没有合适的资源?快使用搜索试试~ 我知道了~
首页非线性时间序列:非参数和参数方法
资源详情
资源推荐
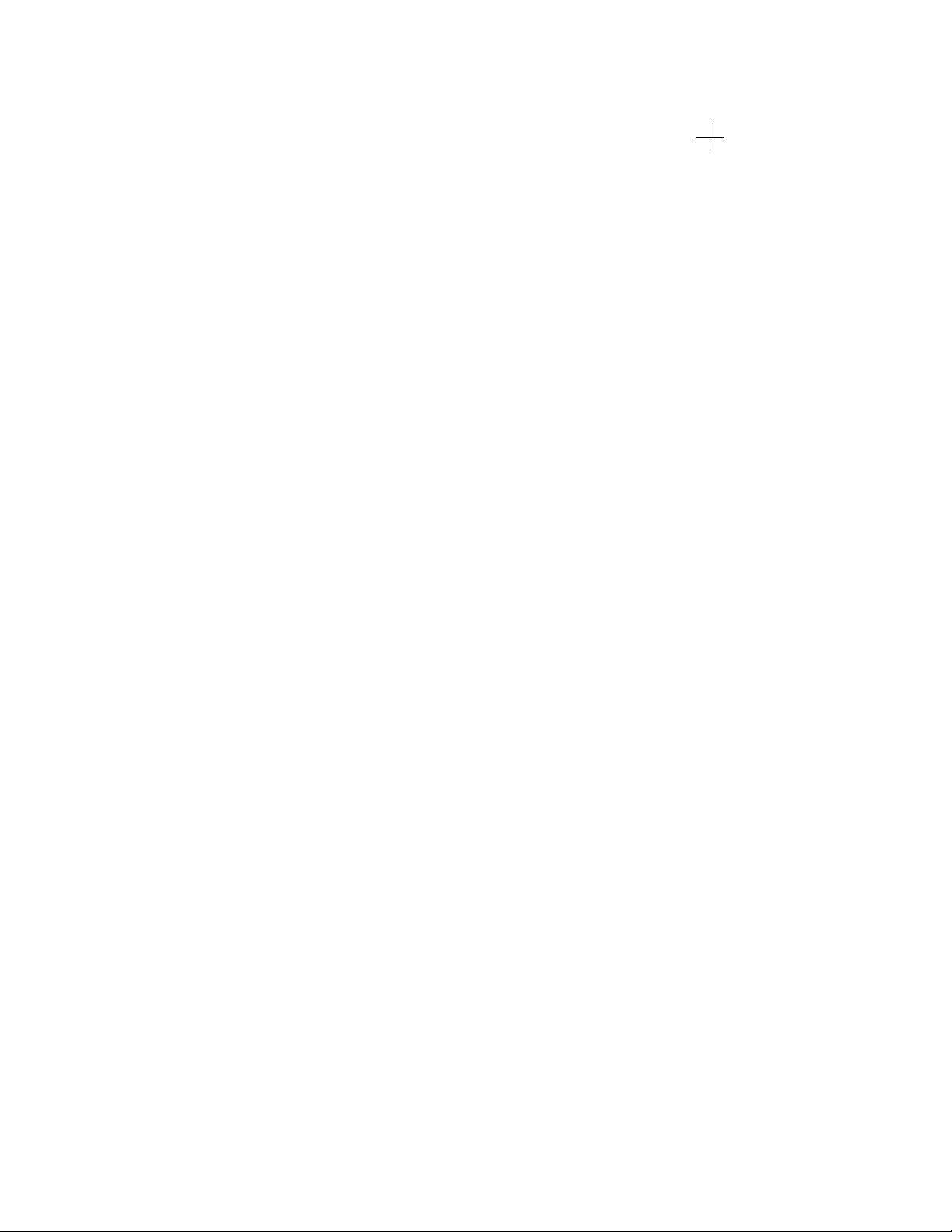
This is page i
Printer: Opaque this
Jianqing Fan
Qiwei Yao
Nonlinear Time Series:
Nonparametric and Parametric Meth-
ods
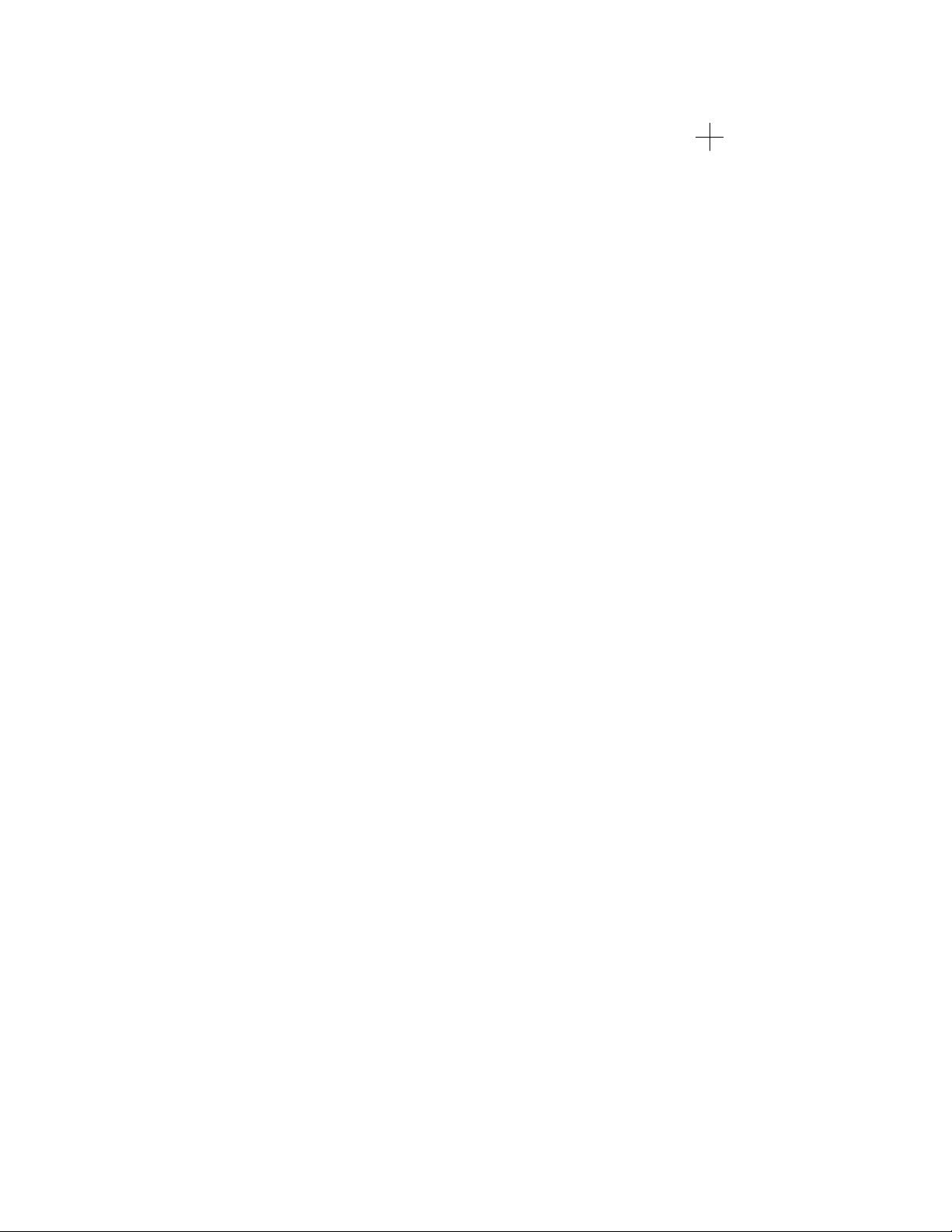
This is page ii
Printer: Opaque this
TO THOSE
WHO EDUCATE US;
WHOM WE LOVE; AND
WITH WHOM WE COLLABORATE
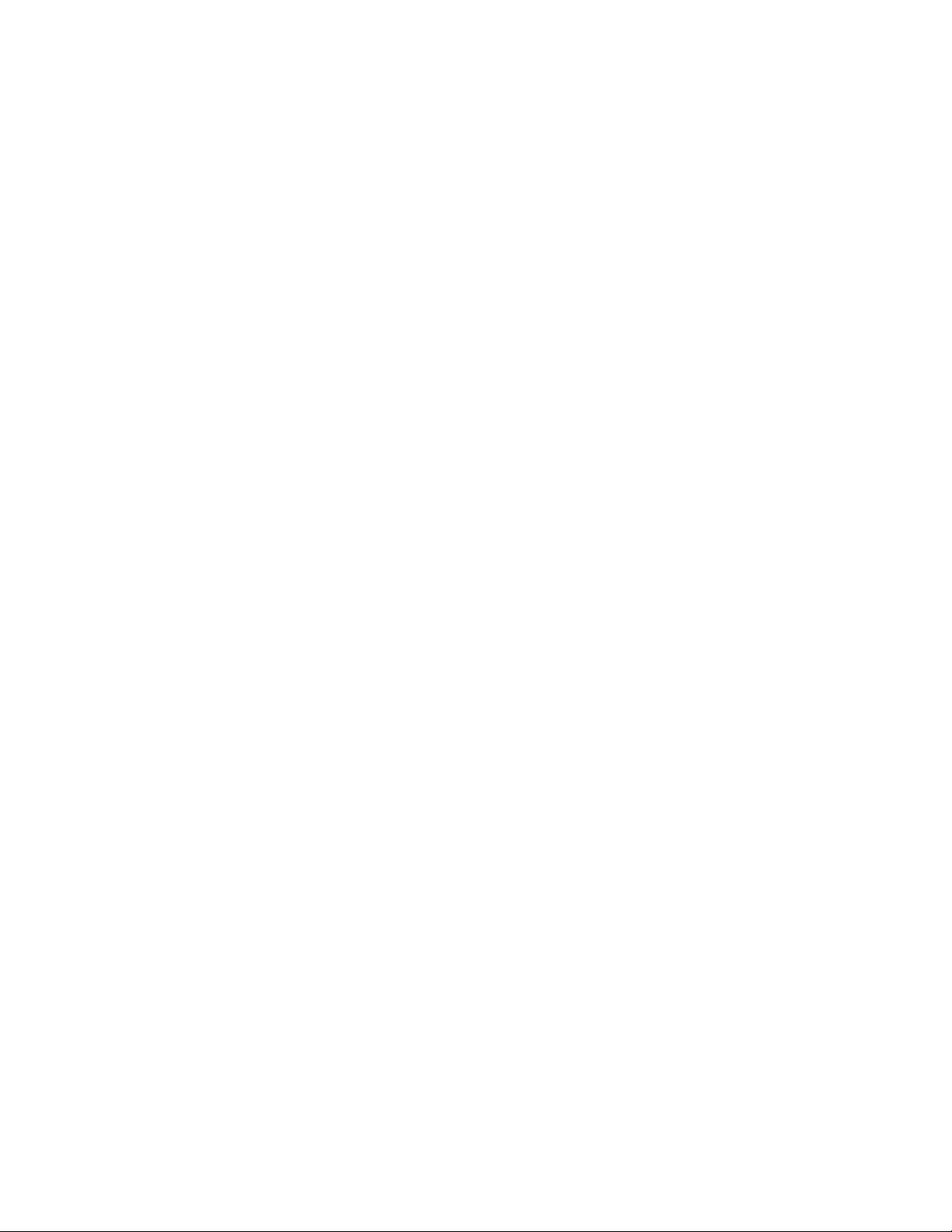
iii
Back-cover
This book is on the contemporary statistical methods and theory of
nonlinear time series analysis. The principal focus is on nonparametric
and semiparametric techniques developed in the last decade. It covers the
techniques for modelling in state-space, in frequency-domain as well as in
time-domain. To reflect the integration of parametric and nonparametric
methods in analyzing time series data, the book also presents an up-to-date
exposure on some parametric nonlinear models, including ARCH/GARCH
models and threshold models. A compact view on linear ARMA models
is also provided. Data arising in real applications are used throughout to
show how nonparametric approaches may help to reveal local structure in
high-dimensional data. Important technical tools are also introduced.
The book is designed to appeal to graduate students, application-oriented
time series analysts, new and experienced researchers. It will have the value
both within the statistical community and across a broad spectrum of other
fields such as econometrics, empirical finance, population biology and ecol-
ogy. The prerequisites are merely sound basic courses in probability and
statistics.
Jianqing Fan, coauthor of the highly regarded b ook Local Polynomial
Modeling, is Professor of Statistics at the University of North Carolina at
Chapel Hill and the Chinese University of Hong Kong. His published work
on nonparametric modeling, nonlinear time series, financial econometrics,
analysis of longitudinal data, model selection, wavelets and other aspects
of methodological and theoretical statistics has been recognized with the
Presidents’ Award from the Committee of Presidents of Statistical Soci-
eties, the Hettleman Prize for Artistic and Scholarly Achievement from the
University of North Carolina, and by his election as a fellow of the Ameri-
can Statistical Association and the Institute of Mathematical Statistics.
