没有合适的资源?快使用搜索试试~ 我知道了~
首页贝叶斯网络导论(An Introduction to Bayesian Networks)
资源详情
资源评论
资源推荐
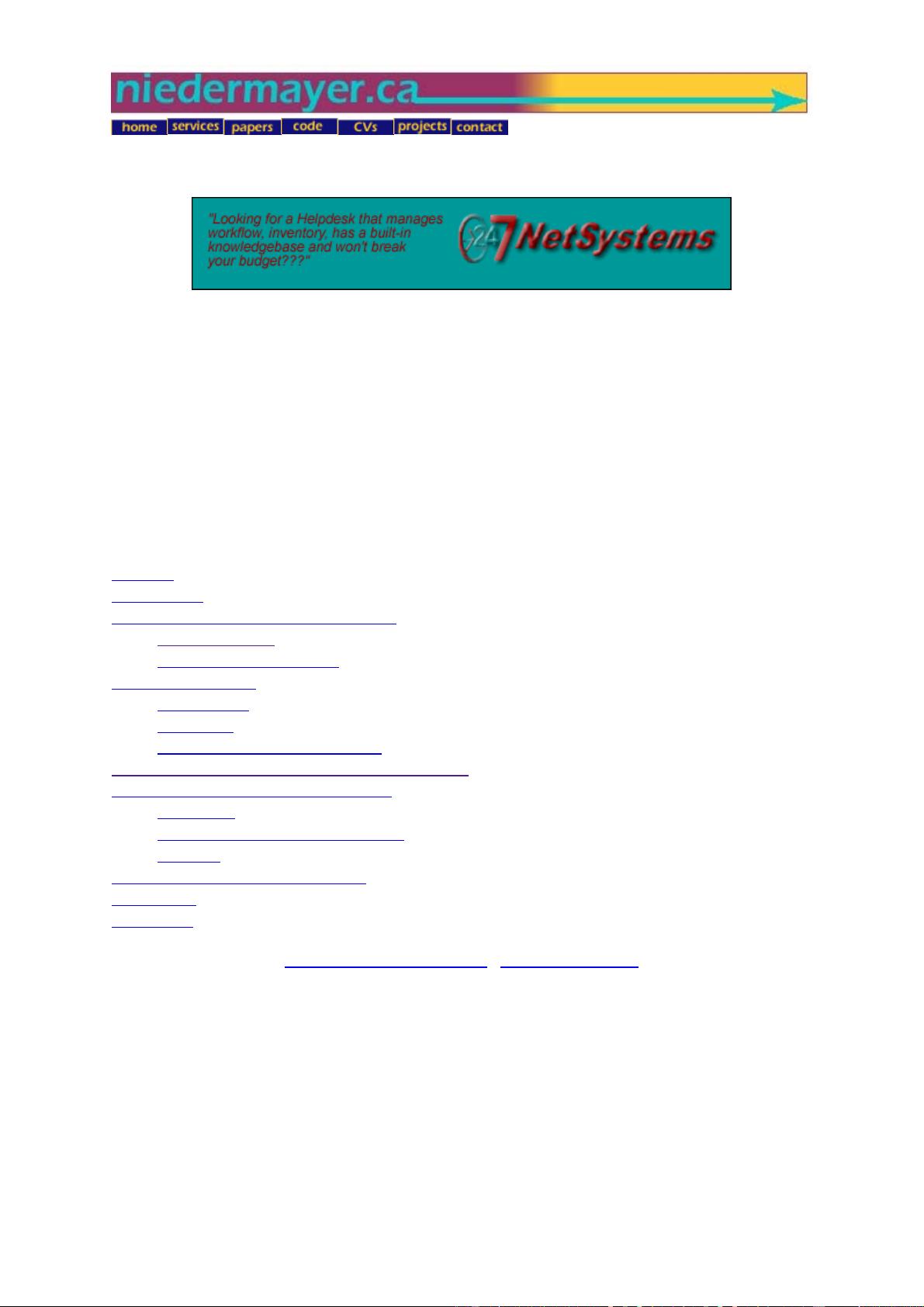
An Introduction to Bayesian Networks and their Contemporary Applic... http://www.niedermayer.ca/papers/bayesian
/
1 of 12 17/10/2005 09:51
An Introduction to Bayesian Networks and their
Contemporary Applications
Daryle Niedermayer, I.S.P., PMP, B.Sc., B.A., M.Div.
