【Matplotlib子图绘制秘籍】:揭秘专业子图绘制技巧
发布时间: 2024-07-12 08:09:02 阅读量: 36 订阅数: 42 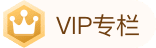
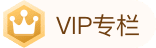
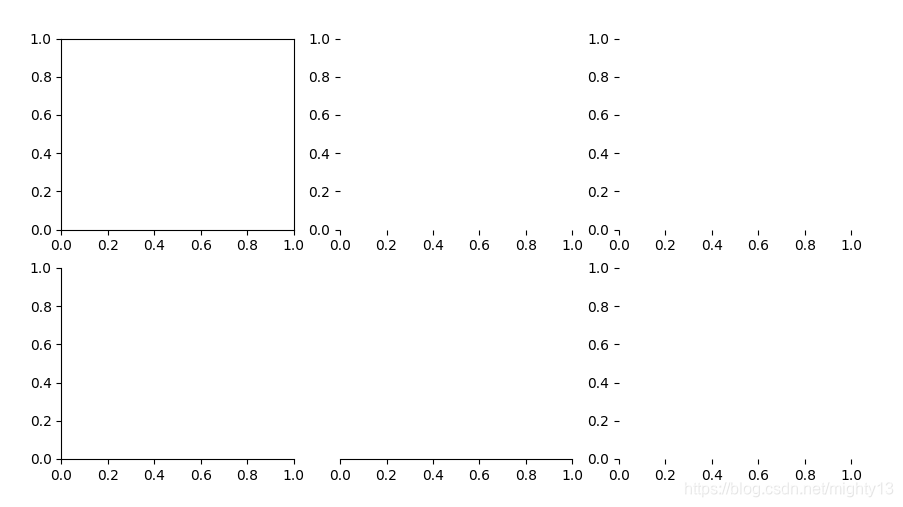
# 1. Matplotlib子图概述**
Matplotlib子图是一种强大的工具,它允许您在单个图形中绘制多个子图。子图可以用来显示不同的数据透视图,比较不同的数据集,或创建交互式可视化。
子图的创建和管理非常简单。您可以使用`plt.subplot()`函数创建子图,并使用`plt.subplots()`函数创建多个子图。子图的布局和调整可以通过`plt.subplots_adjust()`函数进行控制。
# 2. Matplotlib子图绘制基础
### 2.1 子图的创建和管理
#### 2.1.1 子图的创建方法
Matplotlib提供了多种创建子图的方法:
- `subplot()`:创建单个子图,指定行数、列数和子图位置。
- `subplots()`:创建多个子图,返回一个包含子图对象的元组。
- `add_subplot()`:在现有图形中添加子图。
```python
# 使用 subplot() 创建单个子图
import matplotlib.pyplot as plt
fig, ax = plt.subplots()
ax.plot([1, 2, 3], [4, 5, 6])
plt.show()
# 使用 subplots() 创建多个子图
fig, axs = plt.subplots(2, 2)
axs[0, 0].plot([1, 2, 3], [4, 5, 6])
axs[0, 1].plot([7, 8, 9], [10, 11, 12])
axs[1, 0].plot([13, 14, 15], [16, 17, 18])
axs[1, 1].plot([19, 20, 21], [22, 23, 24])
plt.show()
```
#### 2.1.2 子图的布局和调整
子图的布局和调整可以通过以下方法进行:
- `set_position()`:设置子图在图形中的位置。
- `set_aspect()`:设置子图的宽高比。
- `tight_layout()`:自动调整子图之间的间距。
```python
# 设置子图位置
fig, ax = plt.subplots()
ax.set_position([0.2, 0.2, 0.6, 0.6])
plt.show()
# 设置子图宽高比
fig, ax = plt.subplots()
ax.set_aspect(2)
plt.show()
# 自动调整子图间距
fig, axs = plt.subplots(2, 2)
axs[0, 0].plot([1, 2, 3], [4, 5, 6])
axs[0, 1].plot([7, 8, 9], [10, 11, 12])
axs[1, 0].plot([13, 14, 15], [16, 17, 18])
axs[1, 1].plot([19, 20, 21], [22, 23, 24])
plt.tight_layout()
plt.show()
```
### 2.2 子图的属性设置
#### 2.2.1 标题、标签和刻度的设置
子图的标题、标签和刻度可以通过以下方法进行设置:
- `set_title()`:设置子图标题。
- `set_xlabel()`、`set_ylabel()`:设置 x 轴和 y 轴标签。
- `set_xticks()`、`set_yticks()`:设置 x 轴和 y 轴刻度。
```python
# 设置子图标题
fig, ax = plt.subplots()
ax.set_title("Matplotlib Subplot Example")
plt.show()
# 设置轴标签
fig, ax = plt.subplots()
ax.set_xlabel("X-axis")
ax.set_ylabel("Y-axis")
plt.show()
# 设置刻度
fig, ax = plt.subplots()
ax.set_xticks([1, 2, 3, 4, 5])
ax.set_yticks([10, 20, 30, 40, 50])
plt.show()
```
#### 2.2.2 图形区域的设置
图形区域的设置可以通过以下方法进行:
- `set_xlim()`、`set_ylim()`:设置 x 轴和 y 轴的范围。
- `set_facecolor()`:设置图形区域的背景颜色。
- `grid()`:显示网格线。
```python
# 设置轴范围
fig, ax = plt.subplots()
ax.set_xlim(0, 10)
ax.set_ylim(0, 100)
plt.show()
# 设置背景颜色
fig, ax = plt.subplots()
ax.set_facecolor("lightblue")
plt.show()
# 显示网格线
fig, ax = plt.subplots()
