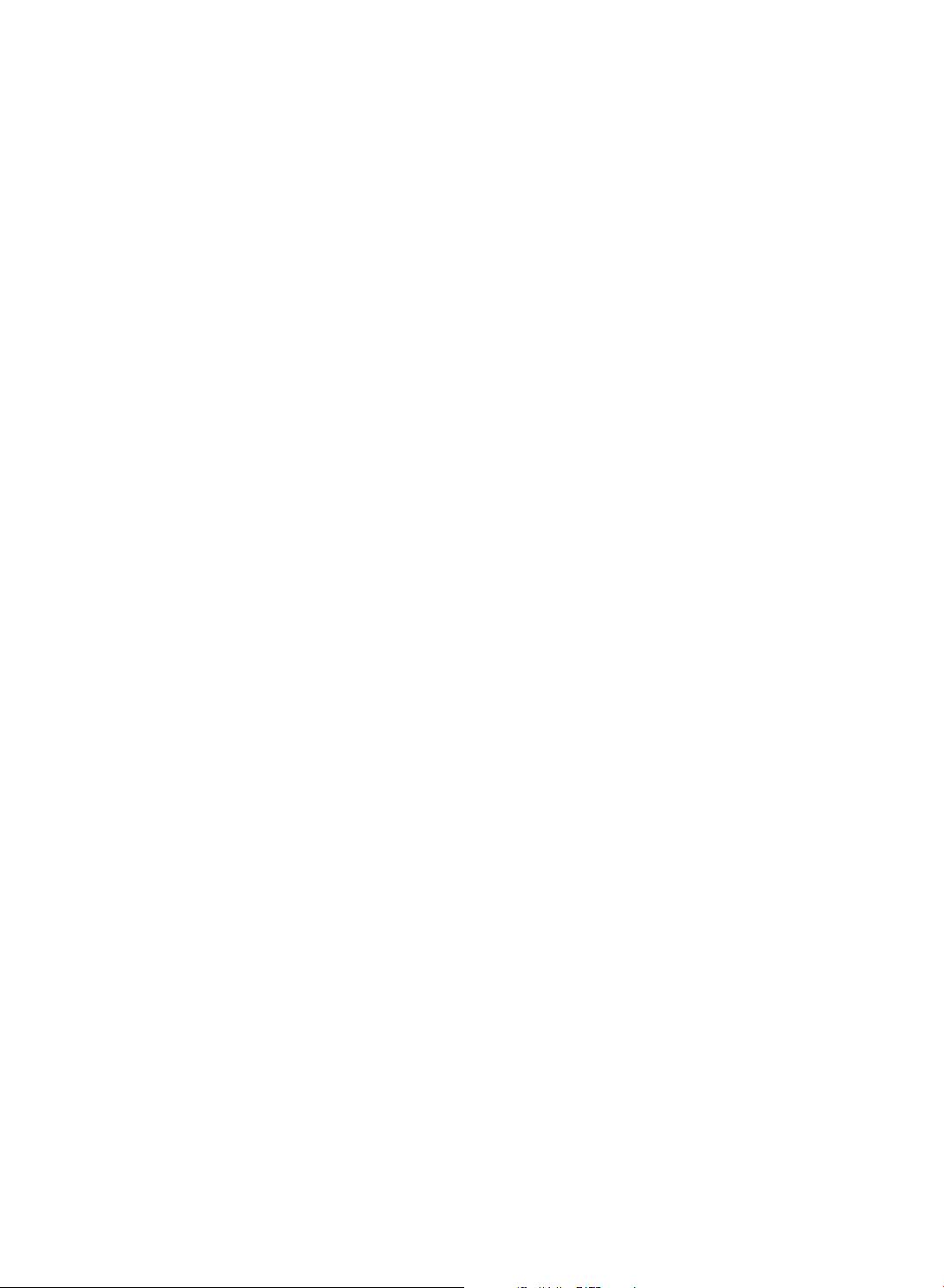
H. Liao and Z. Xu / Multi-criteria decision making with intuitionistic fuzzy PROMETHEE 1705
there is no criteria such that b is strongly better than a,
then a will be preferred over b [1, 15, 17, 31]. Based
on the positive outranking flow, the negative outranking
flow and the net outranking flow, the PROMETHEE can
derive partial or complete ranking of alternatives [13].
