将tensor进行整理,参数为conf_thres,用python代码怎么写
时间: 2024-03-17 13:42:47 浏览: 20
假设要整理的tensor为`tensor_data`,参数`conf_thres`表示阈值,以下是用Python代码实现的示例:
```python
import torch
def filter_tensor_by_confidence(tensor_data, conf_thres):
# 获取置信度大于阈值的元素的索引
idx = torch.where(tensor_data[:, :, 4] >= conf_thres)
# 根据索引获取符合条件的元素
filtered_tensor = tensor_data[idx]
return filtered_tensor
```
在这个示例中,我们通过`torch.where`函数获取了置信度大于等于阈值的元素的索引,然后通过索引获取了符合条件的元素。这个函数返回一个新的tensor,其中包含符合条件的元素。
相关问题
import torchimport cv2import numpy as npfrom models.experimental import attempt_loadfrom utils.general import non_max_suppressionclass YoloV5Detector: def __init__(self, model_path, conf_thresh=0.25, iou_thresh=0.45): self.conf_thresh = conf_thresh self.iou_thresh = iou_thresh self.device = torch.device("cuda" if torch.cuda.is_available() else "cpu") self.model = attempt_load(model_path, map_location=self.device) self.model.eval() def detect(self, image_path): img = cv2.imread(image_path) img = cv2.cvtColor(img, cv2.COLOR_BGR2RGB) img = img.transpose(2, 0, 1) img = np.ascontiguousarray(img) img = torch.from_numpy(img).to(self.device).float() / 255.0 # Run inference with torch.no_grad(): results = self.model(img, size=img.shape[-2:]) results = non_max_suppression(results, conf_thres=self.conf_thresh, iou_thres=self.iou_thresh) return results
这是一个使用 YOLOv5 模型进行目标检测的 Python 代码。该类 YoloV5Detector 包含了模型加载、图片预处理和推理的功能。具体来说,会使用 OpenCV 库读取图片,将其转换为 RGB 格式,然后转换为 PyTorch 的 Tensor 格式,并将其送入 YOLOv5 模型中进行推理。最后,使用非极大值抑制算法(NMS)筛选出检测出来的物体,并返回结果。其中,conf_thresh 和 iou_thresh 分别表示置信度和重叠阈值,可以通过调整这两个参数来控制检测结果的准确率和召回率。
python代码用yolov5识别物体类别为餐桌要怎么说
使用yolov5识别物体的方法如下:
首先,需要安装yolov5模型和相关的Python库。使用以下命令安装:
```
pip install torch torchvision
pip install git+https://github.com/ultralytics/yolov5.git
```
然后,将图片加载到Python中,使用以下代码:
```
import cv2
# Load image
img = cv2.imread("image.jpg")
```
接着,使用yolov5模型识别物体类别,使用以下代码:
```
import torch
from models.experimental import attempt_load
from utils.general import non_max_suppression, scale_coords
from utils.torch_utils import select_device
# Load model
weights = 'yolov5s.pt'
device = select_device('cpu')
model = attempt_load(weights, map_location=device)
# Define class
class_names = ['table']
# Convert image to tensor
img = torch.from_numpy(img).to(device)
# Inference
results = model(img)
results = non_max_suppression(results, conf_thres=0.5, iou_thres=0.5)
# Process results
for result in results:
if result is not None:
# Rescale coordinates
result[:, :4] = scale_coords(img.shape[2:], result[:, :4], img.shape[:2]).round()
# Print class and confidence
for res in result:
class_idx = int(res[5])
class_name = class_names[class_idx]
confidence = res[4]
print(f"Class: {class_name}, Confidence: {confidence}")
```
在上面的代码中,我们使用`attempt_load`函数加载yolov5模型,然后使用`non_max_suppression`函数进行非极大值抑制以过滤重叠的边界框。接着,我们将坐标缩放回图像大小,并打印检测到的物体的类别和置信度。
如果要识别多个物体类别,可以将`class_names`列表中的`'table'`替换为包含所有类别名称的列表。
相关推荐
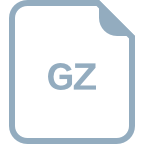












