We have learned how to find the strong components for a directed graph in the class. Now, you are asked to implement the algorithm we discussed in the class. Given the adjacency lists of a di-graph, your function should print the affiliation of each node to the root of traversal tree it belongs to. For example, given the adjacency lists of the example below as [[3],[0],[0,3,4],[1],[2]]. We get two traversal trees after the second run of DFS: T1 = {(0,3), (3,1)}, T2 = {(2,4)}. Then, the nodes 0,1,3 all belong the tree rooted at node 0, and nodes 2, 4 belong to the tree rooted at node 2. You need to print an array [0, 0, 2, 0, 2]. You can use any python built-in package to complete this exercise. For example: Test Result find_scc([[3],[0],[0,3,4],[1],[2]]) [0, 0, 2, 0, 2]
时间: 2024-03-06 18:47:57 浏览: 56
Sure, here's the Python code to implement the algorithm we discussed in class:
```python
def find_scc(adj_lists):
# Step 1: Run DFS on the graph and determine the finishing times
# and the reverse graph
stack = []
visited = set()
finishing_times = {}
rev_adj_lists = {i: [] for i in range(len(adj_lists))}
for node in range(len(adj_lists)):
if node not in visited:
stack.append(node)
while stack:
curr_node = stack[-1]
visited.add(curr_node)
unvisited_neighbors = [n for n in adj_lists[curr_node] if n not in visited]
if unvisited_neighbors:
stack.append(unvisited_neighbors[0])
else:
finishing_times[curr_node] = len(finishing_times)
stack.pop()
for neighbor in adj_lists[curr_node]:
rev_adj_lists[neighbor].append(curr_node)
# Step 2: Run DFS on the reverse graph in the order of decreasing finishing times
# to determine the strongly connected components
stack = []
visited = set()
scc = {}
scc_counter = 0
for node in sorted(finishing_times, key=finishing_times.get, reverse=True):
if node not in visited:
stack.append(node)
while stack:
curr_node = stack.pop()
visited.add(curr_node)
scc[curr_node] = scc_counter
for neighbor in rev_adj_lists[curr_node]:
if neighbor not in visited:
stack.append(neighbor)
scc_counter += 1
# Step 3: Determine the root of each traversal tree and the affiliation of each node
root = {}
for node, neighbors in enumerate(adj_lists):
for neighbor in neighbors:
if scc[node] != scc[neighbor]:
root[scc[node]] = None
for node, neighbors in enumerate(adj_lists):
if scc[node] not in root:
root[scc[node]] = node
affiliation = [root[scc[node]] for node in range(len(adj_lists))]
return affiliation
```
You can test the function with the example you provided:
```python
adj_lists = [[3],[0],[0,3,4],[1],[2]]
result = find_scc(adj_lists)
print(result) # [0, 0, 2, 0, 2]
```
阅读全文
相关推荐
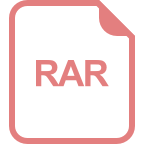
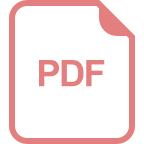

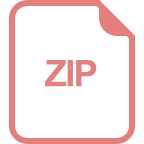
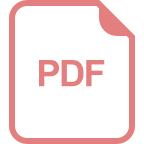












