Ridge.fit() got an unexpected keyword argument 'feature_names'
时间: 2023-11-11 11:42:00 浏览: 30
The error message you are seeing suggests that the `fit()` method in your Ridge regression model is not recognizing the `feature_names` argument that you are using. This may be because the `feature_names` argument is not a valid argument for the `fit()` method of the Ridge regression class.
To resolve this issue, you can try removing the `feature_names` argument from the `fit()` method call. Alternatively, you can try using a different regression model that supports the `feature_names` argument, or you can modify the code of the Ridge regression class to support the `feature_names` argument.
相关问题
from sklearn.linear_model import Ridge ridge = Ridge(alpha=1.0) ridge.fit(X_train[features_without_ones],y_train) w_ridge = [] w_ridge.extend(ridge.coef_) w_ridge.append(ridge.intercept_) w1["ridge_sklearn_w"] = w_ridge w1.round(decimals=2)
这段代码使用了scikit-learn库中的Ridge回归模型来拟合特征矩阵X_train和目标变量y_train,并将得到的系数和截距存储在列表w_ridge中。最后,将系数和截距放入字典w1中,键为"ridge_sklearn_w"。函数round(decimals=2)是将结果四舍五入到小数点后两位。Ridge回归是一种线性回归模型,其中加入了L2正则化项,可以用来处理多重共线性问题。
Ridge_reg.fit什么意思
Ridge_reg.fit是一个方法,通常用于线性回归中的岭回归(Ridge regression)模型。它用于拟合(fit)训练数据,并训练模型以适应数据的特征和目标变量。岭回归是一种用于处理多重共线性问题的回归分析技术,它通过加入一个正则化项(L2范数)来限制模型的复杂度,防止过拟合。
具体来说,Ridge_reg.fit方法会根据给定的训练数据和目标变量来调整模型的参数,以最小化预测值与实际值之间的差异。在拟合过程中,模型会尝试找到最佳的权重参数,以使损失函数(通常是均方误差)最小化。这样,我们就可以使用训练好的模型来进行预测和推断。
相关推荐
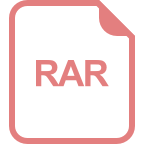
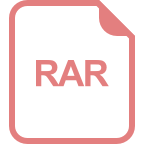