ax.grid()
plt.show()
```
#### 2.2.3 图例的设置
图例可以通过以下方法进行设置:
- `legend()`:创建图例。
- `set_title()`:设置图例标题。
- `set_loc()`:设置图例位置。
```python
# 创建图例
fig, ax = plt.subplots()
ax.plot([1, 2, 3], [4, 5, 6], label="Line 1")
ax.plot([7, 8, 9], [10, 11, 12], label="Line 2")
ax.legend()
plt.show()
# 设置图例标题
fig, ax = plt.subplots()
ax.plot([1, 2, 3], [4, 5, 6], label="Line 1")
ax.plot([7, 8, 9], [10, 11, 12], label="Line 2")
ax.legend(title="Legend
# 3. Matplotlib子图高级绘制技巧
### 3.1 多轴子图
#### 3.1.1 创建和管理多轴子图
多轴子图允许在一个绘图区域中绘制多个子图,每个子图具有自己的坐标轴和数据。要创建多轴子图,可以使用`matplotlib.pyplot.subplot2grid`函数。该函数需要三个参数:
- `shape`:一个元组,指定子图的网格形状,例如`(2, 2)`表示一个2行2列的网格。
- `location`:一个整数,指定子图在网格中的位置,从左上角开始从1开始编号。
- `rowspan`和`colspan`:可选参数,指定子图跨越的行数和列数。
```python
import matplotlib.pyplot as plt
# 创建一个2行2列的多轴子图
fig, axes = plt.subplots(2, 2)
# 在第一个子图中绘制折线图
axes[0, 0].plot([1, 2, 3], [4, 5, 6])
# 在第二个子图中绘制柱状图
axes[0, 1].bar([1, 2, 3], [4, 5, 6])
# 在第三个子图中绘制散点图
axes[1, 0].scatter([1, 2, 3], [4, 5, 6])
# 在第四个子图中绘制饼图
axes[1, 1].pie([1, 2, 3])
plt.show()
```
#### 3.1.2 共享轴和独立轴
在多轴子图中,可以共享轴或设置独立轴。默认情况下,所有子图共享相同的x轴和y轴。要共享轴,可以使用`sharex`和`sharey`参数。
```python
# 创建一个2行2列的多轴子图,共享x轴和y轴
fig, axes = plt.subplots(2, 2, sharex=True, sharey=True)
```
要设置独立轴,可以使用`independent_axes`参数。
```python
# 创建一个2行2列的多轴子图,具有独立轴
fig, axes = plt.subplots(2, 2, independent_axes=True)
```
### 3.2 极坐标子图
#### 3.2.1 极坐标子图的创建
极坐标子图用于绘制极坐标数据。要创建极坐标子图,可以使用`matplotlib.pyplot.polar`函数。
```python
import matplotlib.pyplot as plt
# 创建一个极坐标子图
fig, ax = plt.subplots(subplot_kw={'projection': 'polar'})
# 绘制极坐标数据
ax.plot([0, np.pi/2, np.pi, 3*np.pi/2, 2*np.pi], [1, 2, 3, 4, 5])
plt.show()
```
#### 3.2.2 极坐标数据的绘制
在极坐标子图中,可以使用`plot`函数绘制极坐标数据。`plot`函数需要两个参数:
- `r`:径向坐标值。
- `theta`:角度坐标值。
```python
# 绘制一个极坐标散点图
ax.scatter(theta, r)
```
### 3.3 3D子图
#### 3.3.1 3D子图的创建
3D子图用于绘制三维数据。要创建3D子图,可以使用`matplotlib.pyplot.figure`函数并指定`projection`参数为`'3d'`。
```python
import matplotlib.pyplot as plt
from mpl_toolkits.mplot3d import Axes3D
# 创建一个3D子图
fig = plt.figure()
ax = fig.add_subplot(111, projection='3d')
```
#### 3.3.2 3D数据的绘制
在3D子图中,可以使用`plot3D`函数绘制3D数据。`plot3D`函数需要三个参数:
- `x`:x坐标值。
- `y`:y坐标值。
- `z`:z坐标值。
```python
# 绘制一个3D线框图
ax.plot3D(x, y, z)
```
# 4. Matplotlib子图交互操作
### 4.1 子图的交互式操作
Matplotlib提供了交互式操作子图的功能,允许用户与图形进行交互,以进行探索和分析。
