_map_to_pandas
时间: 2024-06-29 18:00:27 浏览: 167
`map_to_pandas`通常是指将非pandas数据结构(如字典、列表或其他迭代器)转换为pandas DataFrame的过程。这个过程可以帮助你更方便地进行数据分析和操作,因为pandas DataFrame提供了丰富的数据处理工具。
以下是一个简单的例子,演示如何使用`map_to_pandas`将字典列表转换为DataFrame:
```python
import pandas as pd
# 假设我们有如下字典列表
data = [
{'name': 'Alice', 'age': 25},
{'name': 'Bob', 'age': 30},
{'name': 'Charlie', 'age': 35}
]
# 使用 `pd.DataFrame.from_dict` 或 `pd.json_normalize` (如果数据是json格式)
df = pd.DataFrame(data)
# 输出:
# name age
# 0 Alice 25
# 1 Bob 30
# 2 Charlie 35
```
在这个例子中,`pd.DataFrame.from_dict`函数接收一个字典列表,创建了一个DataFrame,其中字典的键作为列名,值作为对应列的数据。
相关问题
df = pd.read_excel('BRA.xlsx') x = df['gauge_lon'].tolist() y = df['gauge_lat'].tolist() clusters = df['cluster'].tolist() # 读取Excel数据 brazil_map = Basemap(projection='cyl', llcrnrlon=-85, llcrnrlat=-35, urcrnrlon=-30, urcrnrlat=10, resolution='l') brazil_map.readshapefile("C:/Users/dellpc/Desktop/Paper/gadm36_BRA_shp/gadm36_BRA_1",'shape', drawbounds=True) brazil_map.drawmapboundary(fill_color='white') brazil_map.fillcontinents(color='white',lake_color='white') brazil_map.scatter(x,y,latlon=True,s=3,c=clusters,cmap='cool') brazil_map.colorbar() plt.savefig('brazil_map.png')优化这段代码
这段代码是用Python中的pandas库读取名为'BRA.xlsx'的Excel文件,并将其中的'gauge_lon'、'gauge_lat'和'cluster'三列数据分别转换为列表x、y和clusters。
pandas to_csv
可以使用pandas的to_csv()方法将DataFrame保存为csv文件。例如,使用以下代码将DataFrame保存为csv文件:
```python
import pandas as pd
df = pd.read_csv('./data/34/sample_pandas_normal.csv', index_col=0)
df.to_csv('./data/34/to_csv_out.csv')
```
这将把DataFrame保存为名为to_csv_out.csv的文件。如果你想将数据追加到现有的csv文件中,可以使用mode='a'参数。例如:
```python
df.to_csv('./data/34/to_csv_out.csv', mode='a', header=False)
```
这将把数据追加到to_csv_out.csv文件中,而不会添加新的列名。
如果你想指定整数或浮点数列的格式,可以先将DataFrame中的列转换为字符串格式,然后再保存为csv文件。例如:
```python
df\['col1'\] = df\['col1'\].map('{:.3f}'.format)
df\['col2'\] = df\['col2'\].map('{:.3e}'.format)
df\['col3'\] = df\['col3'\].map('{:#010x}'.format)
df.to_csv('./data/34/to_csv_out_float_format_str.csv')
```
这将把DataFrame中的col1列保留3位小数,col2列使用科学计数法表示,col3列以十六进制格式保存。
请注意,保存后的列类型将变为object。你可以使用df.dtypes来检查列的数据类型。
\[1\] \[2\] \[3\]
#### 引用[.reference_title]
- *1* *2* *3* [34_Pandas对CSV文件内容的导出和添加(to_csv)](https://blog.csdn.net/qq_18351157/article/details/113520345)[target="_blank" data-report-click={"spm":"1018.2226.3001.9630","extra":{"utm_source":"vip_chatgpt_common_search_pc_result","utm_medium":"distribute.pc_search_result.none-task-cask-2~all~insert_cask~default-1-null.142^v92^chatsearchT3_1"}} ] [.reference_item]
[ .reference_list ]
阅读全文
相关推荐
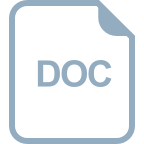
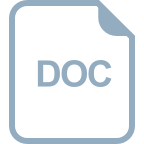
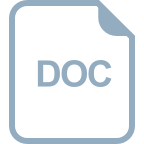
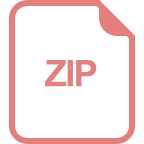
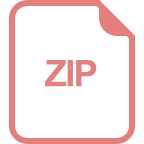
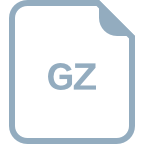
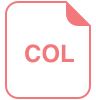
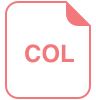




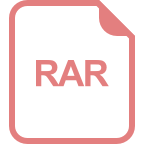




