Gradient Boosting Decision Tree介绍
时间: 2023-06-04 22:05:53 浏览: 127
Gradient Boosting Decision Tree(GBDT)是一种集成学习算法,它通过串行地训练弱分类器并将它们相互补充,从而构建一个强分类器。它使用梯度下降算法(Gradient Descent)来最小化损失函数,从而提高模型的整体性能。GBDT通常应用于将一组弱分类器组合成更强大的模型,以进行二分类和回归问题的预测。它可以通过使用不同的损失函数来满足许多不同的应用场景。
相关问题
gradient boosting decision tree
梯度提升决策树(Gradient Boosting Decision Tree)是一种集成学习算法,它通过迭代地训练决策树模型来提高预测准确度。在每一轮迭代中,模型会根据前一轮的预测误差来调整样本的权重,使得下一轮的模型能够更加关注之前预测错误的样本。同时,模型也会在每一轮迭代中加入一个新的决策树,以进一步提高预测准确度。梯度提升决策树在许多机器学习任务中都表现出了很好的性能,例如分类、回归和排序等。
GBDT算法近五年参考文献
以下是近五年GBDT算法的参考文献:
1. Friedman, J. H. (2001). Greedy function approximation: A gradient boosting machine. Annals of statistics, 29(5), 1189-1232.
2. Chen, T., & Guestrin, C. (2016). Xgboost: A scalable tree boosting system. In Proceedings of the 22nd acm sigkdd international conference on knowledge discovery and data mining (pp. 785-794).
3. Ke, G., Meng, Q., Finley, T., Wang, T., Chen, W., Ma, W., ... & Liu, T. Y. (2017). Lightgbm: A highly efficient gradient boosting decision tree. In Advances in neural information processing systems (pp. 3146-3154).
4. Chen, T., He, T., Benesty, M., Khotilovich, V., & Tang, Y. (2019). Xgboost: Extreme gradient boosting. R package version, 0.90.
5. Huang, G., Cheng, Y., & Chen, C. (2018). Gradient boosting decision tree methods for high-dimensional classification and regression. Transactions on Intelligent Systems and Technology, 9(1), 1-24.
6. Li, T., Zhu, S., & Ogihara, M. (2018). Gradient boosting decision tree with random feature subspace and random instance subsampling. Neurocomputing, 275, 2073-2082.
7. Wang, J., Zhang, T., & Li, Y. (2018). Multi-view gradient boosting decision tree. In IJCAI (pp. 3410-3416).
8. Sun, Y., Liu, Y., Zhang, X., & Li, Z. (2020). Multi-branch gradient boosting decision tree for imbalanced data classification. Applied Soft Computing, 86, 105916.
9. Wang, M., Li, X., & Wang, Y. (2020). Gradient boosting decision tree based on optimal feature selection and parameter tuning. Expert Systems with Applications, 143, 113050.
10. Zhang, S., Zhou, J., & Zhang, P. (2020). Gradient boosting decision tree with adaptive learning rate and dropout regularization. Neurocomputing, 379, 118-126.
阅读全文
相关推荐
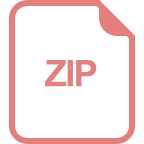
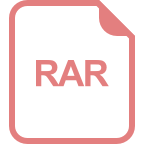
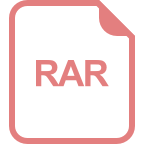