Qiwei Yao is Professor of Statistics at the London School of Economics
and Political Science. He is an elected member of the International Sta-
tistical Institute, has served on editorial board for Journal of the Royal
Statistical Society (Series B) and Australian and New Zealand Journal of
Statistics.
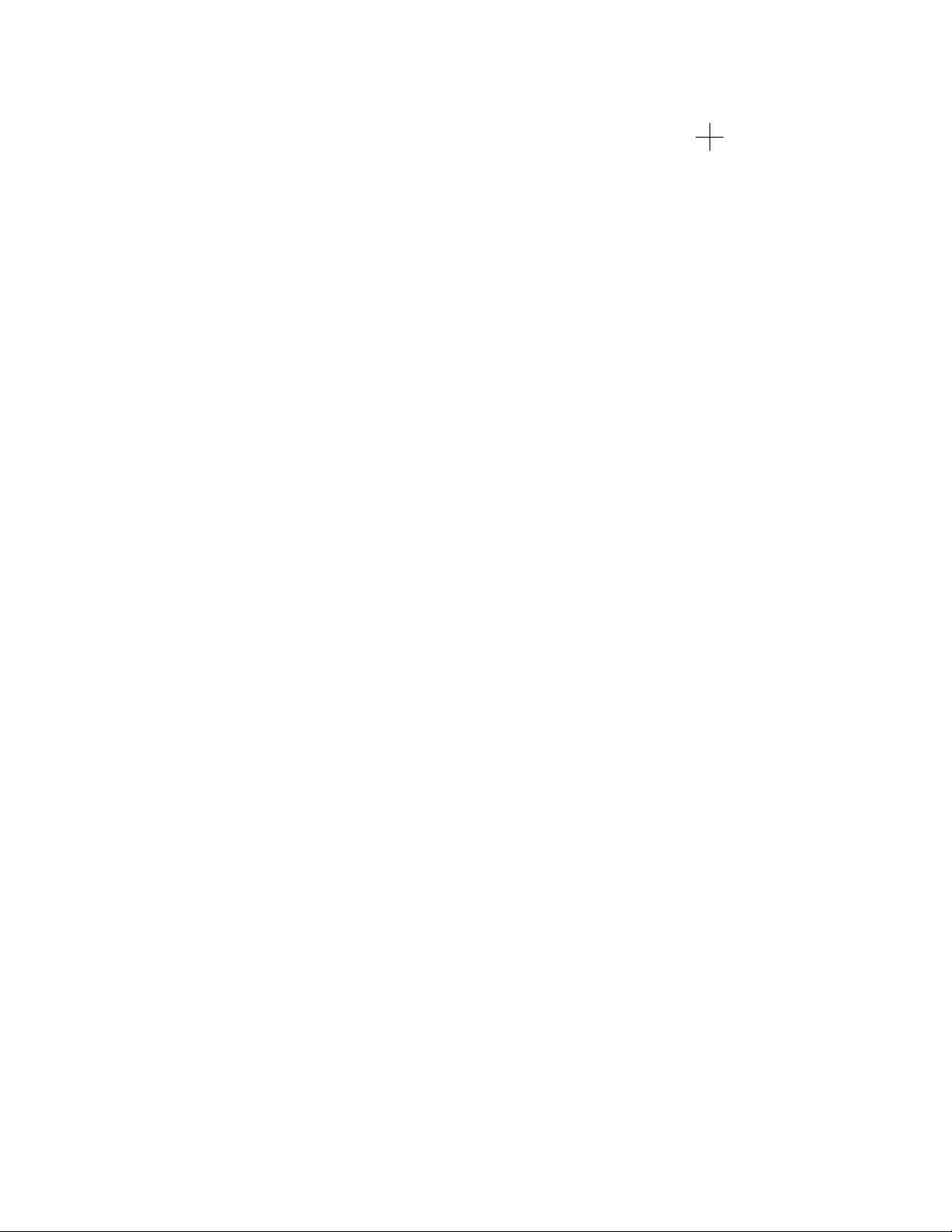
This is page v
Printer: Opaque this
Preface
Among many exciting developments in statistics over the last two decades,
Nonlinear Time Series and Data-analytic Nonparametric Methods have
greatly advanced along seemingly unrelated paths. In spite of the fact that
the application of nonparametric techniques in time series can be backdated
to as early as 1940’s, there still exists healthy and justified skepticism about
the capability of nonparametric methods in analyzing time series data. As
enthusiastic explorers of the modern nonparametric tool-kit, we feel obliged
to assemble together the newly developed relevant techniques in one place.
The aim of this book is to advocate those modern nonparametric techniques
which have proved useful for analyzing real time series data, and to provoke
further research in both methodology and theory for nonparametric time
series analysis.
Exponential increases in computing power (Moore’s law) and falling costs
have had a profound impact on the development of statistics including time
series. Modern computers and the information age have brought us oppor-
tunities with challenges. Technological inventions have lead to the explosion
in data collection (e.g. daily grocery sales, stock market trading, microar-
ray data). The internet makes big data warehouses readily accessible. It
has become commonplace to collect and analyze time series data of un-
precedented size and complexity. Whilst classic parametric models, which
postulate global structures for underlying systems, are still very useful,
large sizes of data sets invite the search for more refined structures, which
leads to better understandings and approximations of the real world. Be-
yond postulated parametric models, there are infinite other possibilities.
Nonparametric techniques provide useful exploratory tools to this venture,
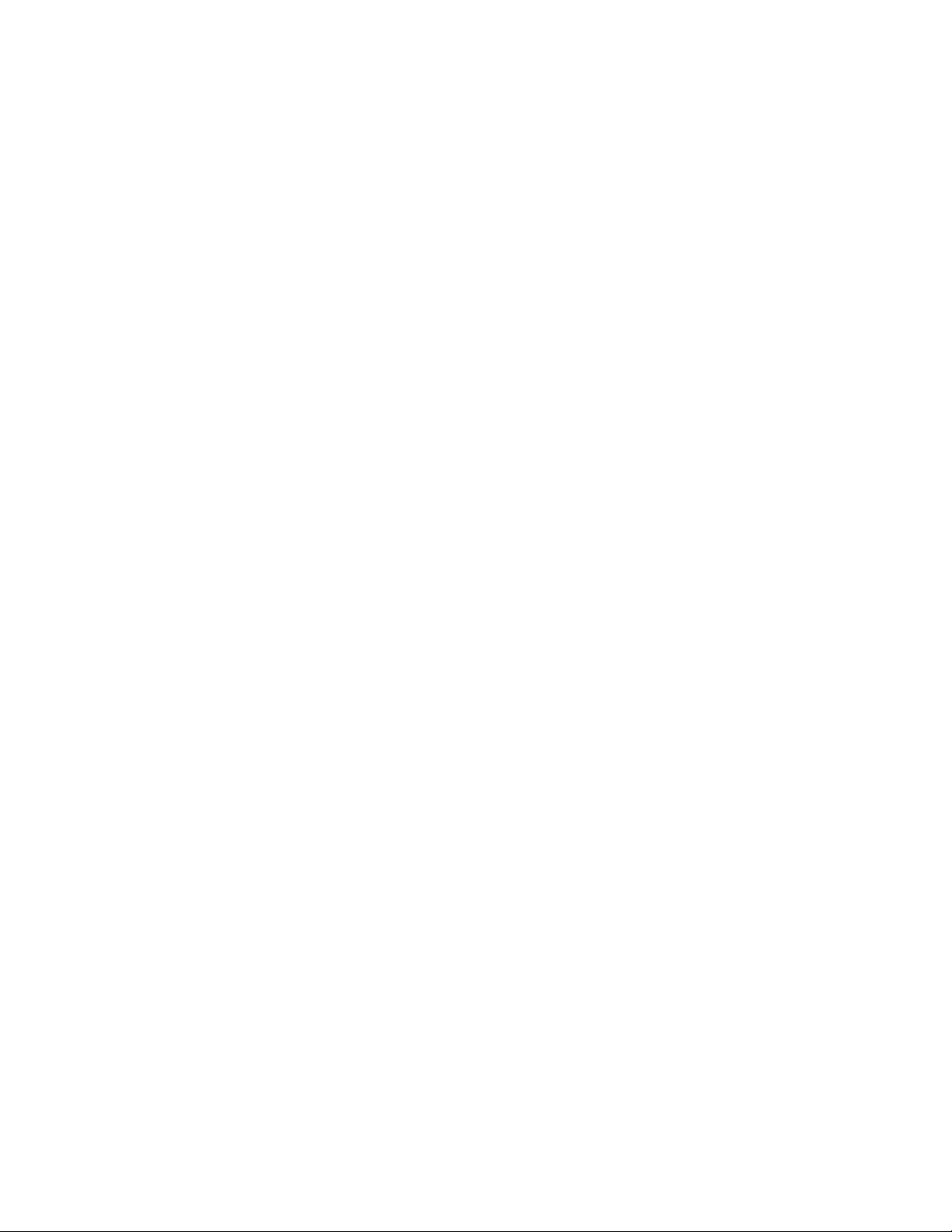
vi PREFACE
including the suggestion of new and the validating of existing parametric
models.
In this book, we present an up-to-date picture of techniques for analyz-
ing time series data. Although we have tried to maintain a good balance
among methodology, theory, and numerical illustration, our primary goal
is to present a comprehensive and self-contained account for each of the
key methodologies. For practical relevant time series models, we aim for
exposure with definition, probability properties (if possible), statistical in-
ference methods, and numerical examples with real data sets. We also in-
dicate where to find our (only our!) favorite computing codes to implement
these statistical methods. When soliciting real-data examples, we attempt
to maintain a good balance among different disciplines, though our per-
sonal interests in quantitative finance, risk management, and biology can
be easily seen. It is our hope that readers can apply these techniques to
their own data sets.
We trust that the book will be of interest to those coming to the area
for the first time, and to readers more familiar with the field. Application-
oriented time series analysts will also find this book useful as it focuses on
methodology and includes several case studies with real data sets. We be-
lieve that nonparametric methods must go hand in hand with parametric
methods in applications. In particular, parametric models provide explana-
tory power and concise descriptions of the underlying dynamics, which,
when used sensibly, is an advantage over nonparametric models. For this
reason, we have also provided a compact view on the parametric methods
for both linear and selected nonlinear time series models. This will also
give new comers sufficient information on the essence of the more classical
approaches. We hope that this book will reflect the power of the integration
of nonparametric and parametric approaches in analyzing time series data.
The book has been prepared for a broad readership — the prerequisites are
merely sound basic courses in probability and statistics. Whilst advanced
mathematics has provided valuable insights into nonlinear time series, the
methodological power of both nonparametric and parametric approaches
can be understood without sophisticated technical details. Due to the in-
nate nature of the subject, it is inevitable that we occasionally appeal for
more advanced mathematics; such sections are marked with a ‘*’. Most
technical arguments are collected in ‘Complements’ section at the end of
each chapter, but key ideas are left within text.
The introduction in Chapter 1 sets the scene for the book. Chapter 2
deals with basic probabilistic properties of time series processes. The high-
lights include strict stationarity via ergodic Markov chains (§2.1) and mix-
ing properties (§2.6). We also provide a generic central limit theorm for
kernel-based nonparametric regression estimation for α-mixing processes.
A compact view of linear ARMA models is given in Chapter 3, including
Gaussian MLE (§3.3), model selection criteria (§3.4), and linear forecasting
with ARIMA models (§3.7). Chapter 4 introduces three types of paramet-
剩余545页未读,继续阅读
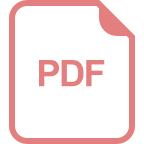

















microfisher
- 粉丝: 3
- 资源: 10
上传资源 快速赚钱
我的内容管理 收起
我的资源 快来上传第一个资源
我的收益
登录查看自己的收益我的积分 登录查看自己的积分
我的C币 登录后查看C币余额
我的收藏
我的下载
下载帮助

会员权益专享
最新资源
- 京瓷TASKalfa系列维修手册:安全与操作指南
- 小波变换在视频压缩中的应用
- Microsoft OfficeXP详解:WordXP、ExcelXP和PowerPointXP
- 雀巢在线媒介投放策划:门户网站与广告效果分析
- 用友NC-V56供应链功能升级详解(84页)
- 计算机病毒与防御策略探索
- 企业网NAT技术实践:2022年部署互联网出口策略
- 软件测试面试必备:概念、原则与常见问题解析
- 2022年Windows IIS服务器内外网配置详解与Serv-U FTP服务器安装
- 中国联通:企业级ICT转型与创新实践
- C#图形图像编程深入解析:GDI+与多媒体应用
- Xilinx AXI Interconnect v2.1用户指南
- DIY编程电缆全攻略:接口类型与自制指南
- 电脑维护与硬盘数据恢复指南
- 计算机网络技术专业剖析:人才培养与改革
- 量化多因子指数增强策略:微观视角的实证分析
资源上传下载、课程学习等过程中有任何疑问或建议,欢迎提出宝贵意见哦~我们会及时处理!
点击此处反馈


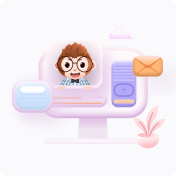
安全验证
文档复制为VIP权益,开通VIP直接复制
