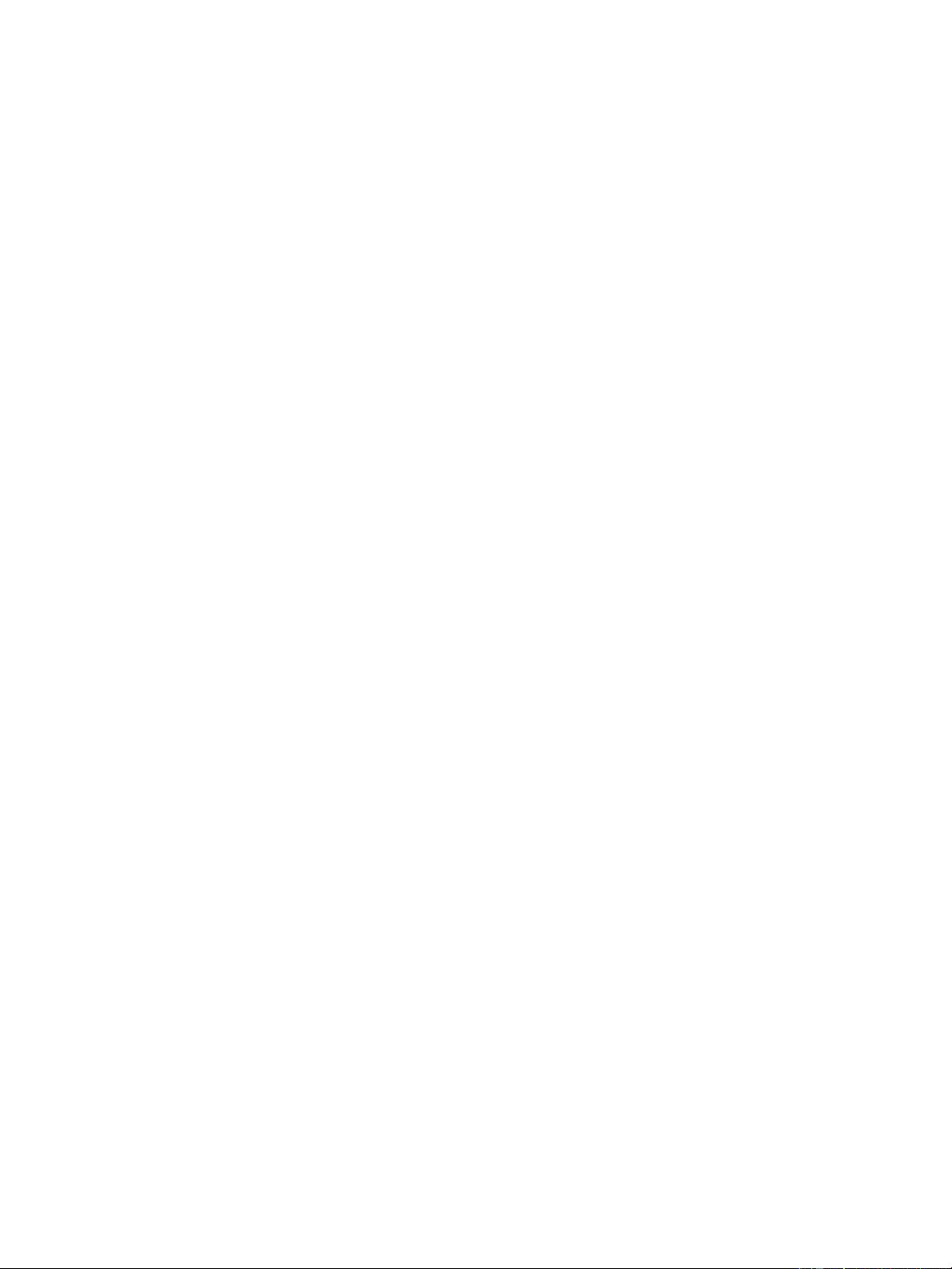
1658 IEEE JOURNAL OF SELECTED TOPICS IN APPLIED EARTH OBSERVATIONS AND REMOTE SENSING, VOL. 11, NO. 5, MAY 2018
Let x
(i)
and y
(j )
denote the ith input feature map and jth
output feature map of a convolutional layer. Therefore, a con-
volutional operation with activation function applied to x
(i)
can
be expressed as
y
(j )
= f
b
(j )
+
i
k
(i)(j )
∗ x
(i)
(1)
where k
(i)(j )
is a convolutional kernel which is applied to ith
input feature map to obtain the jth output feature map, and b
(j )
indicates the bias. The symbol ∗ indicates the convolutional op-
erator and f indicates the activation function. If a convolutional
layer consists of M input feature maps and N output feature
maps, there will be N convolutional kernels with the size of
d × d × M, where d × d also indicates the size of local recep-
tive fields. Besides, each kernel contains a bias. Selection of
proper activation function is an essential part of a neural net-
work, and conventional activation functions include Sigmoid,
Tanh, and ReLU [35]. For example, (1) can be re-expressed as
the following by incorporating the nonlinear ReLU activation
function:
y
(j )
= max
0,b
(j )
+
i
k
(i)(j )
∗ x
(i)
. (2)
B. Remote Sensing Image Fusion Based on CNN
Most of current remote sensing image fusion methods usu-
ally contain two steps: Feature extraction and feature fusion.
