python实现提取骨架算法
时间: 2023-07-26 20:18:14 浏览: 52
可以使用OpenCV库来实现骨架提取算法。以下是一个基于OpenCV的Python代码示例:
```python
import cv2
# 读取图像
img = cv2.imread("skeleton.png", 0)
# 二值化处理
ret, thresh = cv2.threshold(img, 127, 255, 0)
# 膨胀操作
kernel = cv2.getStructuringElement(cv2.MORPH_CROSS, (3, 3))
dilated = cv2.dilate(thresh, kernel, iterations=3)
# 骨架提取
skeleton = cv2.ximgproc.thinning(dilated)
# 显示结果
cv2.imshow("Original", img)
cv2.imshow("Skeleton", skeleton)
cv2.waitKey(0)
```
这个示例代码中,首先使用`cv2.imread()`函数读取图像,然后使用`cv2.threshold()`函数将图像二值化。接着,使用`cv2.dilate()`函数对二值图像进行膨胀操作,使得骨架更容易被提取。最后,使用`cv2.ximgproc.thinning()`函数提取骨架,得到提取出的骨架图像。
相关问题
骨架提取算法python
骨架提取算法,又称为细化算法,是一种可以将二值图像中的对象细化为单像素宽度的算法。下面是一个基于Python的骨架提取算法示例:
```python
import cv2
import numpy as np
def thinning(img):
# 定义细化算法需要的核
kernel = cv2.getStructuringElement(cv2.MORPH_CROSS, (3, 3))
size = np.size(img)
skel = np.zeros(img.shape, np.uint8)
# 循环直到没有像素需要细化为止
while True:
eroded = cv2.erode(img, kernel)
temp = cv2.dilate(eroded, kernel)
temp = cv2.subtract(img, temp)
skel = cv2.bitwise_or(skel, temp)
img = eroded.copy()
zeros = size - cv2.countNonZero(img)
if zeros == size:
break
return skel
# 读入二值图像
img = cv2.imread('image.png', 0)
# 对图像进行反色处理
img = cv2.bitwise_not(img)
# 进行骨架提取
skel = thinning(img)
# 显示结果
cv2.imshow('Original Image', img)
cv2.imshow('Thinning Image', skel)
cv2.waitKey(0)
cv2.destroyAllWindows()
```
在这个示例中,我们使用OpenCV库来读取和显示图像,并定义了一个名为"thinning"的函数来进行骨架提取。在该函数中,我们使用了腐蚀和膨胀操作来实现细化算法,并循环直到没有像素需要细化为止。最后,我们将得到的骨架图像显示出来。
骨架快速提取的算法python
常用的骨架快速提取算法有多种,其中一种是基于中轴线的细化算法,可以用Python实现。
1. 首先,将二值化的图像进行细化(或称为骨架化),得到中轴线。
2. 对中轴线进行拓扑分析,找到所有端点和交叉点。
3. 从任意一个端点出发,沿着中轴线向交叉点方向搜索,直到找到另一个端点为止。这一过程中,记录下搜索路径上的所有交叉点和端点,这些点就是骨架的分支点。
4. 对于每个分支点,将其周围的中轴线分成若干条子骨架,这些子骨架之间没有交叉点或端点,每个子骨架的起点是该分支点,终点是其周围的交叉点或端点。
5. 对于每个子骨架,用最小二乘法拟合一条直线,得到该子骨架的方向和长度。将所有子骨架的方向和长度记录下来,得到整个骨架的形状。
以下是一个基于OpenCV的Python代码示例,实现了基于中轴线的骨架快速提取算法:
```python
import cv2
import numpy as np
# 读入二值化图像
img = cv2.imread("binary_image.png", cv2.IMREAD_GRAYSCALE)
# 细化图像,得到中轴线
skel = cv2.ximgproc.thinning(img)
# 找到所有端点和交叉点
keypoints = cv2.xfeatures2d.StarDetector_create().detect(skel)
# 遍历所有端点,提取骨架
skeleton = []
for kp in keypoints:
if kp.response < 100: # 筛选出较长的端点
continue
path = [kp.pt] # 记录搜索路径
while True:
neighbors = cv2.getStructuringElement(cv2.MORPH_CROSS, (3, 3))
neighbors[1, 1] = 0
neighbor_kps = [kp for kp in cv2.KeyPoint_convert(cv2.KeyPointsFilter.filter(keypoints, neighbors))]
if len(neighbor_kps) == 1: # 到达另一个端点
path.append(neighbor_kps[0])
break
elif len(neighbor_kps) == 2: # 继续搜索
path.append(neighbor_kps[0])
path.append(neighbor_kps[1])
skel[int(path[-1][1]), int(path[-1][0])] = 0 # 避免重复搜索
kp = neighbor_kps[1] # 选取下一个邻居
else: # 遇到分叉点或环
break
if len(path) > 2: # 筛选出较长的骨架
skeleton.append(path)
# 提取骨架方向和长度
shapes = []
for path in skeleton:
coords = np.array(path, np.float32)
direction = cv2.fitLine(coords, cv2.DIST_L2, 0, 0.01, 0.01)
length = np.linalg.norm(coords[-1] - coords[0])
shapes.append((direction, length))
# 显示骨架
display_img = cv2.cvtColor(skel, cv2.COLOR_GRAY2BGR)
for shape in shapes:
pt1 = tuple(np.round(shape[0][:2] - shape[0][2:] * shape[1]).astype(int))
pt2 = tuple(np.round(shape[0][:2] + shape[0][2:] * shape[1]).astype(int))
cv2.line(display_img, pt1, pt2, (0, 0, 255), 2)
cv2.imshow("skeleton", display_img)
cv2.waitKey()
```
其中,使用了OpenCV的细化函数(`cv2.ximgproc.thinning`)和拟合直线函数(`cv2.fitLine`),以及Star特征检测器(`cv2.xfeatures2d.StarDetector_create`)。通过遍历所有端点,沿着中轴线搜索骨架并拟合直线,最终得到了骨架的形状。
相关推荐
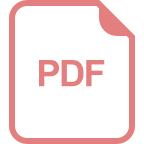