Some scholars have compared these two methods, and
also with some utility theory based methods (for more
details, readers can see [16, 26, 38]). The ELECTRE
requires the inputs of preference parameters which can
be determined by the decision maker directly or through
inference from answers to a series of questions. All the
information required in the PROMETHEE is clear and
easy to define; meanwhile, the method is easy to imple-
ment and also useful in handling incomparability. This
paper focuses on the later method, i.e., PROMETHEE,
and tries to investigate its new extension for MCDM
under uncertainty.
Since it was firstly appeared in 1982 [10], the
PROMETHEE method has been extended into different
forms, especially for the fuzzy input data (see Sec-
tion 2.1 for details). After analyzing all these extended
forms deeply, we can find that the central idea to these
extensions is that they are based on different types of
fuzzy inputs respectively, such as interval numbers,
fuzzy numbers, fuzzy intervalnumbers, and generalized
fuzzy numbers. Some limitation may arise due to these
fuzzy sets themselves since all the above mentioned
forms of fuzzy sets are characterized by single-valued
functions of membership, which can’t be used to
express the support and objection evidences simulta-
neously. While, another extended form of fuzzy set,
named intuitionistic fuzzy set (IFS) [5, 6], which is char-
acterized by a membership function, a non-membership
function and a hesitancy function, can solve this
problem perfectly. Once not possessing a precise or
sufficient level of knowledge over the problem domain
due to the complexity, people usually have some uncer-
tainty in assigning the preference evaluation values to
the objects considered, which makes the judgments
of cognitive performance exhibit the characteristics of
affirmation, negation and hesitation. Due to the defi-
nitely powerfulness of modeling humans’ perception
and cognition, the IFS has attracted more and more
scholars’ attention and has been applied to many differ-
ent fields [5, 6, 23, 24, 28, 32, 33, 35, 36]. As to the two
above-mentioned outranking methods, the integration
of intuitionistic fuzzy logic into ELECTRE seems to be
more advanced [31]. Generally, PROMETHEE could
also assimilate the intuitionistic fuzzy set in express-
ing the preferences of the decision maker. Therefore,
to make the PROMETHEE method more applicable in
handling uncertain information, in this paper we try to
extend the PROMETHEE method within the context of
IFSs, which is the focus of this paper.
The remainder of this paper is set out as follows.
Section 2 briefly reviews the recent advances
in PROMETHEE and describes the classical
PROMETHEE II method. Some basic knowledge
about intuitionistic fuzzy set is also given in this sec-
tion. Section 3 proposes the IF-PROMETHEE method.
Two illustrative examples are given in Section 4 to
show the applicability and efficiency of the developed
method. The paper ends with some concluding remarks
in Section 5.
2. Preliminaries
2.1. Extensions of the PROMETHEE methods
The main idea of PROMETHEE is to derive a par-
tial or complete ranking of alternatives based on the
positive outranking flow, the negative outranking flow
and the net outranking flow. Many different types of
PROMETHEE methods used in different circumstances
have been proposed in recent years. The PROMETHEE
I (partial ranking) and the PROMETHEE II (complete
ranking) were developed by Brans [10]. After that, sev-
eral different kinds of PROMETHEE versions were
proposed, such as the PROMETHEE III (ranking based
on intervals) and the PROMETHEE IV (continuous
case). The PROMETHEE V was proposed to han-
dle problems with segmentation constraints [11]. The
PROMETHEE VI was introduced for the representation
of the human brain [12]. The PROMETHEE GDSS was
developed for group decision-making and the visual
interactive module GAIA was established for graphi-
cal representation [13]. Due to the simplicity in both
concept and application, the family of PROMETHEE
methods has been applied successfully in many fields,
such as environment management [38], business and
financial management [3], information technology [2],
project selection [19] and so on. Behzadian et al. [7]
made a literature review on the applications of the
PROMETHEE methods.
Although those PROMETHEE methods are useful in
handling specific MCDM problem, there are still some
drawbacks arisen from the fact that the above mentioned
PROMETHEE methods are all modeled with the crisp
input data, which is not the usual case since fuzziness
and impreciseness are common in our daily life. The
fuzzy input data involved in a decision making problem
arises from: