tf.string_join函数未定义
时间: 2024-08-13 20:06:40 浏览: 96
`tf.string_join` 是 TensorFlow 中的一个函数,用于将多个字符串连接成一个字符串。然而,这个函数在早期版本的 TensorFlow (如 TensorFlow 1.x) 中是可用的,但在 TensorFlow 2.x 及以后版本中已经被弃用,取而代之的是 `tf.strings.join` 函数。
如果你在 TensorFlow 2.x 或更高版本中遇到 `tf.string_join` 未定义的问题,你应该使用 `tf.strings.join` 来进行字符串连接。`tf.strings.join` 接收一个字符串张量和一个分隔符作为参数,可以方便地处理字符串数组。
这里是 `tf.strings.join` 的基本用法:
```python
import tensorflow as tf
strings = tf.constant(["Hello", " ", "World"]) # 字符串张量
separator = tf.constant(",") # 分隔符
concatenated_string = tf.strings.join(strings, separator)
```
如果你是在 TensorFlow 1.x 版本中遇到这个问题,你需要查阅 TensorFlow 1.x 的文档,或者将代码升级到 TensorFlow 2.x。
阅读全文
相关推荐
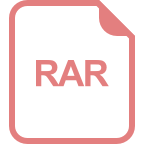
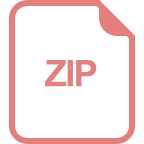
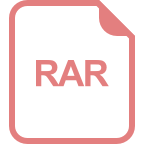
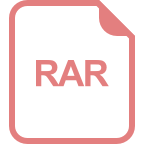
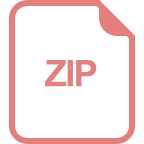
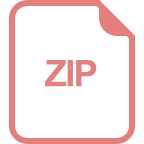
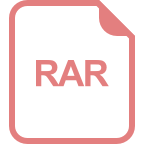
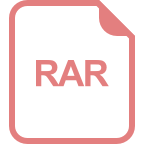
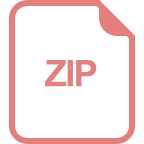
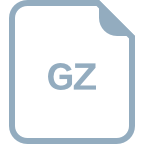
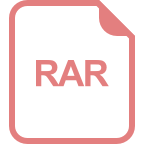
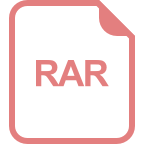
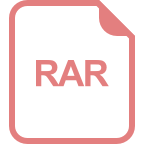
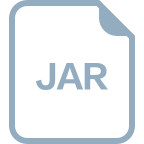
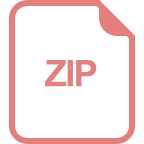
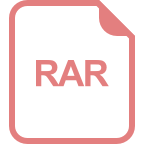
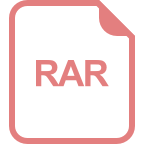
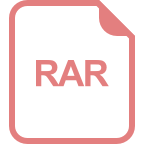
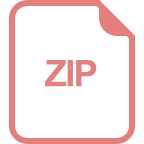