【Python pip Installation Tutorial for NumPy】: From Beginner to Expert, Step-by-Step Guide to Installing NumPy
发布时间: 2024-09-15 15:03:07 阅读量: 33 订阅数: 25 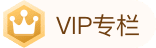
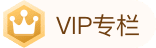
# 1. Introduction to Installing Numpy with Python pip
Python pip is a package management tool used in Python to manage and install packages. Numpy is a Python library used for scientific computing, providing powerful multi-dimensional array objects and advanced functions for manipulating these arrays. Installing Numpy using pip is the most common method for utilizing Numpy within Python.
In this section, we will introduce the basic principles and steps for installing Numpy with pip, including how to use the pip command-line tool and how to install Numpy on different operating systems. We will also discuss some common issues encountered during Numpy installation and their solutions.
# 2. Theoretical Foundation of Numpy Installation
### 2.1 Python Package Management Tool pip
The Python package management tool pip is used within the Python ecosystem for managing and installing Python packages. It allows users to easily install, update, and uninstall third-party Python packages, thus simplifying the Python development and deployment process.
pip works by interacting with the Python Package Index (PyPI) repository, which contains thousands of Python packages available for installation. Pip uses a file called requirements.txt to specify which packages and their version dependencies should be installed.
### 2.2 Principles of Numpy Installation
Numpy is a Python library for scientific computing that provides advanced functions for multi-dimensional arrays and matrix operations. The installation principles of Numpy are similar to other Python packages, utilizing pip to download and install the Numpy package from the PyPI repository.
The Numpy installation process involves the following steps:
1. Pip downloads the binary file or source code of the Numpy package from the PyPI repository.
2. Pip verifies the integrity and signature of the package.
3. Pip unzips the package and installs it into the Python environment.
4. Pip updates the Python path to include the installation directory of the Numpy package.
### 2.3 Different Ways to Install Numpy
There are several ways to install Numpy:
- **Using pip:** This is the simplest and most commonly used method. The command is as follows:
```
pip install numpy
```
- **From source code:** This method requires downloading the Numpy source code from the PyPI repository and manually compiling and installing it. The command is as follows:
```
python setup.py install
```
- **Using conda:** Conda is a package manager for managing Python packages and environments. It can install Numpy using the following command:
```
conda install numpy
```
- **Using Docker image:** Docker images can contain pre-installed Numpy and other dependencies. It can be installed using the following command:
```
docker run -it --rm python:3.8-slim-numpy bash
```
**Code Block 2.1: Installing Numpy with pip**
```python
import numpy as np
# Create a 3x3 array
arr = np.array([[1, 2, 3], [4, 5, 6], [7, 8, 9]])
# Print the array
print(arr)
```
**Logical Analysis:**
This code block demonstrates how to use pip to install Numpy and import it into a Python script. It creates a 3x3 array and prints it to the console.
**Argument Explanation:**
* `numpy`: The alias for the Numpy library.
* `np.array()`: The function used to create Numpy arrays.
* `arr`: The created Numpy array.
* `print()`: The function used to print the array.
# 3. Practical Guide to Numpy Installation
### 3.1 Installing Numpy with pip
Using pip to install Numpy is the simplest and most straightforward method, with the following steps:
1. **Open the Command Prompt or Terminal:** On Windows, press `Win + R` keys and enter `cmd`; on macOS or Linux, open the terminal.
2. **Enter the pip installation command:** Enter the following command and press the Enter key:
```
pip install numpy
```
3. **Wait for the installation to complete:** Pip will automatically download and install Numpy and its dependencies. The installation process may take a few minutes, depending on your network speed and computer performance.
4. **Verify the installation:** After installation, enter the following command to verify if Numpy has been successfully installed:
```
python -c "import numpy; print(numpy.__version__)"
```
### 3.2 Common Issues with Numpy Installation and Solutions
While installing Numpy, you may encounter the following common issues:
| **Issue** | **Solution** |
|---|---|
| **Dependency not satisfied** | Ensure that the Python development environment and pip are installed. If not, install Python and pip. |
| **Pip not found** | Ensure that pip has been added to the system path. On Windows, add `C:\Python\Scripts` to the `PATH` environment variable; on macOS or Linux, add `/usr/local/bin` to the `PATH` environment variable. |
| **Timeout error** | Check your network connection and ensure that the firewall is not blocking pip's access to the internet. |
| **Version conflict** | If different versions of Numpy are installed, uninstall the old version and reinstall. |
### 3.3 Verification and Uninstallation After Numpy Installation
**Verification:**
***Import Numpy:** In the Python interpreter or script, import the Numpy module:
```
import numpy as np
```
***Check the version:** Print the Numpy version number:
```
print(np.__version__)
```
**Uninstallation:**
***Using pip:** Enter the following command to uninstall Numpy:
```
pip uninstall numpy
```
***Manual uninstallation:** Delete the Numpy installation directory (usually located in the `site-packages` directory) and remove the Numpy executable from the `PATH` environment variable.
# 4. Advanced Applications of Numpy Installation
### 4.1 Automation and Scripting of Numpy Installation
Manual installation of Numpy may be feasible for small-scale projects, but for large projects or situations where Numpy needs to be installed on multiple machines, automation becomes crucial. Automation can be achieved through scripting, saving time and effort, and ensuring a consistent installation process.
