C Language Pixel Data Loading and Analysis [Image Reading] BMP Image Loading
发布时间: 2024-09-14 19:01:22 阅读量: 20 订阅数: 16 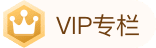
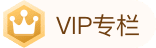
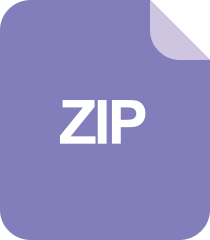
CameraFirmware_Clanguage_imageprocessing_USBprogramming_Camerafi
# 1. Introduction
1.1 What is the BMP Image Format
1.2 The Importance of Image Processing in C Language
1.3 Purpose and Structure Overview of This Article
In the realm of image processing and computer vision, BMP (Bitmap) is a common lossless image file format known for its straightforward storage structure and direct access to pixel data. As a low-level language, C plays a crucial role in image processing, with its direct and efficient characteristics making it the preferred choice for image processing algorithms and application development. This article will introduce the basics of the BMP image format, explore the importance of C language in image processing, and provide an overview of the purpose and structure of this article.
# 2. Parsing the BMP Image File Format
The BMP (Bitmap) image file is a common lossless image file format that plays a significant role in image processing. Understanding the structure of BMP image files can help us better grasp how image data is stored and processed. This section will dissect the BMP image file format, including an overview of the file structure, a detailed analysis of the file header, and an introduction to the storage of pixel data. Let's delve into the details of the BMP image file format together.
# 3. Implementing BMP Image Reading in C Language
In this chapter, we will discuss in detail how to use C language to implement BMP image reading. By following these steps, we can successfully read BMP image files and process their pixel data.
#### 3.1 Opening BMP Image Files and Reading File Header Information
First, we need to open the BMP image file and read the file header information for further parsing of the pixel data. Below is a simple example code:
```c
#include <stdio.h>
#include <stdint.h>
#pragma pack(push, 1) // Disable alignment
typedef struct {
uint16_t type; // File type
uint32_t size; // File size
uint16_t reserved1; // Reserved field
uint16_t reserved2; // Reserved field
uint32_t offset; // Data offset
} BMPHeader;
#pragma pack(pop)
int main() {
FILE* file = fopen("sample.bmp", "rb");
if (file == NULL) {
printf("Error opening file.\n");
return 1;
}
BMPHeader header;
fread(&header, sizeof(BMPHeader), 1, file);
// Read and print file header information
printf("File type: %c%c\n", header.type & 0xff, header.type >> 8);
printf("File size: %d bytes\n", header.size);
printf("Data offset: %d bytes\n", header.offset);
fclose(file);
return 0;
}
```
With this code, we can open a BMP image file, read the file header information, and output various parameters such as file type, file size, and data offset.
#### 3.2 Reading Pixel Data from a BMP Image File
Next, we will discuss how to read the pixel data from a BMP image file, which is one of the most critical steps in image processing. Below is a simple example code:
```c
#include <stdio.h>
#include <stdint.h>
typedef struct {
uint8_t blue;
uint8_t green;
uint8_t red;
} Pixel;
int main() {
// Assume the BMP file header and offset have already been read
FILE* file = fopen("sample.bmp", "rb");
if (file == NULL) {
printf("Error opening file.\n");
return 1;
}
fseek(file, header.offset, SEEK_SET);
Pixel pixel;
while (fread(&pixel, sizeof(Pixel), 1, file)) {
// Process pixel data, operations such as brightness analysis, filter processing, etc., can be performed
}
fclose(file);
return 0;
}
```
In this code, we use a struct `Pixel` to represent the color information of each pixel and read pixel data one by one through a loop for subsequent image processing operations.
#### 3.3 Memory Management and Pixel Data Parsing
In actual image processing, we may need to perform further operations and parsing on pixel data, which requires careful memory management and pixel data format analysis. When processing pixel data, pay close attention to memory allocation and deallocation to avoid issues such as memory leaks.
Through the above steps, we can implement the reading of BMP image files and successfully obtain pixel data for further processing. Next, in the following chapter, we will discuss how to process and analyze image pixel data.
# 4. Image Pixel Data Processing and Analysis
Image processing is not just about reading image data; more importantly, it's about processing and analyzing the image data. In this chapter, we will delve into the structure of image pixel data, brightness adjustment, and feature analysis.
#### 4.1 Image Pixel Data Structure Analysis
In image processing, understanding the structure of image pixel data is crucial. Each pixel usually consists of color values from three channels: RGB. During processing, factors such as the range of pixel values and the arrangement must be considered. In-depth analysis of the image pixel data structure can better implement various image processing algorithms.
```python
# Code example: Retrieve image pixel data and print pixel value range
import numpy as np
import cv2
# Read image
image = cv2.imread('image.bmp')
# Get pixel value range
min_value = np.min(image)
max_value = np.max(image)
print(f"Min pixel value: {min_value}, Max pixel value: {max_value}")
```
**Code Summary:** With the above code example, we can obtain the pixel value range of the image, which aids in subsequent brightness adjustment and feature analysis.
**Result Explanation:** The printed minimum and maximum pixel values can help us understand the range of image pixel data and provide a reference for subsequent processing.
#### 4.2 Image Brightness Analysis and Adjustment
Image brightness ***mon brightness adjustment methods in image processing include linear transformation, histogram equalization, etc. Below we take histogram equalization as an example to analyze and adjust image brightness.