For example, when the multiresolution analysis or sparse rep-
resentation is used to achieve image fusion, the first step is to
express the source images via a series of base filters or atoms in
a dictionary. After the expressions are derived, the second step
is to choose appropriate strategies, such as weights differences,
to fuse expressions of source images so that expressions of the
fusion image can be generated. It is noteworthy that all of the
procedures can also be equalized to apply different convolu-
tional kernels to achieve feature extraction and feature fusion.
We will demonstrate this operation in Section III-A in detail.
Therefore, as convolutional layers can achieve the effect as same
as traditional fusion methods, it is reasonable and reliable to use
CNN to extract the characteristics of different remote sensing
images and fuse them to obtain f usion image.
The conventional CNN is usually adopted to solve the im-
age classification problems [30], [54]. By putting an image into
networks, the output will be the probability of the image be-
longing to each category. While putting CNN to deal with im-
age super-resolution reconstruction issues, the designed CNN
removes the pooling procedure, and the output of the network is
reconstructed images which have the same size as input images.
Specifically, inputs and labels for network training are low-
resolution and high-resolution images respectively [36], [37].
In order to lower the difference between network outputs and
labels, the network will continuously learn parameters to fit la-
bels. To utilize CNN to remote sensing image fusion, we adopt
the same thoughts from the field of image super-resolution re-
construction. Fusion aims to generate a MS image with high
spatial resolution. Therefore, the label of the network is a high
spatial resolution MS image and the inputs are a PAN image
and a low spatial resolution MS image.
Presently, there are few deep learning based studies in re-
mote sensing image fusion. Masi et al. [39], Palsson et al. [40],
and Zhong et al. [55] directly adopted SRCNN [36], which is
a popular network for image super-resolution reconstruction,
to implement remote sensing image fusion. However, the first
two methods only use three convolutional layers and cannot
adequately leverage the depth of the network to extract deep
features. Also, these methods regard the PAN images as a band
and overlay it on the MS images to train t he network, which
ignores distinctive characteristics of these two types of images.
While the third method only uses SRCNN to do image super-
resolution rather than image fusion. The fusion procedure is still
finished by traditional Gram-Schmidt transform method.
III. P
ROPOSED FUSION FRAMEWORK
In this section, we demonstrate our remote sensing image
fusion method in detail. The network architecture is shown in
Fig. 2, which uses the acronym RSIFNN in the f ollowing, mean-
ing CNN-based remote sensing image fusion.
A. Network Design
The proposed method contains the same procedures as the
classical remote sensing fusion methods: Feature extraction and
feature fusion. Different from other deep learning-based fu-
sion methods [39], [40], [55], the proposed method design two
branches to extract features of the MS and PAN images sep-
arately. Fig. 2 shows the whole training scheme of RSIFNN,
where the input MS and PAN images generated from the Quick-
Bird satellite are of 2.8 m and 0.7 m spatial resolution.
The output of network should be the MS image with same
spatial resolution as the PAN image. This image needs to be as
similar as possible to the ideal MS image (label) which obtained
by a sensor with same spatial resolution as the PAN image.
However, this ideal MS image does not exist. It will cause
troubles for our training and performance assessment if there
do not exist labels. Fortunately, this problem can be solved by
using Wald’s protocol [38]. In Wald’s protocol, the original MS
images are regarded as labels. For keeping the same spatial
resolution as the original MS image (labels), the original PAN
image needs to be down-sampled according to the ratio between
the resolution of MS image and PAN image. At the same time,
the input MS image with low spatial resolution is generated
by down-sampling the original MS image and interpolating the
down-sampled data. Fig. 3 shows the procedure of preparing
training samples. In the case of QuickBird images, we have MS
images and PAN images of 2.8 m and 0.7 m spatial resolution.
The inputs are obtained by down-sampling the original MS and
PAN images by factor 4 (the multiple relationships between the
original MS images and PAN images) to get MS images and
PAN images of 11.2 m and 2.8 m spatial resolution. Therefore,
we can transform original fusion task to obtain fused MS images
(2.8 m) by fusing low-resolution MS images (11.2 m) and PAN
images (2.8 m). In this way, we have labels (original MS images)
Authorized licensed use limited to: Hebei University of Technology. Downloaded on March 26,2021 at 10:51:46 UTC from IEEE Xplore. Restrictions apply.