#### 4.1.1 Installing Numpy with pip Script
pip provides a convenient API for writing scripts to automate the installation and management of packages. Below is an example of using a pip script to install Numpy:
```python
import pip
def install_numpy():
"""Install Numpy using pip"""
pip.main(['install', 'numpy'])
if __name__ == '__main__':
install_numpy()
```
This script can be saved as a file (e.g., `install_numpy.py`) and then run using the Python interpreter:
```bash
python install_numpy.py
```
This will automatically install Numpy.
#### 4.1.2 Installing Numpy with Ansible
Ansible is a popular automation tool used for configuring and managing IT infrastructure. It can script the installation of Numpy using YAML. Below is an example of using Ansible to install Numpy:
```yaml
- name: Install Numpy
pip:
name: numpy
```
This script can be saved as a file (e.g., `install_numpy.yml`) and then run using Ansible:
```bash
ansible-playbook install_numpy.yml
```
This will automatically install Numpy on the target machine.
### 4.2 Virtual Environment Management for Numpy Installation
Virtual environments allow for the installation and running of Python packages in isolated environments without affecting system-wide installations. This is particularly useful for testing different versions of Numpy or isolating Numpy from other packages.
#### 4.2.1 Creating a Virtual Environment with venv
Python provides an inbuilt `venv` module for creating virtual environments. Here is how to create a virtual environment using `venv`:
```bash
python -m venv venv_name
```
This will create a virtual environment named `venv_name` in the current directory.
#### 4.2.2 Installing Numpy in a Virtual Environment
To install Numpy into a virtual environment, use the following command:
```bash
source venv_name/bin/activate
pip install numpy
```
This will install Numpy into the virtual environment.
### 4.3 Version Management and Upgrades for Numpy Installation
Different versions of Numpy may come with different features and bug fixes. Managing Numpy versions is important for keeping the software up-to-date and avoiding compatibility issues.
#### 4.3.1 Managing Numpy Versions with pip
pip provides the `--upgrade` option to upgrade installed packages. Below is how to use pip to upgrade Numpy:
```bash
pip install --upgrade numpy
```
This will upgrade Numpy to the latest version.
#### 4.3.2 Managing Numpy Versions with conda
Conda is a popular Python package management tool that provides advanced version management features. Below is how to install a specific version of Numpy using conda:
```bash
conda install numpy=1.23.4
```
This will install Numpy version 1.23.4 in the conda environment.
# 5.1 Performance Optimization of Numpy Installation
In practical applications, performance optimization of Numpy installation is crucial, as it can significantly improve code execution efficiency. Here are some tips for optimizing Numpy installation performance:
- **Using binary installation packages:** Download precompiled binary installation packages from the official website to avoid the compilation process, thus saving a lot of time.
- **Using pip caching:** Pip caching can store downloaded installation packages, avoiding the need for repeated downloads, thereby speeding up the installation process. You can set the cache directory using `pip config set global.cache-dir <cache_directory>`.
- **Using parallel installation:** For large Numpy installations, you can enable parallel installation using the `pip install --process=n` option, where `n` is the number of processes to use.
- **Optimizing pip configuration:** By modifying the `pip.conf` file, you can optimize pip's behavior, such as increasing the download timeout or setting a proxy server.
- **Using virtual environments:** Installing Numpy in a virtual environment can isolate dependencies for different projects, prevent conflicts, and improve installation efficiency.
## 5.2 Security Considerations for Numpy Installation
Security considerations for Numpy installation should not be overlooked, as they involve system and data security. Here are some security precautions:
- **Download from official sources:** Only download Numpy installation packages from the official website or trusted repositories to avoid downloading malware or modified versions.
- **Check file integrity:** After downloading the Numpy installation package, use a checksum tool (like `sha256sum`) to check its integrity, ensuring the file has not been tampered with.
- **Use secure protocols:** Use secure protocols (such as HTTPS) when downloading and installing Numpy to prevent data leakage.
- **Limit installation permissions:** Only grant necessary user permissions to install Numpy to avoid unauthorized installations.
- **Regular updates:** Regularly update Numpy to fix security vulnerabilities and enhance performance.
## 5.3 Troubleshooting and Debugging for Numpy Installation
During the Numpy installation process, various issues may arise. Here are some troubleshooting and debugging tips:
- **Check dependencies:** Ensure that all dependencies required for Numpy, such as Python and NumPy, are installed.
- **View error logs:** Carefully examine the error logs in pip or installation scripts to identify the root cause of the problem.
- **Use the --verbose option:** Add the `--verbose` option to the installation command to get more detailed installation information.
- **Use debug mode:** Add the `--debug` option to the pip command to enable debug mode and get more in-depth error information.
- **Seek community support:** Ask for help on Stack Overflow or other online forums, discussing problems with other users and developers.
0
0
相关推荐
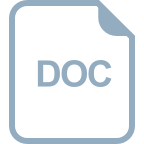
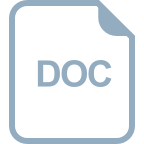
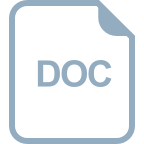
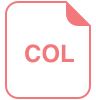
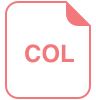
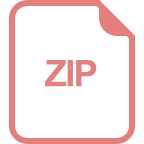
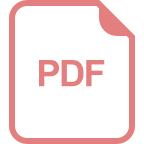
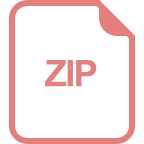