```python
# Code example: Perform histogram equalization on the image
import cv2
# Read image
image = cv2.imread('image.bmp', cv2.IMREAD_GRAYSCALE)
# Perform histogram equalization
equalized_image = cv2.equalizeHist(image)
# Display the original and processed images
cv2.imshow('Original Image', image)
cv2.imshow('Equalized Image', equalized_image)
cv2.waitKey(0)
cv2.destroyAllWindows()
```
**Code Summary:** Through the process of histogram equalization, the brightness distribution of the image can be effectively adjusted, enhancing the visual quality of the image.
**Result Explanation:** By comparing the original and histogram-equalized images, we can observe the effect of brightness equalization on the visual effect of the image.
#### 4.3 Feature Analysis of Image Data
Image data has rich features, including color distribution, texture features, shape features, etc. Feature analysis of image data can help us understand the content and structure of the image, providing a basis for subsequent tasks such as image classification and detection.
```python
# Code example: Extract color histogram features from the image
import cv2
import matplotlib.pyplot as plt
# Read image
image = cv2.imread('image.bmp')
image = cv2.cvtColor(image, cv2.COLOR_BGR2RGB)
# Calculate color histogram
histogram = cv2.calcHist([image], [0, 1, 2], None, [256, 256, 256], [0, 256, 0, 256, 0, 256])
# Visualize color histogram
fig = plt.figure()
ax = fig.add_subplot(111, projection='3d')
X, Y, Z = np.meshgrid(range(256), range(256), range(256))
ax.scatter(X, Y, Z, c=histogram.flatten())
plt.show()
```
**Code Summary:** Through the extraction and visualization of color histogram features, we can intuitively understand the color distribution features of the image.
**Result Explanation:** Through the visualization of the color histogram, we can analyze the features of the image data from the perspective of color distribution, laying the foundation for subsequent image analysis.
# 5. Image Processing Application Examples
Image processing is a very important aspect of the computer vision field. By processing and analyzing images, various functions and applications can be realized. The following will introduce some common image processing application examples, including image resizing, image filter application, and image quality assessment.
#### 5.1 Image Resizing
Image resizing is one of the common operations in image processing, which can change the size of an image by adjusting its pixel dimensions. This has extensive applications in image display, printing, storage, and more. The following is an example Python code demonstrating how to resize an image using the PIL library:
```python
from PIL import Image
# Open image file
img = Image.open('input.jpg')
# Resize image to 200x200 pixels
resized_img = img.resize((200, 200))
# Save the resized image
resized_img.save('output.jpg')
```
With this code, we can resize the image named `input.jpg` to 200x200 pixels and save it as `output.jpg`.
#### 5.2 Image Filter Application
Image filters can add various special effects to images, such as blurring, sharpening, edge detection, etc., for beautifying images or enhancing image features. Below is an example Python code using the OpenCV library to achieve a blurring effect:
```python
import cv2
# Read image file
img = cv2.imread('input.jpg')
# Apply Gaussian blur
blurred_img = cv2.GaussianBlur(img, (15, 15), 0)
# Save the processed image
cv2.imwrite('output.jpg', blurred_img)
```
This code will apply Gaussian blur to the image named `input.jpg` and save it as `output.jpg`.
#### 5.3 Image Quality Assessment
Image quality assessment is a very important aspect of the image processing field, used to evaluate various aspects of an image, such as clarity, contrast, and color. The following is an example Python code using the OpenCV library to calculate image clarity:
```python
import cv2
# Read image file
img = cv2.imread('input.jpg')
# Calculate image clarity
blur = cv2.Laplacian(img, cv2.CV_64F).var()
print(f'Image clarity is: {blur}')
```
With this code, we can calculate the clarity of the image named `input.jpg` and output the result.
These are the introductions to image processing application examples. These functions are frequently used in actual development and can help us better process and analyze image data.
# 6. Conclusion and Outlook
In this article, we have detailed how to read and process BMP image pixel data in C language. Through parsing the BMP image format, we have gained an in-depth understanding of the structure and storage method of BMP image files. In the section on implementing BMP image reading in C language, we have shown how to open files, read file header information, and obtain pixel data, and we have discussed memory management and data parsing.
In the part on image pixel data processing and analysis, we have explored the importance of analyzing the structure and features of image pixel data, and we have introduced methods for adjusting image brightness. Finally, we have provided several image processing application examples, including image resizing, image filter application, and image quality assessment.
#### 6.1 Summary of This Article
After reading this article, the reader should have mastered the method of reading BMP image pixel data in C language, understood the basic processes and operational steps of image processing. With this knowledge, the reader can further expand the applications in the image processing field and realize more interesting functions and effects.
#### 6.2 Future Development Direction of Image Processing
With the development of artificial intelligence and deep learning technologies, the image processing field will also welcome more innovations and breakthroughs. In the future, image processing technology will become more intelligent and automated, such as image recognition, object detection, image generation, and other aspects will be further developed and applied.
#### 6.3 Conclusion
As an important part of the computer vision field, image processing has brought us many conveniences and pleasures. We hope this article has been helpful in the reader's study and work in the field of image processing and look forward to readers continuously exploring and innovating in practice, contributing their own strength to the development of image processing technology.
0
0