softmax损失函数代码实现
时间: 2024-06-01 07:07:15 浏览: 15
softmax损失函数是一种用于多分类问题的损失函数,它可以将多个类别的输出概率归一化并计算交叉熵损失。在代码实现中,我们需要对每个样本的输出概率进行归一化,并计算它们与对应标签的交叉熵损失。
以下是softmax损失函数的代码实现示例:
```python
import numpy as np
def softmax_loss(scores, y):
# scores为模型对每个类别的输出概率
# y为对应的标签
num_samples = scores.shape
exp_scores = np.exp(scores)
probs = exp_scores / np.sum(exp_scores, axis=1, keepdims=True)
loss = -np.log(probs[range(num_samples), y])
data_loss = np.sum(loss) / num_samples
return data_loss
# 示例
scores = np.array([[1.2, 0.9, 0.3], [0.8, 1.5, 0.6], [0.2, 0.4, 1.1]])
y = np.array([0, 1, 2])
loss = softmax_loss(scores, y)
print(loss)
```
相关问题
L-softmax损失函数 代码
L-softmax(Large Margin Softmax)损失函数是一种用于增强分类器边界的损失函数。它通过在原始的softmax损失函数上引入一个额外的角度约束,使得不同类别之间的边界更加明确。下面是一个示例代码,展示了如何在PyTorch框架中实现L-softmax损失函数:
```python
import torch
import torch.nn as nn
class LSoftmaxLoss(nn.Module):
def __init__(self, num_classes, margin):
super(LSoftmaxLoss, self).__init__()
self.num_classes = num_classes
self.margin = margin
self.theta = nn.Parameter(torch.FloatTensor(num_classes, num_classes-1))
nn.init.kaiming_uniform_(self.theta)
def forward(self, input, target):
batch_size = input.size(0)
input_norm = torch.norm(input, p=2, dim=1, keepdim=True)
input_normalized = input.div(input_norm.expand_as(input))
target_onehot = torch.zeros(batch_size, self.num_classes).to(input.device)
target_onehot.scatter_(1, target.view(-1, 1), 1)
target_onehot.sub_(input_normalized * (1 - self.margin))
output = input_normalized.mm(self.theta)
loss = nn.CrossEntropyLoss()(output, target)
return loss
```
使用时,你可以将这个LSoftmaxLoss类作为损失函数使用,例如:
```python
num_classes = 10
margin = 4.0
loss_fn = LSoftmaxLoss(num_classes, margin)
output = model(input)
loss = loss_fn(output, target)
loss.backward()
```
在这个示例中,`num_classes`表示类别的数量,`margin`表示额外的角度约束。你可以根据你的实际应用进行调整。希望这个代码能够帮助到你!
softmax函数回归代码实现
以下是一个使用Python实现softmax函数回归的代码示例:
```python
import numpy as np
def softmax(x):
exp_x = np.exp(x)
return exp_x / np.sum(exp_x)
def softmax_regression(X, y, num_classes, num_iterations, learning_rate):
num_features = X.shape[1]
weights = np.zeros((num_features, num_classes))
for i in range(num_iterations):
for j in range(X.shape[0]):
x = X[j]
y_true = y[j]
y_pred = softmax(np.dot(x, weights))
error = y_pred - (y_true == np.arange(num_classes))
gradient = np.outer(x, error)
weights -= learning_rate * gradient
return weights
# Example usage
X = np.array([[1, 2, 3], [4, 5, 6], [7, 8, 9]])
y = np.array([0, 1, 2])
num_classes = 3
num_iterations = 1000
learning_rate = 0.1
weights = softmax_regression(X, y, num_classes, num_iterations, learning_rate)
print(weights)
```
这个代码实现了一个简单的softmax函数回归模型,用于多分类问题。给定一个输入向量x,模型通过将x与权重矩阵相乘并应用softmax函数来预测每个类别的概率。在训练过程中,模型使用梯度下降算法来最小化交叉熵损失函数。
相关推荐
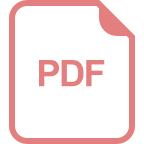
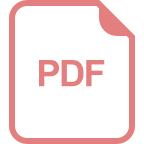
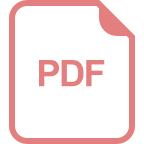












