convert tf.reshape to pytorch
时间: 2023-10-21 12:04:58 浏览: 44
To convert `tf.reshape` to PyTorch, you can use the `view` method in PyTorch. Here's an example:
```python
# TensorFlow code:
import tensorflow as tf
x = tf.constant([[1, 2], [3, 4], [5, 6]])
y = tf.reshape(x, [2, 3])
# PyTorch equivalent:
import torch
x = torch.tensor([[1, 2], [3, 4], [5, 6]])
y = x.view(2, 3)
```
In this example, `x` is a 3x2 tensor, and `y` is the reshaped tensor with shape 2x3. The `view` method in PyTorch works similarly to `tf.reshape` in TensorFlow.
相关问题
tf.reshape 和np.reshape
tf.reshape和np.reshape都是用于改变张量形状的函数。它们的参数都是一个张量和一个目标形状,但是在使用上有一些不同。tf.reshape是TensorFlow中的函数,用于改变张量的形状,它的参数是一个张量和一个目标形状,返回一个新的张量。而np.reshape是NumPy中的函数,也用于改变数组的形状,它的参数也是一个数组和一个目标形状,返回一个新的数组。两者的功能类似,但是在使用上有一些不同,例如在处理GPU上的张量时,tf.reshape比np.reshape更高效。此外,tf.reshape还可以在计算图中进行自动微分,而np.reshape则不能。
tf.reshape
`tf.reshape` 是 TensorFlow 中用于改变张量形状的函数。它可以将一个张量转换成指定的形状,而不改变张量的数据。它的语法如下:
```python
tf.reshape(tensor, shape, name=None)
```
其中,`tensor` 参数是需要改变形状的张量,`shape` 参数是目标形状,可以是一个列表或元组。如果 `shape` 中某个元素是 `-1`,则表示这一维的大小由函数自动计算得出,以保证张量元素总数不变。
例如,将一个形状为 `(2, 3, 4)` 的张量转换成形状为 `(6, 4)` 的张量可以这样实现:
```python
import tensorflow as tf
x = tf.ones((2, 3, 4))
y = tf.reshape(x, (6, 4))
print(y.shape) # 输出 (6, 4)
```
需要注意的是,`tf.reshape` 函数返回的是一个新的张量,原张量并不会被修改。如果在 `shape` 中指定的形状与原张量的元素总数不同,则会抛出异常。
相关推荐
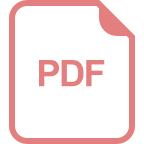
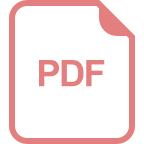
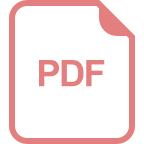












