nn.Conv2d group
时间: 2023-11-23 07:51:26 浏览: 80
nn.Conv2d中的group参数用于指定卷积操作的分组数量。在卷积操作中,输入和输出都会被分成多个通道组。每个组都有一组滤波器(卷积核),分别应用于对应的通道组。这个参数主要用于将卷积操作分解为多个独立的、较小的卷积操作,以降低计算复杂度。
分组卷积适用于一些特殊的网络结构设计,例如Inception模块中的分支卷积。通过将卷积操作拆分为多个组,可以减少计算量,并且可以提高模型的表示能力。
在nn.Conv2d中,group参数默认值为1,表示不使用分组卷积,即将输入和输出视为单个通道组。如果将group设置为输入通道数和输出通道数之间的一个因子,则会进行分组卷积操作。
相关问题
def init(self, in_channel, out_channel, stride=1, downsample=None, groups=1, width_per_group=64): super(Bottleneck, self).init() width = int(out_channel * (width_per_group / 64.)) * groups self.conv1 = nn.Conv2d(in_channels=in_channel, out_channels=width, kernel_size=1, stride=1, bias=False) # squeeze channels self.bn1 = nn.BatchNorm2d(width) self.conv2 = nn.Conv2d(in_channels=width, out_channels=width, groups=groups, kernel_size=3, stride=stride, bias=False, padding=1) self.bn2 = nn.BatchNorm2d(width) self.conv3 = nn.Conv2d(in_channels=width, out_channels=out_channelself.expansion, kernel_size=1, stride=1, bias=False) # unsqueeze channels self.bn3 = nn.BatchNorm2d(out_channelself.expansion) self.relu = nn.ReLU(inplace=True) self.downsample = downsample
这段代码是一个 Bottleneck 模块的初始化函数。Bottleneck 模块是 ResNet 中的一种基本模块,在卷积神经网络中用于增加网络的深度和宽度。该模块包含三个卷积层,其中第一个和第三个卷积层是 1x1 卷积层,用于减少和增加通道数,中间的卷积层是 3x3 的卷积层,用于增加模块的感受野。该模块还包含 Batch Normalization 和 ReLU 激活函数。其中,参数 in_channel 和 out_channel 分别指输入和输出的通道数,stride 指卷积的步长,downsample 指是否需要下采样以匹配输入和输出的通道数,groups 和 width_per_group 用于分组卷积。该模块的输出通道数是 out_channel*self.expansion,其中 self.expansion 是一个常量,用于保持输入和输出通道数的一致性。需要注意的是,该段代码的 init 函数应该是 __init__ 函数的拼写错误,应该修改为 __init__。
def __init__(self, in_channel, out_channel, stride=1, downsample=None, groups=1, width_per_group=64): super(Bottleneck, self).__init__() width = int(out_channel * (width_per_group / 64.)) * groups self.conv1 = nn.Conv2d(in_channels=in_channel, out_channels=width, kernel_size=1, stride=1, bias=False) # squeeze channels self.bn1 = nn.BatchNorm2d(width) # ----------------------------------------- self.conv2 = nn.Conv2d(in_channels=width, out_channels=width, groups=groups, kernel_size=3, stride=stride, bias=False, padding=1) self.bn2 = nn.BatchNorm2d(width) # ----------------------------------------- self.conv3 = nn.Conv2d(in_channels=width, out_channels=out_channel*self.expansion, kernel_size=1, stride=1, bias=False) # unsqueeze channels self.bn3 = nn.BatchNorm2d(out_channel*self.expansion) self.relu = nn.ReLU(inplace=True) self.downsample = downsample
这段代码是一个 Bottleneck 模块的初始化函数。Bottleneck 模块是 ResNet 中的一种基本模块,在卷积神经网络中用于增加网络的深度和宽度。该模块包含三个卷积层,其中第一个和第三个卷积层是 1x1 卷积层,用于减少和增加通道数,中间的卷积层是 3x3 的卷积层,用于增加模块的感受野。该模块还包含 Batch Normalization 和 ReLU 激活函数。其中,参数 in_channel 和 out_channel 分别指输入和输出的通道数,stride 指卷积的步长,downsample 指是否需要下采样以匹配输入和输出的通道数,groups 和 width_per_group 用于分组卷积。该模块的输出通道数是 out_channel*self.expansion,其中 self.expansion 是一个常量,用于保持输入和输出通道数的一致性。
阅读全文
相关推荐
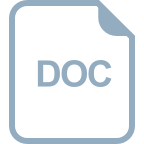
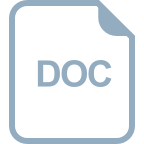
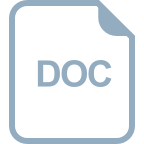













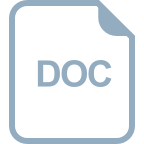