img_noisy_torch = torch.from_numpy(img_resh_DN).view(1,p1,nr1,nc1).type(dtype)
时间: 2024-04-19 15:30:16 浏览: 35
这是一个将NumPy数组转换为Torch张量的代码行。它将一个名为`img_resh_DN`的NumPy数组转换为一个形状为(1, p1, nr1, nc1)的Torch张量,并将其类型设置为`dtype`。`dtype`是一个指定张量数据类型的变量,可能是`torch.float32`或`torch.float64`等。这个代码行通过使用`torch.from_numpy()`函数和`.view()`方法来完成转换和形状调整操作。
相关问题
转matlab: n_points_total = numpy.int(noisy_sensor_measured_total.shape[1]/(n_obs_in_sensor_array + 1)) intrinsic_process_total_reshaped = numpy.reshape(intrinsic_process_total, [dim_intrinsic, n_points_total, n_obs_in_sensor_array + 1], order='C') noisy_sensor_measured_total_reshaped = numpy.reshape(noisy_sensor_measured_total, [dim_measurement, n_points_total, n_obs_in_sensor_array + 1], order='C') intrinsic_process_base_total = intrinsic_process_total_reshaped[:, :, 0] intrinsic_process_step_total = intrinsic_process_total_reshaped[:, :, 1:] noisy_sensor_base_total = noisy_sensor_measured_total_reshaped[:, :, 0] n_obs_used_in_each_cluster = min(n_obs_used_in_cluster, n_obs_in_sensor_array) obs_used_in_each_cluster_indexes = numpy.random.choice(n_obs_in_sensor_array, size=n_obs_used_in_each_cluster, replace=False) sensor_array_matrix = sensor_array_matrix_dense[:, obs_used_in_each_cluster_indexes] noisy_sensor_step_total = noisy_sensor_measured_total_reshaped[:, :, 1:]
在Matlab中,您可以将以下Python代码转换为对应的Matlab代码:
```matlab
n_points_total = int(size(noisy_sensor_measured_total, 2) / (n_obs_in_sensor_array + 1));
intrinsic_process_total_reshaped = reshape(intrinsic_process_total, [dim_intrinsic, n_points_total, n_obs_in_sensor_array + 1]);
noisy_sensor_measured_total_reshaped = reshape(noisy_sensor_measured_total, [dim_measurement, n_points_total, n_obs_in_sensor_array + 1]);
intrinsic_process_base_total = intrinsic_process_total_reshaped(:, :, 1);
intrinsic_process_step_total = intrinsic_process_total_reshaped(:, :, 2:end);
noisy_sensor_base_total = noisy_sensor_measured_total_reshaped(:, :, 1);
n_obs_used_in_each_cluster = min(n_obs_used_in_cluster, n_obs_in_sensor_array);
obs_used_in_each_cluster_indexes = datasample(1:n_obs_in_sensor_array, n_obs_used_in_each_cluster, 'Replace', false);
sensor_array_matrix = sensor_array_matrix_dense(:, obs_used_in_each_cluster_indexes);
noisy_sensor_step_total = noisy_sensor_measured_total_reshaped(:, :, 2:end);
```
请注意,上述代码中的 `datasample` 函数用于在 `1:n_obs_in_sensor_array` 中随机选择 `n_obs_used_in_each_cluster` 个索引,并且不允许重复。如果您的Matlab版本不支持 `datasample` 函数,可以使用以下代码替代:
```matlab
obs_used_in_each_cluster_indexes = randsample(1:n_obs_in_sensor_array, n_obs_used_in_each_cluster, false);
```
这里使用了 `randsample` 函数来实现相同的功能。
import torch class Network(torch.nn.Module): def __init__(self, n_fft=1024, n_hop=160, n_hidden=1024): super().__init__() self.linear1 = torch.nn.LSTM(n_fft//2+1, n_hidden//2, num_layers=2, batch_first=True, bidirectional=True) self.linear2 = torch.nn.Linear(n_hidden, n_fft//2+1) self.n_fft = n_fft self.n_hop = n_hop # self.window = self.register_buffer('window', torch.hann_window(n_fft)) def forward(self, noisy): # 傅里叶变换 noisy_spec = torch.stft(noisy, self.n_fft, self.n_hop, window=self.window, return_complex=True) # [B,C,T] noisy_amplitude = torch.abs(noisy_spec).transpose(2,1) # [B,T,C=513] # 神经网络计算 hidden = self.linear1(noisy_amplitude)[0] # [B,T,n_hidden=1024] mask = self.linear2(hidden).sigmoid() # [B,T,C=513] (0,1) # 降噪结果 denoise_spec = noisy_spec * mask.transpose(2,1) denoise_wav = torch.istft(denoise_spec, self.n_fft, self.n_hop, window=self.window) # 返回降噪后波形和降噪后谱图 return denoise_wav, denoise_spec if __name__ == "__main__": net = Network() clean = torch.randn(4, 16000) noise = torch.randn(4, 16000) denoise_wav, denoise_spec = net(clean + noise) print(denoise_spec.shape, denoise_wav.shape)
这段代码实现了一个基于LSTM的音频降噪网络,其核心思路是使用LSTM对音频信号的幅度谱进行处理,从而得到一个掩膜(mask),该掩膜可以在频域上降噪信号。具体来说,该网络的输入是含有噪声的干净音频信号,经过傅里叶变换得到幅度谱,之后通过LSTM计算得到一个掩膜,最后将该掩膜应用于幅度谱上,得到降噪后的幅度谱,再通过逆傅里叶变换得到降噪后的音频信号。
具体来说,该网络包括以下几个部分:
- `__init__`函数:定义了一个两层的LSTM网络和一个线性层(用于将LSTM输出的隐藏状态转换为掩膜),并初始化了窗函数等参数。
- `forward`函数:实现了整个网络的前向传播过程。首先对输入信号进行傅里叶变换,得到幅度谱,之后将幅度谱通过LSTM处理得到一个掩膜,再将该掩膜应用于幅度谱上,得到降噪后的幅度谱,最后通过逆傅里叶变换得到降噪后的音频信号。
- `if __name__ == "__main__"`:对网络的功能进行测试,输入为一个随机产生的4秒钟的干净音频信号和一个随机产生的4秒钟的噪声信号,输出为降噪后的幅度谱和音频信号。
需要注意的是,由于该网络使用了LSTM,其计算成本较高,需要在计算资源允许的情况下使用。此外,该网络的性能也取决于数据集的质量和训练过程的参数设置等因素。
阅读全文
相关推荐

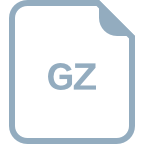














