r_vector.matrix()解释
时间: 2024-05-24 12:13:05 浏览: 110
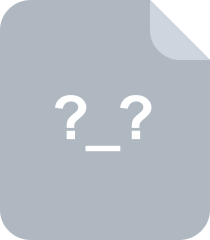
Vector_matrix
r_vector.matrix()是R语言中的一个函数,它将一个向量转换为一个矩阵。该函数的使用格式为:
r_vector.matrix(x, nrow = NULL, ncol = NULL, byrow = FALSE)
参数说明:
- x:要转换为矩阵的向量。
- nrow:矩阵的行数。
- ncol:矩阵的列数。
- byrow:逻辑值,表示矩阵的填充方式。默认为FALSE,按列填充;若为TRUE,则按行填充。
如果nrow和ncol都是NULL,则矩阵的行数和列数将根据向量的长度自动确定。如果只指定了其中一个参数,则另一个参数将自动计算。
示例:
# 创建一个向量
my_vector <- c(1, 2, 3, 4, 5, 6)
# 将向量转换为矩阵
my_matrix <- r_vector.matrix(my_vector, nrow = 2)
# 输出矩阵
my_matrix
# [,1] [,2] [,3]
# [1,] 1 3 5
# [2,] 2 4 6
在上面的示例中,将一个长度为6的向量转换为一个2行3列的矩阵。由于没有指定填充方式,默认按列填充。结果显示一个2x3的矩阵。
阅读全文
相关推荐
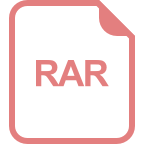
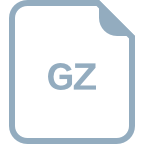


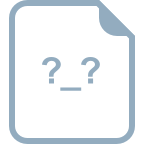








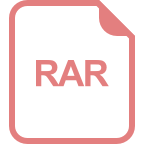