在MLP中导入Transformer模块
时间: 2023-11-14 17:46:50 浏览: 69
好的,这是一个关于机器学习的技术问题,我可以回答。在MLP中导入Transformer模块可以帮助提升神经网络的性能和准确性。Transformer是一种基于自注意力机制的神经网络模型,它被成功应用于自然语言处理领域,如机器翻译。通过将Transformer模块导入MLP中,可以使得神经网络更好地捕捉输入数据中的长程依赖关系,进而提升模型的性能。
相关问题
我想在swin-transformer中添加se注意力模块 请给出代码
好的,为了在Swin Transformer中添加SE注意力模块,您需要进行以下步骤:
1. 首先,在您的代码中导入SE注意力模块所需要的依赖项:
```
import torch
import torch.nn as nn
import torch.nn.functional as F
```
2. 接着定义SE注意力模块的代码如下:
```
class SEAttention(nn.Module):
def __init__(self, in_channels, ratio=16):
super(SEAttention, self).__init__()
self.avg_pool = nn.AdaptiveAvgPool2d(1)
self.fc = nn.Sequential(
nn.Linear(in_channels, in_channels // ratio),
nn.ReLU(inplace=True),
nn.Linear(in_channels // ratio, in_channels),
nn.Sigmoid()
)
def forward(self, x):
b, c, _, _ = x.size()
y = self.avg_pool(x).view(b, c)
y = self.fc(y).view(b, c, 1, 1)
return x * y.expand_as(x)
```
3. 最后,在Swin Transformer中添加SE注意力模块的代码如下:
```
class SwinTransformerBlock(nn.Module):
def __init__(self, embed_dim, num_heads, mlp_ratio=4., qkv_bias=False, qk_scale=None, drop=0., attn_drop=0., drop_path=0., act_layer=nn.GELU, norm_layer=nn.LayerNorm, se_ratio=0.):
super().__init__()
self.norm1 = norm_layer(embed_dim)
self.attn = Attention(
embed_dim, num_heads=num_heads, qkv_bias=qkv_bias, qk_scale=qk_scale, attn_drop=attn_drop, proj_drop=drop)
self.drop_path = DropPath(drop_path) if drop_path > 0. else nn.Identity()
self.norm2 = norm_layer(embed_dim)
mlp_hidden_dim = int(embed_dim * mlp_ratio)
self.mlp = Mlp(in_features=embed_dim, hidden_features=mlp_hidden_dim, act_layer=act_layer, drop=drop)
if se_ratio > 0.:
self.se = SEAttention(embed_dim, int(embed_dim * se_ratio))
else:
self.se = nn.Identity()
def forward(self, x):
x = x + self.drop_path(self.attn(self.norm1(x)))
x = x + self.drop_path(self.mlp(self.norm2(x)))
x = self.se(x)
return x
```
在这个代码中,我们在Swin Transformer Block中添加了一个SEAttention模块,并通过参数`se_ratio`控制SE注意力模块的比例。如果您想要使用SE注意力模块,请将`se_ratio`设置为一个大于0的值,否则将其设置为0即可。
希望这能帮助到您!