#### 4.1.1 缩放和平移
* **缩放:**
* 使用鼠标滚轮或按住`Ctrl`键并拖动鼠标进行缩放。
* `matplotlib.pyplot.zoom()`函数可用于编程缩放。
* **平移:**
* 按住`Alt`键并拖动鼠标进行平移。
* `matplotlib.pyplot.pan()`函数可用于编程平移。
#### 4.1.2 数据点的选择和操作
* **数据点选择:**
* 使用鼠标单击或按住`Shift`键并拖动鼠标选择数据点。
* `matplotlib.pyplot.ginput()`函数可用于编程选择数据点。
* **数据点操作:**
* 选择数据点后,可以通过以下方式进行操作:
* 移动:按住鼠标并拖动。
* 删除:按`Delete`键。
* 编辑:双击数据点打开编辑对话框。
### 4.2 子图的保存和导出
#### 4.2.1 图像格式的选择
Matplotlib支持多种图像格式,包括:
| 格式 | 描述 |
|---|---|
| PNG | 便携式网络图形,适用于网络和屏幕显示。 |
| JPEG | 联合图像专家组,适用于照片和图像。 |
| SVG | 可缩放矢量图形,适用于可缩放和编辑的图形。 |
| PDF | 便携式文档格式,适用于高质量打印和文档。 |
#### 4.2.2 图像的保存和导出
* **保存图像:**
* `matplotlib.pyplot.savefig()`函数可用于将图像保存到文件中。
* 参数:
* `filename`:保存的文件名。
* `format`:图像格式(例如`'png'`、`'jpeg'`)。
* **导出图像:**
* `matplotlib.pyplot.show()`函数可用于显示交互式图形。
* `matplotlib.pyplot.close()`函数可用于关闭交互式图形。
* `matplotlib.pyplot.figure`对象还提供了`savefig()`和`show()`方法。
```python
# 保存图像为 PNG 格式
import matplotlib.pyplot as plt
plt.savefig('my_plot.png', format='png')
# 显示交互式图形
plt.show()
# 关闭交互式图形
plt.close()
```
# 5. Matplotlib子图实战应用
### 5.1 数据可视化
#### 5.1.1 折线图、柱状图和散点图
折线图、柱状图和散点图是数据可视化的基本类型。Matplotlib提供了丰富的API来绘制这些图表。
```python
import matplotlib.pyplot as plt
# 折线图
plt.plot([1, 2, 3, 4], [5, 6, 7, 8])
plt.xlabel("X轴")
plt.ylabel("Y轴")
plt.title("折线图")
plt.show()
# 柱状图
plt.bar([1, 2, 3, 4], [5, 6, 7, 8])
plt.xlabel("X轴")
plt.ylabel("Y轴")
plt.title("柱状图")
plt.show()
# 散点图
plt.scatter([1, 2, 3, 4], [5, 6, 7, 8])
plt.xlabel("X轴")
plt.ylabel("Y轴")
plt.title("散点图")
plt.show()
```
#### 5.1.2 饼图和雷达图
饼图和雷达图可以用来表示比例数据或多维数据。
```python
# 饼图
plt.pie([10, 20, 30, 40], labels=["A", "B", "C", "D"])
plt.title("饼图")
plt.show()
# 雷达图
plt.radar([10, 20, 30, 40], labels=["A", "B", "C", "D"])
plt.title("雷达图")
plt.show()
```
### 5.2 图像处理
#### 5.2.1 图像的读取和显示
Matplotlib可以读取和显示图像文件。
```python
from matplotlib import image
# 读取图像
img = image.imread("image.png")
# 显示图像
plt.imshow(img)
plt.title("图像")
plt.show()
```
#### 5.2.2 图像的处理和增强
Matplotlib提供了丰富的图像处理和增强功能。
```python
# 灰度化
img_gray = image.rgb2gray(img)
# 锐化
img_sharp = image.sharpen(img)
# 旋转
img_rotate = image.rotate(img, 45)
# 显示处理后的图像
plt.subplot(131)
plt.imshow(img_gray, cmap="gray")
plt.title("灰度化")
plt.subplot(132)
plt.imshow(img_sharp)
plt.title("锐化")
plt.subplot(133)
plt.imshow(img_rotate)
plt.title("旋转")
plt.show()
```
0
0
相关推荐