December 1, 1998
Table of Contents
Abstract1.
Introduction
2.
An Introduction to Bayesian Inference
Bayes Theoremi.
Bayes Theorem Applied
ii.
3.
Bayesian Networks
Introductioni.
Definition
ii.
Bayesian Networks Illustrated
iii.
4.
Algorithmic Implications of Bayesian Networks
5.
Practical Uses for Bayesian Networks
AutoClassi.
Introduction of Search Heuristics
ii.
Lumiere
iii.
6.
Limitations of Bayesian Networks
7.
Conclusion
8.
References
9.
Return to My Home Page
| My Other Papers
Abstract
Bayesian Networks are becoming an increasingly important area for research and application in the entire
field of Artificial Intelligence. This paper explores the nature and implications for Bayesian Networks
beginning with an overview and comparison of inferential statistics and Bayes' Theorem. The nature,
relevance and applicability of Bayesian Network theory for issues of advanced computability forms the
core of the current discussion. A number of current applications using Bayesian networks is examined.
The paper concludes with a brief discussion of the appropriateness and limitations of Bayesian Networks
for human-computer interaction and automated learning.
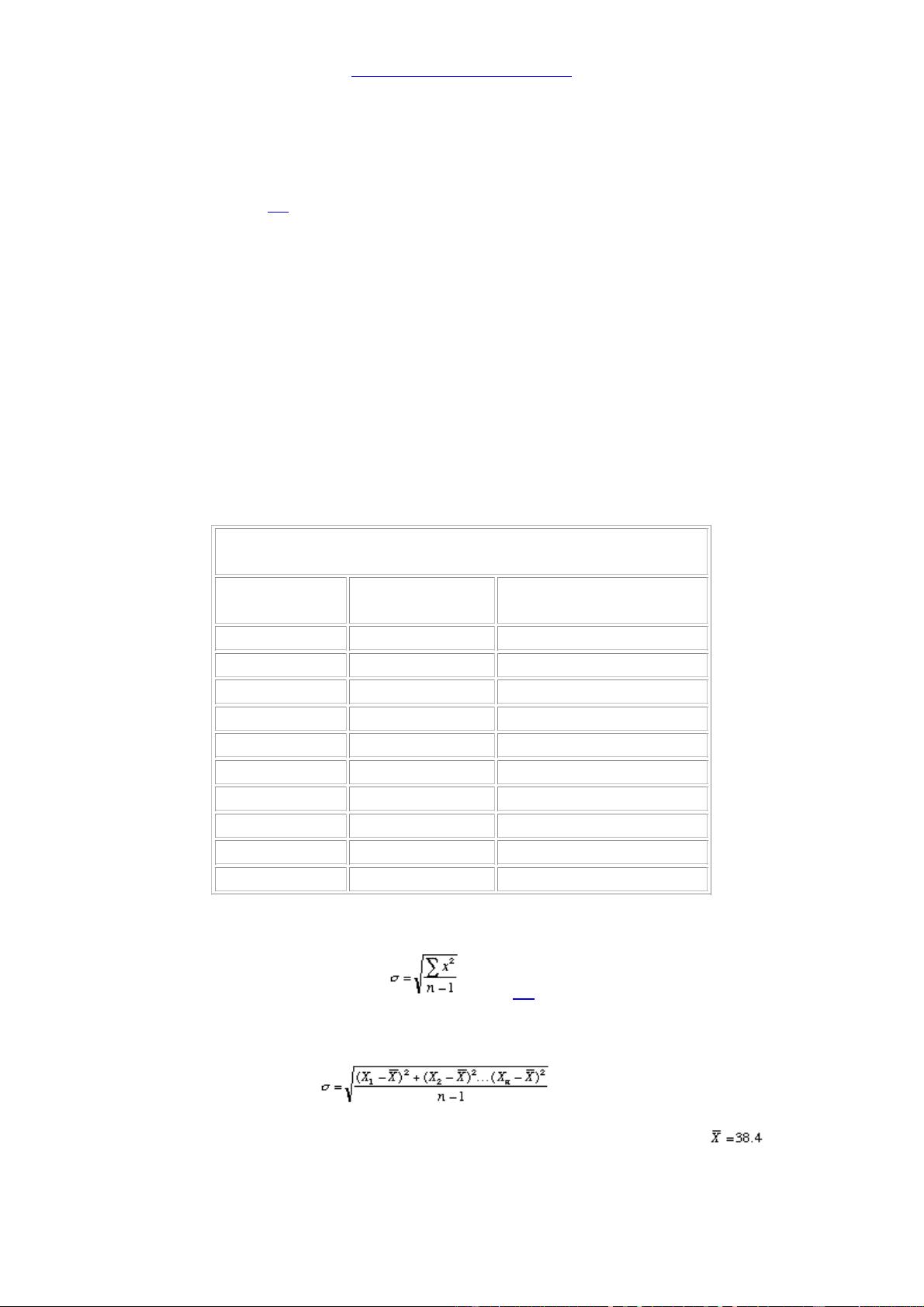
An Introduction to Bayesian Networks and their Contemporary Applic... http://www.niedermayer.ca/papers/bayesian
/
2 of 12 17/10/2005 09:51
Back to the Table of Contents
Introduction
Inferential statistics is a branch of statistics that attempts to make valid predictions based on only a sample
of all possible observations[1]
. For example, imagine a bag of 10,000 marbles. Some are black and some
