翻译:class Vehicle(): def __init__(self): self.depot_id=0 self.x_coord=0 self.y_coord=0 self.type=0 self.capacity=0 self.free_speed=1 self.fixed_cost=1.0 self.variable_cost=1.0 self.numbers=0 self.start_time=0 self.end_time=1440
时间: 2024-04-28 21:25:24 浏览: 150
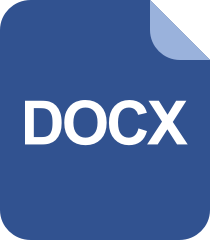
ESXi5.X安装DELL_Equallogic多路径软件.docx
这段代码定义了一个类 `Vehicle`,并且在初始化函数 `__init__()` 中定义了该类的属性,包括 `depot_id`、`x_coord`、`y_coord`、`type`、`capacity`、`free_speed`、`fixed_cost`、`variable_cost`、`numbers`、`start_time` 和 `end_time`。这些属性可以在类的实例化过程中赋值,用于描述车辆的特征和限制条件。
阅读全文
相关推荐
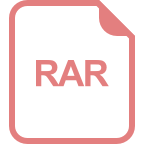
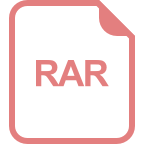









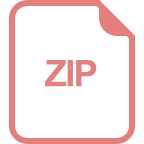






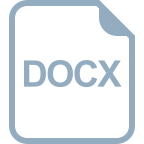