training samples
时间: 2023-08-29 14:08:08 浏览: 38
训练样本是指用于训练机器学习模型的输入数据和相应的输出结果。在深度学习中,训练样本通常是大量的数据,用于训练神经网络模型。这些数据包括输入数据和相应的标签或答案。训练样本的质量和数量对于机器学习模型的性能和准确性至关重要。通常,更多和更高质量的训练样本会导致更好的模型性能。在某些情况下,数据的质量比数量更为重要,例如,对于医疗图像或金融数据等敏感领域,质量是至关重要的。为了获得高质量的训练数据,通常需要进行数据清洗、预处理和标注等工作。
相关问题
Given a dataset with only two training samples and . Suppose we want to build a Linear Regression model with learning rate . At first, and . 1)Please calculate the cost . 2)Update the parameters and for one time. 3)Please calculate the new cost after updating.
Given:
- Two training samples: `(2, 3)` and `(4, 5)`
- Initial parameters: `theta0 = 0, theta1 = 0`
- Learning rate: `alpha = 0.01`
To build a Linear Regression model, we need to minimize the cost function. The cost function for Linear Regression is:
```
J(theta0, theta1) = (1/2m) * sum(i=1 to m)[hypothesis(xi) - yi]^2
```
where `m` is the total number of training samples, `xi` is the feature of the `i`th training sample, `yi` is the label of the `i`th training sample, and `hypothesis(xi)` is the predicted value for `xi` using the current values of `theta0` and `theta1`.
1) Calculate the initial cost:
```
hypothesis(2) = theta0 + theta1 * 2 = 0 + 0 * 2 = 0
hypothesis(4) = theta0 + theta1 * 4 = 0 + 0 * 4 = 0
J(theta0, theta1) = (1/4) * [(0 - 3)^2 + (0 - 5)^2] = 13/4 = 3.25
```
So the initial cost is `3.25`.
2) Update the parameters for one time:
```
theta0 = theta0 - alpha * (1/m) * sum(i=1 to m)[hypothesis(xi) - yi] = 0 - 0.01 * (1/2) * [(0 - 3) + (0 - 5)] = 0.04
theta1 = theta1 - alpha * (1/m) * sum(i=1 to m)[(hypothesis(xi) - yi) * xi] = 0 - 0.01 * (1/2) * [(0 - 3) * 2 + (0 - 5) * 4] = 0.11
```
So the updated parameters are `theta0 = 0.04` and `theta1 = 0.11`.
3) Calculate the new cost after updating:
```
hypothesis(2) = theta0 + theta1 * 2 = 0.04 + 0.11 * 2 = 0.26
hypothesis(4) = theta0 + theta1 * 4 = 0.04 + 0.11 * 4 = 0.46
J(theta0, theta1) = (1/4) * [(0.26 - 3)^2 + (0.46 - 5)^2] = 2.69/4 = 0.6725
```
So the new cost after updating is `0.6725`.
decision_function is not available when novelty=False. Use novelty=True if you want to use LOF for novelty detection and compute decision_function for new unseen data. Note that the opposite LOF of the training samples is always available by considering the negative_outlier_factor_ attribute.怎么解决python
这个错误通常发生在你使用 scikit-learn 的 LocalOutlierFactor 模型时,尝试在 novelty=False 的情况下调用 decision_function() 方法时。
LocalOutlierFactor 模型的 decision_function() 方法只适用于 novelty=True 的情况,即用于新的未见过的数据的离群值检测。如果 novelty=False,表示你只是在对已有的数据进行离群值检测,那么就不能使用 decision_function() 方法。
如果你确实需要在 novelty=False 的情况下使用 decision_function() 方法,你可以将模型的 novelty 参数设置为 True,例如:
```python
from sklearn.neighbors import LocalOutlierFactor
clf = LocalOutlierFactor(novelty=True)
clf.fit(X_train)
scores = clf.decision_function(X_test)
```
这样就可以使用 decision_function() 方法来计算测试数据的离群得分了。需要注意的是,这样做会将模型用于新的未见过的数据,而不是仅用于已有的数据。另外,训练数据的相反LOF值始终可通过考虑 negative_outlier_factor_ 属性获得。
相关推荐
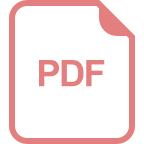
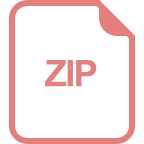