white, but of which the exact proportion of these colours is unknown. It is unnecessary to count all the
marbles in order to make some statement about this proportion. A randomly acquired sample of 1,000
marbles may be sufficient to make an inference about the proportion of black and white marbles in the
entire population. If 40% of our sample are white, then we may be able to infer that about 40% of the
population are also white.
To the layperson, this process seems rather straight forward. In fact, it might seem that there is no need to
even acquire a sample of 1,000 marbles. A sample of 100 or even 10 marbles might do.
This is assumption is not necessarily correct. As the sample size becomes smaller, the potential for error
grows. For this reason, inferential statistics has developed numerous techniques for stating the level of
confidence that can be placed on these inferences.
If we took ten samples of 100 marbles each, we might find the following results:
Table 1: Relative proportions of 10 samples from a
population of 10,000
Sample Number
Number of White
Marbles
Number of Black Marbles
1
40 60
2
35 65
3
47 53
4
50 50
5
31 69
6
25 75
7
36 64
8
20 80
9
45 55
10
55 45
We are then in a position to calculate the "Standard Deviation" of these samples:
(eq. 1)[2]
where x
2
is the sum of the squares so that the equation is expanded to:
(eq. 2)
and n is the number of samples. In our example, the mean number of White marbles is
.
We might be tempted to say that about 40% of the marbles are white, but we are unable to argue that point
with any degree of certainty. Using equation 2 above, we determine that the Standard Deviation is 11.15.