请问我怎么在swin transformer中添加注意力机制
在Swin Transformer中添加注意力机制需要进行以下步骤:
1. 导入需要的模块
在Swin Transformer的代码中,需要导入以下模块:
``` python
import torch
from torch import nn
from torch.nn import functional as F
```
2. 实现注意力机制
在Swin Transformer中,可以通过实现自定义的注意力机制来添加注意力机制。
``` python
class Attention(nn.Module):
def __init__(self, in_channels, out_channels, kernel_size=1, stride=1):
super(Attention, self).__init__()
self.conv = nn.Conv2d(in_channels, out_channels, kernel_size, stride, padding=kernel_size // 2, groups=out_channels)
self.norm = nn.BatchNorm2d(out_channels)
self.act = nn.ReLU(inplace=True)
self.pool = nn.AdaptiveAvgPool2d(1)
self.conv_atten = nn.Conv2d(out_channels, out_channels, kernel_size=1, stride=1)
self.sigmoid = nn.Sigmoid()
def forward(self, x):
x = self.conv(x)
x = self.norm(x)
x = self.act(x)
x = self.pool(x)
x = self.conv_atten(x)
x = self.sigmoid(x)
return x
```
在这个自定义的注意力模块中,使用了卷积、BN、ReLU、全局平均池化、卷积、Sigmoid等操作,来实现对输入特征图的注意力加权。
3. 在Swin Transformer中使用注意力机制
在Swin Transformer中,可以在需要添加注意力机制的地方,将Attention模块加入到网络中。
例如,在Swin Transformer的基础块中,可以在第二个分支的卷积之前添加注意力模块:
``` python
class SwinTransformerBlock(nn.Module):
def __init__(self, dim, input_resolution, num_heads, window_size=7, shift_size=0,
mlp_ratio=4., qkv_bias=False, qk_scale=None, drop=0., attn_drop=0.,
drop_path=0., act_layer=nn.ReLU, norm_layer=nn.LayerNorm):
super().__init__()
self.dim = dim
self.input_resolution = input_resolution
self.num_heads = num_heads
self.window_size = window_size
self.shift_size = shift_size
self.mlp_ratio = mlp_ratio
self.qkv_bias = qkv_bias
self.qk_scale = qk_scale
self.drop = drop
self.attn_drop = attn_drop
self.drop_path = drop_path
self.norm1_name, norm2_name = norm_layer.__name__.split('.')[-1], norm_layer.__name__.split('.')[-1]
self.norm1 = norm_layer(dim)
self.attn = WindowAttention(
dim, window_size=window_size, num_heads=num_heads, qkv_bias=qkv_bias, qk_scale=qk_scale, attn_drop=attn_drop, proj_drop=drop)
self.norm2 = norm_layer(dim)
self.mlp = Mlp(in_features=dim, hidden_features=int(dim * mlp_ratio), act_layer=act_layer, drop=drop)
self.conv_atten = Attention(dim, dim//8) # 添加注意力模块
def forward(self, x, mask_matrix=None):
H, W = self.input_resolution
B, L, C = x.shape
assert L == H * W, "input feature has wrong size"
# norm before attn
x = self.norm1(x)
# calculate attention mask
if mask_matrix is None:
mask_matrix = torch.zeros((1, H, W, H, W), dtype=x.dtype, device=x.device) # 生成全零的mask矩阵
if self.window_size == H and self.shift_size == 0:
# use global attention
attn_mask = mask_matrix
else:
# calculate attention mask for SW-MSA
attn_mask = self.calculate_mask(mask_matrix)
# atention
x = x.reshape(B, H, W, C).permute(0, 3, 1, 2)
# 添加注意力模块
x = x * self.conv_atten(x)
x = x.permute(0, 2, 3, 1).reshape(B, H * W, C)
x, attn = self.attn(x, attn_mask)
# drop path
if self.drop_path > 0.:
x = drop_path(x, self.drop_path, self.training)
# reesidual connection
x = x + self.drop_path(self.mlp(self.norm2(x)), self.drop_path, self.training)
return x, attn, mask_matrix
```
在这个Swin Transformer基础块的第二个分支的卷积之前,加入了Attention模块,并用该模块对输入特征图进行了注意力加权。
阅读全文
相关推荐
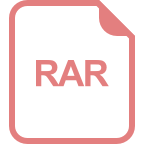
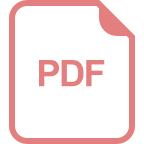
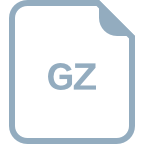
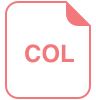
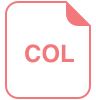
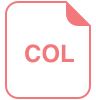
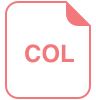
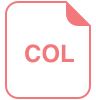







