def edge_attention(self, edges): # an edge UDF to compute unnormalized attention values from src and dst if self.l0 == 0: m = self.leaky_relu(edges.src['a1'] + edges.dst['a2']) else: tmp = edges.src['a1'] + edges.dst['a2'] logits = tmp + self.bias_l0 if self.training: m = l0_train(logits, 0, 1) else: m = l0_test(logits, 0, 1) self.loss = get_loss2(logits[:,0,:]).sum() return {'a': m}
时间: 2024-02-14 13:30:47 浏览: 61
在这段代码中,`edge_attention`函数是一个图神经网络中的边自定义函数。它接收一个包含边信息的edges对象作为输入,并计算未归一化的注意力值。
首先,如果`self.l0`等于0,那么将执行以下操作:
- 计算`edges.src['a1']`和`edges.dst['a2']`的和。
- 使用`self.leaky_relu`函数对和进行激活函数处理,得到注意力值`m`。
接下来,如果`self.l0`不等于0,那么将执行以下操作:
- 计算`edges.src['a1']`和`edges.dst['a2']`的和,存储在变量`tmp`中。
- 将`tmp`与`self.bias_l0`相加,得到logits(对数概率)。
- 如果处于训练模式,则调用`l0_train`函数,传入logits、0和1作为参数,得到一个掩码(mask)`m`。同时,计算损失函数`self.loss`,使用`get_loss2`函数计算logits的损失,并对第一个维度求和。
- 如果处于测试模式,则调用`l0_test`函数,传入logits、0和1作为参数,得到一个掩码(mask)`m`。
最后,函数返回一个包含注意力值的字典,键为'a',值为掩码(mask)`m`。
相关问题
校正以下代码的语法错误 def encode_edge(self, mode, node_history, node_history_st, edge_type, neighbors, neighbors_edge_value, first_history_indices, batch_size): max_hl = self.hyperparams['maximum_history_length'] max_neighbors = 0 for neighbor_states in neighbors: max_neighbors = max(max_neighbors, len(neighbor_states)) edge_states_list = list() # list of [#of neighbors, max_ht, state_dim] for i, neighbor_states in enumerate(neighbors): # Get neighbors for timestep in batch if len(neighbor_states) == 0: # There are no neighbors for edge type # TODO necessary? neighbor_state_length = int( np.sum([len(entity_dims) for entity_dims in self.state[edge_type[1]].values()]) ) edge_states_list.append(torch.zeros((1, max_hl + 1, neighbor_state_length), device=self.device)) else: edge_states_list.append(torch.stack(neighbor_states, dim=0).to(self.device)) # if self.hyperparams['edge_state_combine_method'] == 'sum': # Used in Structural-RNN to combine edges as well. op_applied_edge_states_list = list() for neighbors_state in edge_states_list: op_applied_edge_states_list.append(torch.sum(neighbors_state, dim=0))#torch.sum combined_neighbors = torch.stack(op_applied_edge_states_list, dim=0) # 获取combined_neighbors的第一个维度,代表邻接边的总数 combined_neighbors_0 = combined_neighbors.shape[0] # 创建全零矩阵s_next,形状为[batch_size, max_neighbors, combined_neighbors_0] s_next = torch.zeros((batch_size, max_neighbors, combined_neighbors_0), device=self.device) # 为s_next矩阵中每一行赋值为对应的combined_neighbors # for b in range(batch_size): # s_next[b, :len(neighbors[b]), :] = combined_neighbors[first_history_indices[b]] for i in range(batch_size): s_next[0, i, :] = batch_size[:] for i in range(max_neighbors): s_next[1, i, :] = max_neighbors[i, :] for i in range(combined_neighbors.shape[0]): s_next[2, i, :] = combined_neighbors
代码中缺少冒号,不是合法的 Python 代码。正确的代码应该是这样的:
```
def encode_edge(self, mode, node_history, node_history_st, edge_type, neighbors, neighbors_edge_value, first_history_indices, batch_size):
max_hl = self.hyperparams['maximum_history_length']
max_neighbors = 0
for neighbor_states in neighbors:
max_neighbors = max(max_neighbors, len(neighbor_states))
edge_states_list = list()
```
class Node: def __init__(self, num, name, intro): self.num = num self.name = name self.intro = intro self.visited = False class Edge: def __init__(self, fr, to, weight): self.fr = fr self.to = to self.weight = weight class Graph: def __init__(self): self.nodes = [] self.edges = {} def add_node(self, node): self.nodes.append(node) self.edges[node] = [] def add_edge(self, edge): self.edges[edge.fr].append(edge) self.edges[edge.to].append(Edge(edge.to, edge.fr, edge.weight))graph = Graph() with open('data.txt', 'r', encoding='utf-8') as f: for line in f.readlines(): items = line.strip().split(',') num = int(items[0]) name = items[1] intro = items[2] node = Node(num, name, intro) graph.add_node(node) for i in range(3, len(items), 2): neighbor = int(items[i]) weight = int(items[i+1]) edge = Edge(node, graph.nodes[neighbor-1], weight) graph.add_edge(edge) data.txt里怎么写
data.txt 的格式应该是每一行代表一个节点和它的邻居节点以及边的权重,具体格式如下:
节点编号,节点名称,节点介绍,邻居节点1,边的权重1,邻居节点2,边的权重2,...
例如:
1,Node1,This is Node1,2,10,3,15,4,20
2,Node2,This is Node2,1,10,3,5
3,Node3,This is Node3,1,15,2,5,4,8
4,Node4,This is Node4,1,20,3,8
阅读全文
相关推荐
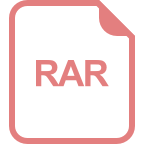
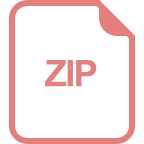
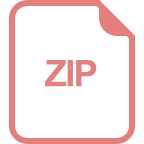