We must then determine the "Sample Error of the Mean" (where s=[sigma]):
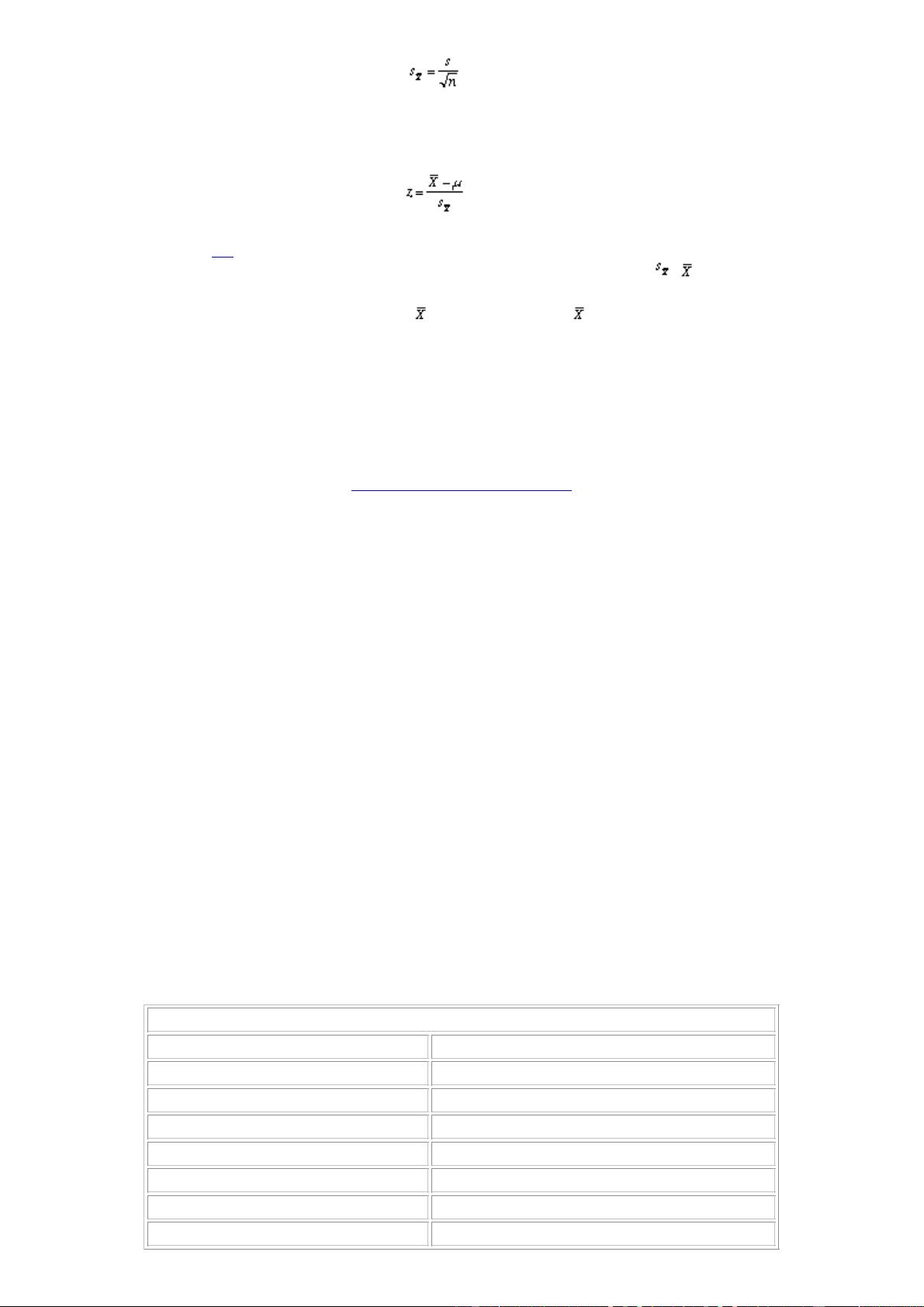
An Introduction to Bayesian Networks and their Contemporary Applic... http://www.niedermayer.ca/papers/bayesian
/
3 of 12 17/10/2005 09:51
(eq. 4)
The confidence we can put on our hypothesis that u=40 of the marbles are white is found using a standard
statistical test called the "z-test":
(eq. 5)
Using a z-test table [3]
and our resulting z-value of -.4532, we find that 32% of the area of the normal
curve would fall below this "z" value. In other words, in 32% of samples given
, would be less than
38.4. Since the distribution is two-sided or "two-tailed" (i.e. the sample average could also be greater than
the population average), we would also expect
to greater than ((u- )+u=) 41.6 in another 32% of cases.
