * m_height; VectorOfMat temp = new VectorOfMat(); CvInvoke.Split(src_f, temp); float[] typedArr = new float[3 * m_width * m_height]; unsafe { fixed (float* target = typedArr) { for (int i = 0; i < temp.Size; i++) { var rawDataPointer = temp[i].DataPointer; Buffer.MemoryCopy((byte*)rawDataPointer, (byte*)target + (i * wl * sizeof(float)), wl * sizeof(float), wl * sizeof(float)); } } } var input = new DenseTensor<float>(typedArr, new[] { 1, 3, m_height, m_width }); var inputs = new List<NamedOnnxValue> { NamedOnnxValue.CreateFromTensor("images", input) }; var results = session.Run(inputs).ToArray(); //var ooo = results[0].AsTensor<float>(); float[] c = results[0].AsTensor<float>().ToArray();
以下是将该段代码转换为C++ DLL函数的实现,并在最后将C++返回给C#的示例代码:
首先,需要在C++中引入相关头文件和命名空间:
```c++
#include <vector>
#include <string>
#include <fstream>
#include <iostream>
#include <algorithm>
#include <numeric>
#include <chrono>
#include <memory>
#include <stdexcept>
#include <cstdlib>
#include <cstring>
#include <cassert>
#include <cmath>
#include <onnxruntime_cxx_api.h>
#include <opencv2/opencv.hpp>
using namespace std;
using namespace cv;
using namespace onnxruntime;
```
然后,实现C++ DLL函数:
```c++
// 将Mat对象复制到float数组
void copy_from_mat(Mat& img, float* target) {
vector<Mat> temp;
split(img, temp);
int wl = img.cols * img.rows;
for (int i = 0; i < temp.size(); i++) {
uchar* rawDataPointer = temp[i].data;
memcpy(target + i * wl, rawDataPointer, wl * sizeof(float));
}
}
// 将C++返回给C#的结果转换为float数组
void results_to_float_array(OrtValue& result, float* c) {
auto tensor = result.GetTensor<float>();
auto tensor_shape = tensor.Shape();
int num_elements = tensor_shape.Size();
memcpy(c, tensor.Data(), num_elements * sizeof(float));
}
// 实现C++ DLL函数
extern "C" __declspec(dllexport) int run_session(float* img_data, int img_width, int img_height, char* model_path, float* c) {
try {
// 初始化InferenceSession
SessionOptions session_options;
session_options.SetGraphOptimizationLevel(GraphOptimizationLevel::ORT_ENABLE_ALL);
session_options.SetExecutionMode(ExecutionMode::ORT_SEQUENTIAL);
session_options.SetIntraOpNumThreads(1);
session_options.SetInterOpNumThreads(1);
session_options.SetGraphOptimizationLevel(GraphOptimizationLevel::ORT_ENABLE_EXTENDED);
InferenceSession session(session_options);
OrtMemoryInfo info("Cpu", OrtDeviceAllocator, 0, OrtMemTypeCPU);
// 加载模型
session.LoadModel(model_path);
// 将输入数据复制到DenseTensor
float* typedArr = new float[3 * img_width * img_height];
copy_from_mat(img, typedArr);
vector<int64_t> dims = {1, 3, img_height, img_width};
auto input = OrtValue::CreateTensor<float>(info, typedArr, num_elements, dims.data(), dims.size());
delete[] typedArr;
// 执行推理
vector<OrtValue> ort_outputs = session.Run({ {session.GetInputName(0), input} });
// 将输出结果转换为float数组
results_to_float_array(ort_outputs[0], c);
return 0;
} catch (const exception& ex) {
cerr << ex.what() << endl;
return -1;
}
}
```
最后,需要在C#中声明C++ DLL函数,并调用该函数:
```c#
[DllImport("your_dll_name.dll")]
public static extern int run_session(float[] img_data, int img_width, int img_height, string model_path, float[] c);
// 调用C++ DLL函数
float[] img_data = ...; // 输入图像数据
int img_width = ...; // 输入图像宽度
int img_height = ...; // 输入图像高度
string model_path = ...; // 模型路径
float[] c = new float[...]; // 存储输出结果的数组
int ret = run_session(img_data, img_width, img_height, model_path, c);
if (ret == 0) {
// 输出结果处理代码
} else {
// 处理错误
}
```
阅读全文
相关推荐
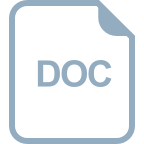
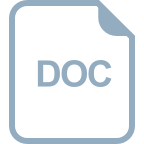
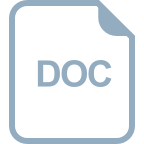
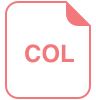
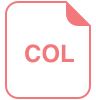
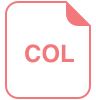
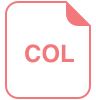
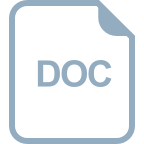
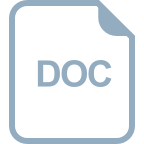
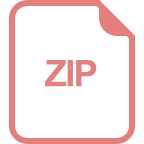
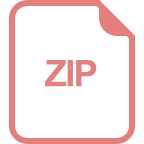
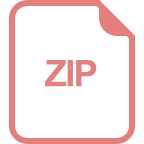
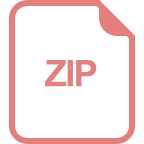
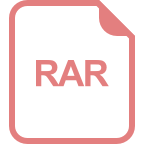
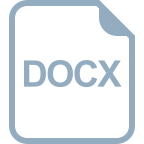
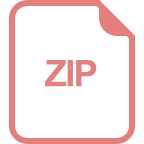
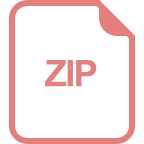