def forward(self, x): ''' Given input of size (batch_size x input_dim), compute output of the network ''' return self.net(x).squeeze(1) def cal_loss(self, pred, target): ''' Calculate loss ''' # TODO: you may implement L1/L2 regularization here eps = 1e-6 l2_reg = 0 alpha = 0.0001 #这段代码是l2正则,但是实际操作l2正则效果不好,大家也也可以调,把下面这段代码取消注释就行 # for name, w in self.net.named_parameters(): # if 'weight' in name: # l2_reg += alpha * torch.norm(w, p = 2).to(device) return torch.sqrt(self.criterion(pred, target) + eps) + l2_reg #lr_reg=0, 上面这段代码用的是均方根误差torch.sqrt(),均方根误差和kaggle评测指标一致,而且训练模型也更平稳
时间: 2024-02-14 12:31:55 浏览: 23
这段代码是定义了一个前向传播函数(forward)和计算损失函数(cal_loss)的方法。
在前向传播函数中,输入x经过self.net神经网络的计算得到输出,然后通过squeeze(1)操作将输出的维度从(batch_size, 1)压缩为(batch_size)。
在计算损失函数时,首先定义了一个很小的常数eps(用于数值稳定性),然后初始化L2正则化项l2_reg为0,并设置正则化系数alpha为0.0001。
接下来的注释部分是一个L2正则化的实现,遍历self.net神经网络的参数,如果参数名中包含'weight',则将该参数的L2范数加到l2_reg上。
最后,计算损失函数的值,使用了均方根误差(RMSE)作为主要的损失项。通过调用self.criterion计算预测值pred和目标值target之间的均方误差,再加上一个很小的常数eps,然后将L2正则化项l2_reg加到损失函数中。
综上所述,这段代码实现了一个带有L2正则化的损失函数的计算,并且使用均方根误差作为主要的损失项。
如果您有任何其他问题,请随时提问!
相关问题
def __len__(self): return math.ceil(len(self.x) / self.batch_size)
这是一个类的方法 `__len__`,它返回一个整数值,表示数据集的长度。
根据提供的代码,`__len__` 方法将 `self.x`(x 数据集)的长度除以 `self.batch_size`(批大小),并使用 `math.ceil` 函数向上取整。这是为了确保返回的长度是一个整数,而不是浮点数。
通过这个方法,可以方便地获取数据集的长度,以便在使用迭代器或者进行循环操作时确定迭代的次数。例如,如果数据集包含 10 个样本,批大小设置为 3,那么返回的长度将是 4,表示迭代器将在 4 次迭代中遍历整个数据集。
def forward(self, x): # [x_len, batch_size, d_model] """ :param x: [x_len, batch_size, emb_size] :return: [x_len, batch_size, emb_size] """ x = x + self.pe[:x.size(0), :] # [src_len,batch_size, d_model] + [src_len, 1, d_model] return self.dropout(x) # [src_len,batch_size, d_model]
这段代码是什么意思?
这段代码是一个Transformer模型的编码器或解码器中的一个self-attention层的前向传播函数。这个函数的输入x是一个形状为[x_len, batch_size, emb_size]的张量,表示输入序列的嵌入表示。这个函数的输出也是一个形状为[x_len, batch_size, emb_size]的张量,表示经过self-attention处理的序列的嵌入表示。
这个函数首先将输入张量x与self.pe(位置编码矩阵)相加,以加入位置信息。这里的self.pe是在模型初始化时构造的位置编码矩阵,它的形状为[max_len, 1, d_model],其中max_len是模型支持的最大序列长度,d_model是嵌入向量的维度。在这个函数中,我们使用了x.size(0)来截取self.pe的前x.size(0)行,以保证位置编码矩阵的长度与输入张量的长度相同。
接下来,将得到的结果通过dropout层进行处理,以减少过拟合的影响,并返回处理后的张量。
相关推荐
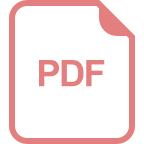
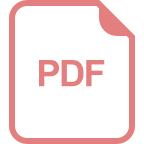