In summary, if we expect 40% of all marbles in the bag to be white, then a series of ten samples with only
38.4% of marbles being white would be expected in (100-64%=) 36% of the time. Clearly, the confidence
we can place in our conclusion is not as good as it was on first glance. This lack of confidence is due to
the high variability among the samples. If we took more samples or larger samples, our confidence in our
conclusion might increase.
Back to the Table of Contents
An Introduction to Bayesian Inference
Classical inferential models do not permit the introduction of prior knowledge into the calculations. For
the rigours of the scientific method, this is an appropriate response to prevent the introduction of
extraneous data that might skew the experimental results. However, there are times when the use of prior
knowledge would be a useful contribution to the evaluation process.
Assume a situation where an investor is considering purchasing some sort of exclusive franchise for a
given geographic territory. Her business plan suggests that she must achieve 25% of market saturation for
the enterprise to be profitable. Using some of her investment funds, she hires a polling company to
conduct a randomized survey. The results conclude that from a random sample of 20 consumers, 25% of
the population would indeed be prepared to purchase her services. Is this sufficient evidence to proceed
with the investment?
If this is all the investor has to go on, she could find herself on her break-even point and could just as
easily turn a loss instead of a profit. She may not have enough confidence in this survey or her plan to
proceed.
Fortunately, the franchising company has a wealth of experience in exploiting new markets. Their results
show that in 20% of cases, new franchises only achieve a 25% market saturation, while in 40% of cases,
new franchises achieve a 30% market saturation. The entire table of their findings appears below:
Table 2: Percent of New Franchises achieving a given Market Saturation
Market Saturation (Proportion) =p Percent of Franchises (Relative Frequency)
0.10 0.05
0.15 0.05
0.20 0.20
0.25 0.20
0.30 0.40
0.35 0.10
Total = 1.00
剩余11页未读,继续阅读
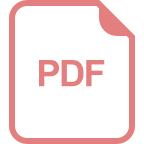
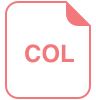
















dichcode
- 粉丝: 3
- 资源: 13
上传资源 快速赚钱
我的内容管理 收起
我的资源 快来上传第一个资源
我的收益
登录查看自己的收益我的积分 登录查看自己的积分
我的C币 登录后查看C币余额
我的收藏
我的下载
下载帮助

会员权益专享
最新资源
- RTL8188FU-Linux-v5.7.4.2-36687.20200602.tar(20765).gz
- c++校园超市商品信息管理系统课程设计说明书(含源代码) (2).pdf
- 建筑供配电系统相关课件.pptx
- 企业管理规章制度及管理模式.doc
- vb打开摄像头.doc
- 云计算-可信计算中认证协议改进方案.pdf
- [详细完整版]单片机编程4.ppt
- c语言常用算法.pdf
- c++经典程序代码大全.pdf
- 单片机数字时钟资料.doc
- 11项目管理前沿1.0.pptx
- 基于ssm的“魅力”繁峙宣传网站的设计与实现论文.doc
- 智慧交通综合解决方案.pptx
- 建筑防潮设计-PowerPointPresentati.pptx
- SPC统计过程控制程序.pptx
- SPC统计方法基础知识.pptx
资源上传下载、课程学习等过程中有任何疑问或建议,欢迎提出宝贵意见哦~我们会及时处理!
点击此处反馈


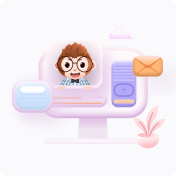
安全验证
文档复制为VIP权益,开通VIP直接复制

评论8