from mindspore.train.callback import ModelCheckpoint, CheckpointConfig, LossMonitor, TimeMonitor class LossCallBack(LossMonitor): """ Monitor the loss in training. If the loss in NAN or INF terminating training. """ def __init__(self, has_trained_epoch=0, per_print_times=per_print_steps): super(LossCallBack, self).__init__() self.has_trained_epoch = has_trained_epoch self._per_print_times = per_print_times def step_end(self, run_context): cb_params = run_context.original_args() loss = cb_params.net_outputs if isinstance(loss, (tuple, list)): if isinstance(loss[0], ms.Tensor) and isinstance(loss[0].asnumpy(), np.ndarray): loss = loss[0] if isinstance(loss, ms.Tensor) and isinstance(loss.asnumpy(), np.ndarray): loss = np.mean(loss.asnumpy()) cur_step_in_epoch = (cb_params.cur_step_num - 1) % cb_params.batch_num + 1 if isinstance(loss, float) and (np.isnan(loss) or np.isinf(loss)): raise ValueError("epoch: {} step: {}. Invalid loss, terminating training.".format( cb_params.cur_epoch_num, cur_step_in_epoch)) if self._per_print_times != 0 and cb_params.cur_step_num % self._per_print_times == 0: # pylint: disable=line-too-long print("epoch: %s step: %s, loss is %s" % (cb_params.cur_epoch_num + int(self.has_trained_epoch), cur_step_in_epoch, loss), flush=True) time_cb = TimeMonitor(data_size=step_size) loss_cb = LossCallBack(has_trained_epoch=0) cb = [time_cb, loss_cb] ckpt_save_dir = cfg['output_dir'] device_target = context.get_context('device_target') if cfg['save_checkpoint']: config_ck = CheckpointConfig(save_checkpoint_steps=save_ckpt_num*step_size, keep_checkpoint_max=10) # config_ck = CheckpointConfig(save_checkpoint_steps=5*step_size, keep_checkpoint_max=10) ckpt_cb = ModelCheckpoint(prefix="resnet", directory=ckpt_save_dir, config=config_ck) cb += [ckpt_cb]
时间: 2024-04-15 15:26:40 浏览: 23
这段代码定义了一些回调函数,用于在训练过程中监控和保存模型。
首先,定义了一个名为LossCallBack的类,继承自LossMonitor回调类。它重写了step_end方法,在每个训练步骤结束时监控损失值。如果损失值为NaN或INF,将抛出ValueError以终止训练。如果_per_print_times参数不为0且当前步骤数是_per_print_times的倍数,将打印当前的训练损失值。
然后,创建了一个TimeMonitor回调实例和一个LossCallBack回调实例。TimeMonitor用于监控训练时间,LossCallBack用于监控训练损失值。
接着,创建了一个回调列表cb,并将time_cb和loss_cb添加到列表中。同时,获取配置文件中的ckpt_save_dir和device_target。
如果配置文件中的save_checkpoint为True,则创建一个CheckpointConfig实例config_ck,用于配置模型保存的参数(保存间隔、最大保存个数等)。然后,创建一个ModelCheckpoint回调实例ckpt_cb,并将其添加到回调列表cb中。
最后,返回回调列表cb,用于在训练过程中使用。
相关问题
import mindspore.nn as nn import mindspore.ops.operations as P from mindspore import Model from mindspore import Tensor from mindspore import context from mindspore import dataset as ds from mindspore.train.callback import ModelCheckpoint, CheckpointConfig, LossMonitor from mindspore.train.serialization import load_checkpoint, load_param_into_net from mindspore.nn.metrics import Accuracy # Define the ResNet50 model class ResNet50(nn.Cell): def __init__(self, num_classes=10): super(ResNet50, self).__init__() self.resnet50 = nn.ResNet50(num_classes=num_classes) def construct(self, x): x = self.resnet50(x) return x # Load the CIFAR-10 dataset data_home = "/path/to/cifar-10/" train_data = ds.Cifar10Dataset(data_home, num_parallel_workers=8, shuffle=True) test_data = ds.Cifar10Dataset(data_home, num_parallel_workers=8, shuffle=False) # Define the hyperparameters learning_rate = 0.1 momentum = 0.9 epoch_size = 200 batch_size = 32 # Define the optimizer optimizer = nn.Momentum(filter(lambda x: x.requires_grad, resnet50.get_parameters()), learning_rate, momentum) # Define the loss function loss_fn = nn.SoftmaxCrossEntropyWithLogits(sparse=True, reduction='mean') # Define the model net = ResNet50() # Define the model checkpoint config_ck = CheckpointConfig(save_checkpoint_steps=1000, keep_checkpoint_max=10) ckpt_cb = ModelCheckpoint(prefix="resnet50", directory="./checkpoints/", config=config_ck) # Define the training dataset train_data = train_data.batch(batch_size, drop_remainder=True) # Define the testing dataset test_data = test_data.batch(batch_size, drop_remainder=True) # Define the model and train it model = Model(net, loss_fn=loss_fn, optimizer=optimizer, metrics={"Accuracy": Accuracy()}) model.train(epoch_size, train_data, callbacks=[ckpt_cb, LossMonitor()], dataset_sink_mode=True) # Load the trained model and test it param_dict = load_checkpoint("./checkpoints/resnet50-200_1000.ckpt") load_param_into_net(net, param_dict) model = Model(net, loss_fn=loss_fn, metrics={"Accuracy": Accuracy()}) result = model.eval(test_data) print("Accuracy: ", result["Accuracy"])这段代码有错误
这段代码确实有一些问题。以下是修复后的代码:
```
import mindspore.nn as nn
import mindspore.ops.operations as P
from mindspore import Model
from mindspore import Tensor
from mindspore import context
from mindspore import dataset as ds
from mindspore.train.callback import ModelCheckpoint, CheckpointConfig, LossMonitor
from mindspore.train.serialization import load_checkpoint, load_param_into_net
from mindspore.nn.metrics import Accuracy
# Define the ResNet50 model
class ResNet50(nn.Cell):
def __init__(self, num_classes=10):
super(ResNet50, self).__init__()
self.resnet50 = nn.ResNet50(num_classes=num_classes)
def construct(self, x):
x = self.resnet50(x)
return x
# Load the CIFAR-10 dataset
data_home = "/path/to/cifar-10/"
train_data = ds.Cifar10Dataset(data_home, num_parallel_workers=8, shuffle=True)
test_data = ds.Cifar10Dataset(data_home, num_parallel_workers=8, shuffle=False)
# Define the hyperparameters
learning_rate = 0.1
momentum = 0.9
epoch_size = 200
batch_size = 32
# Define the model
net = ResNet50()
# Define the optimizer
optimizer = nn.Momentum(filter(lambda x: x.requires_grad, net.get_parameters()), learning_rate, momentum)
# Define the loss function
loss_fn = nn.SoftmaxCrossEntropyWithLogits(sparse=True, reduction='mean')
# Define the model checkpoint
config_ck = CheckpointConfig(save_checkpoint_steps=1000, keep_checkpoint_max=10)
ckpt_cb = ModelCheckpoint(prefix="resnet50", directory="./checkpoints/", config=config_ck)
# Define the training dataset
train_data = train_data.batch(batch_size, drop_remainder=True)
# Define the testing dataset
test_data = test_data.batch(batch_size, drop_remainder=True)
# Define the model and train it
model = Model(net, loss_fn=loss_fn, optimizer=optimizer, metrics={"Accuracy": Accuracy()})
model.train(epoch_size, train_data, callbacks=[ckpt_cb, LossMonitor()], dataset_sink_mode=True)
# Load the trained model and test it
param_dict = load_checkpoint("./checkpoints/resnet50-200_1000.ckpt")
load_param_into_net(net, param_dict)
model = Model(net, loss_fn=loss_fn, metrics={"Accuracy": Accuracy()})
result = model.eval(test_data)
print("Accuracy: ", result["Accuracy"])
```
修复的问题包括:
1. 在定义 optimizer 时,`resnet50` 没有被定义,应该改为 `net`。
2. 在定义 model checkpoint 时,`config` 应该改为 `config_ck`。
3. 在调用 `load_param_into_net` 时,应该传入 `net`,而不是 `loss_fn`。
MES系统界面点击下拉框报错:There was an error in the callback.
当在MES系统界面点击下拉框时出现"There was an error in the callback."的错误提示时,这通常意味着在下拉框的回调函数中发生了错误。回调函数是在用户与下拉框交互时触发的函数,用于处理选择或其他操作。
以下是一些可能导致此错误的常见原因和解决方法:
1. 回调函数错误:请检查回调函数的实现是否正确。确保回调函数的逻辑正确,没有语法错误或逻辑错误。可以通过调试或日志记录来查找并修复问题。
2. 数据源问题:检查下拉框的数据源是否正确配置和加载。确保数据源能够正确提供下拉框所需的选项值。如果数据源有问题,可以尝试重新加载或修复数据源。
3. 权限问题:确认当前登录用户是否具有访问下拉框数据或执行回调函数的权限。如果没有足够的权限,可能会导致回调函数出错。请联系系统管理员以获取适当的权限。
4. 网络或服务器问题:在某些情况下,网络连接不稳定或服务器出现问题可能导致回调函数失败。请确保网络连接正常,并确保服务器运行正常。
如果以上方法都无法解决问题,请考虑联系MES系统的技术支持团队,向他们提供详细的错误信息和操作步骤,以便他们能够提供更具体的帮助和支持。
相关推荐
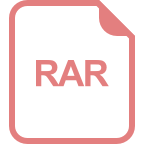
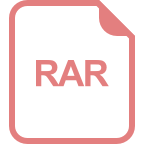
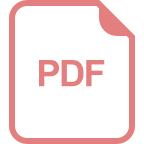












