assert input.is_cuda, "NVFuser InstanceNorm is CUDA only"
时间: 2024-04-13 16:25:28 浏览: 14
该断言语句的作用是检查输入张量是否在CUDA上运行。如果输入张量不在CUDA上运行,将引发一个异常,并显示给定的错误消息。
在深度学习中,通常使用CUDA来加速模型的训练和推理过程。NVFuser是一种特定的实例归一化(Instance Normalization)操作,它要求输入张量在CUDA上运行。如果输入张量不满足这个条件,可能会导致错误的结果或不可预测的行为。
因此,该断言语句用于确保输入张量符合NVFuser InstanceNorm操作的要求。如果输入张量未在CUDA上运行,该断言将触发异常并显示错误消息"NVFuser InstanceNorm is CUDA only"。这样可以帮助开发者及时发现问题并进行修复。
相关问题
Focal 损失函数代码如下:def focal_loss(input_values, gamma): """Computes the focal loss""" p = torch.exp(-input_values) loss = (1 - p) ** gamma * input_values return loss.mean() class FocalLoss(nn.Module): def __init__(self, weight=None, gamma=0.): super(FocalLoss, self).__init__() assert gamma >= 0 self.gamma = gamma self.weight = weight def forward(self, input, target): return focal_loss(F.cross_entropy(input, target, reduction='none', weight=self.weight), self.gamma) LDAM损失函数代码如下:class LDAMLoss(nn.Module): def __init__(self, cls_num_list, max_m=0.5, weight=None, s=30): super(LDAMLoss, self).__init__() m_list = 1.0 / np.sqrt(np.sqrt(cls_num_list)) m_list = m_list * (max_m / np.max(m_list)) m_list = torch.cuda.FloatTensor(m_list) self.m_list = m_list assert s > 0 self.s = s self.weight = weight def forward(self, x, target): index = torch.zeros_like(x, dtype=torch.uint8) index.scatter_(1, target.data.view(-1, 1), 1) index_float = index.type(torch.cuda.FloatTensor) batch_m = torch.matmul(self.m_list[None, :], index_float.transpose(0,1)) batch_m = batch_m.view((-1, 1)) x_m = x - batch_m output = torch.where(index, x_m, x) return F.cross_entropy(self.s*output, target, weight=self.weight) Large Margin aware Focal (LMF) 损失函数是 Focal 损失函数和 LDAM损失函数加权的线性组合,帮我用pytorch代码实现LMF损失函数
以下是LMF损失函数的PyTorch实现:
```
class LMF_Loss(nn.Module):
def __init__(self, cls_num_list, max_m=0.5, weight=None, s=30, gamma=2.0, alpha=0.25):
super(LMF_Loss, self).__init__()
self.gamma = gamma
self.alpha = alpha
self.focal_loss = FocalLoss(weight=weight, gamma=gamma)
self.ldam_loss = LDAMLoss(cls_num_list, max_m=max_m, weight=weight, s=s)
def forward(self, x, target):
focal_loss = self.focal_loss(x, target)
ldam_loss = self.ldam_loss(x, target)
lmf_loss = self.alpha * focal_loss + (1 - self.alpha) * ldam_loss.pow(self.gamma)
return lmf_loss.mean()
```
其中,`gamma`为Focal Loss的超参数,`alpha`为LMF Loss中Focal Loss的权重。其他参数含义与原始的Focal Loss和LDAM Loss相同。
pytorch部分代码如下:train_loss, train_acc = train(model_ft, DEVICE, train_loader, optimizer, epoch,model_ema) if use_amp: with torch.cuda.amp.autocast(): # 开启混合精度 loss = torch.nan_to_num(criterion_train(output, targets)) # 计算loss scaler.scale(loss).backward() # 梯度放大 torch.nn.utils.clip_grad_norm_(model.parameters(), CLIP_GRAD) if not (self._backward_hooks or self._forward_hooks or self._forward_pre_hooks or _global_backward_hooks or global_forward_hooks or global_forward_pre_hooks): return forward_call(*input, **kwargs) class LDAMLoss(nn.Module): def init(self, cls_num_list, max_m=0.5, weight=None, s=30): super(LDAMLoss, self).init() m_list = 1.0 / np.sqrt(np.sqrt(cls_num_list)) m_list = m_list * (max_m / np.max(m_list)) m_list = torch.cuda.FloatTensor(m_list) self.m_list = m_list assert s > 0 self.s = s self.weight = weight def forward(self, x, target): index = torch.zeros_like(x, dtype=torch.uint8) index.scatter(1, target.data.view(-1, 1).type(torch.int64), 1) index_float = index.type(torch.cuda.FloatTensor) batch_m = torch.matmul(self.m_list[None, :], index_float.transpose(0,1)) batch_m = batch_m.view((-1, 1)) x_m = x - batch_m output = torch.where(index, x_m, x) return F.cross_entropy(self.s*output, target, weight=self.weight) 报错:RuntimeError: Expected index [112, 1] to be smaller than self [16, 7] apart from dimension 1 帮我看看如何修改源代码
根据你提供的代码,报错信息显示 index 的形状是 [112, 1],而 x 的形状是 [16, 7],因此 index 与 x 的形状不匹配,导致了这个错误。
为了解决这个问题,你可以尝试将 LDAMLoss 类的 forward 函数中的 index 的形状改为 [x.size(0), x.size(1)],这样就能够与 x 的形状匹配。同时,由于 target.data 已经被弃用,建议改为 target。修改后的代码如下:
```
class LDAMLoss(nn.Module):
def __init__(self, cls_num_list, max_m=0.5, weight=None, s=30):
super(LDAMLoss, self).__init__()
m_list = 1.0 / np.sqrt(np.sqrt(cls_num_list))
m_list = m_list * (max_m / np.max(m_list))
m_list = torch.cuda.FloatTensor(m_list)
self.m_list = m_list
assert s > 0
self.s = s
self.weight = weight
def forward(self, x, target):
index = torch.zeros_like(x, dtype=torch.uint8)
index.scatter_(1, target.view(-1, 1).type(torch.int64), 1)
index_float = index.type(torch.cuda.FloatTensor)
batch_m = torch.matmul(self.m_list[None, :], index_float.transpose(0,1))
batch_m = batch_m.view((-1, 1))
x_m = x - batch_m
output = torch.where(index, x_m, x)
return F.cross_entropy(self.s*output, target, weight=self.weight)
```
另外,你的 init 方法也存在一些错误。super 函数应该在 init 方法的第一行调用,而不是在函数体内。因此,init 方法应该改为:
```
def __init__(self, cls_num_list, max_m=0.5, weight=None, s=30):
super(LDAMLoss, self).__init__()
m_list = 1.0 / np.sqrt(np.sqrt(cls_num_list))
m_list = m_list * (max_m / np.max(m_list))
m_list = torch.cuda.FloatTensor(m_list)
self.m_list = m_list
assert s > 0
self.s = s
self.weight = weight
```
相关推荐










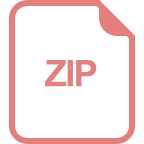
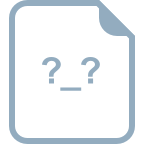
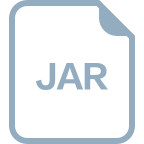